Robust Intrusion Detection for Industrial Control Systems Using Improved Autoencoder and Bayesian Gaussian Mixture Model
Mathematics(2023)
摘要
Machine learning-based intrusion detection systems are an effective way to cope with the increasing security threats faced by industrial control systems. Considering that it is hard and expensive to obtain attack data, it is more reasonable to develop a model trained with only normal data. However, both high-dimensional data and the presence of outliers in the training set result in efficiency degradation. In this research, we present a hybrid intrusion detection method to overcome these two problems. First, we created an improved autoencoder that incorporates the deep support vector data description (Deep SVDD) loss into the training of the autoencoder. Under the combination of Deep SVDD loss and reconstruction loss, the novel autoencoder learns a more compact latent representation from high-dimensional data. The density-based spatial clustering of applications with noise algorithm is then used to remove potential outliers in the training data. Finally, a Bayesian Gaussian mixture model is used to identify anomalies. It learns the distribution of the filtered training data and uses the probabilities to classify normal and anomalous samples. We conducted a series of experiments on two intrusion detection datasets to assess performance. The proposed model performs better than other baseline methods when dealing with high-dimensional and contaminated data.
更多查看译文
关键词
industrial control systems,network security,intrusion detection,anomaly detection,autoencoder
AI 理解论文
溯源树
样例
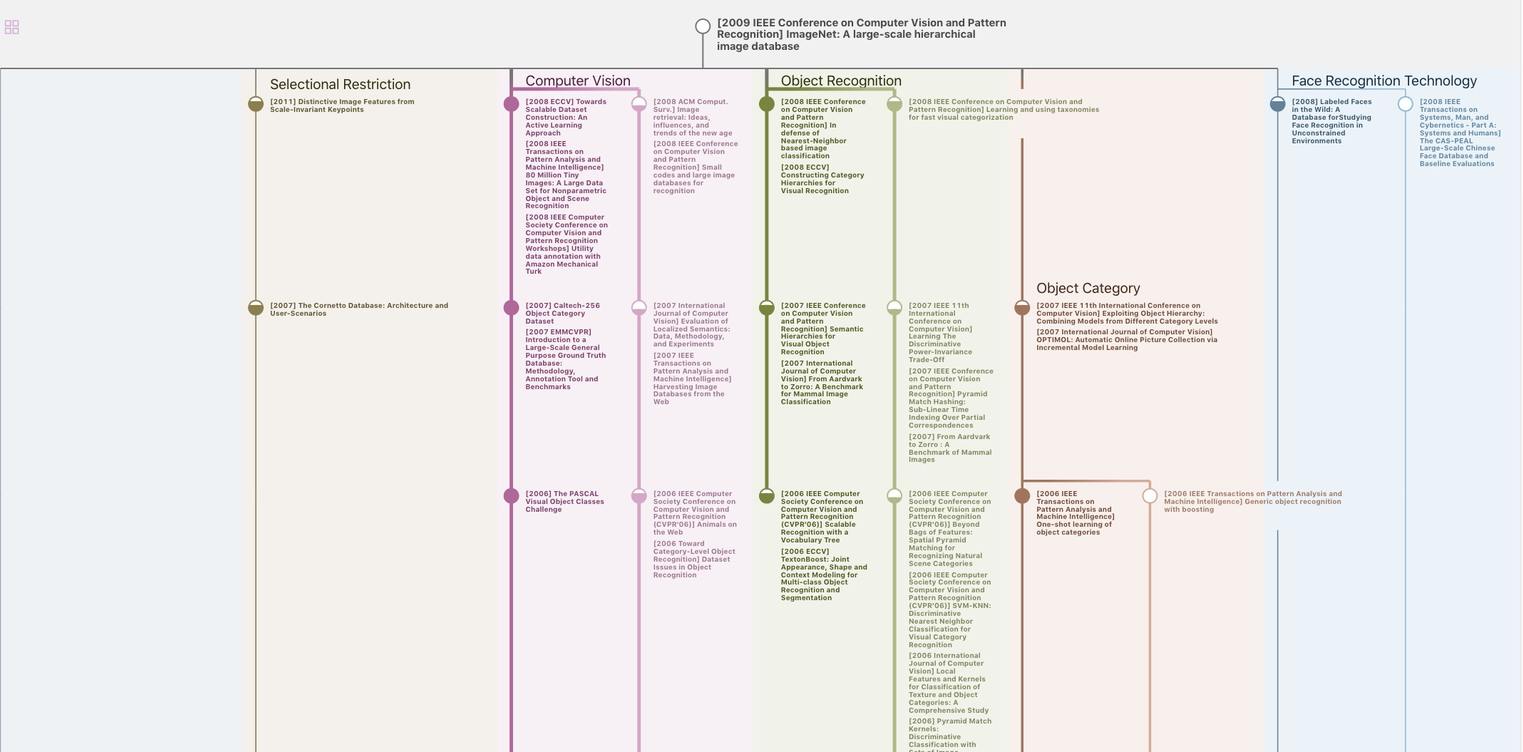
生成溯源树,研究论文发展脉络
Chat Paper
正在生成论文摘要