Comparison of machine-learning and logistic regression models to predict 30-day unplanned readmission: a development and validation study
medRxiv (Cold Spring Harbor Laboratory)(2023)
摘要
We compared the predictive performance of gradient-boosted decision tree (GBDT), random forest (RF), deep neural network (DNN), and logistic regression (LR) with the least absolute shrinkage and selection operator (LASSO) for 30–day unplanned readmission, according to the number of predictor variables and presence/absence of blood–test results. We used electronic health records of patients discharged alive from 38 hospitals in 2015–2017 for derivation (n=339,513) and in 2018 for validation (n=118,074), including basic characteristics (age, sex, admission diagnosis category, number of hospitalizations in the past year, discharge location), diagnosis, surgery, procedure, and drug codes, and blood–test results. We created six patterns of datasets having different numbers of binary variables (that ≥5% or ≥1% of patients or ≥10 patients had) with and without blood–test results. For the dataset with the smallest number of variables (102), the c–statistic was highest for GBDT (0.740), followed by RF (0.734), LR–LASSO (0.720), and DNN (0.664). For the dataset with the largest number of variables (1543), the c–statistic was highest for GBDT (0.764), followed by LR–LASSO (0.755), RF (0.751), and DNN (0.720). We found that GBDT generally outperformed LR–LASSO, but the difference became smaller when the number of variables was increased and blood–test results were used.
### Competing Interest Statement
The authors have declared no competing interest.
### Funding Statement
This study was supported by a Japan Society for the Promotion of Science (JSPS) KAKENHI Grant (No. 19K19430) from the Japanese Ministry of Education, Culture, Sports, Science, and Technology. The funder had no role in study design, data collection, data analysis, data interpretation, or writing.
### Author Declarations
I confirm all relevant ethical guidelines have been followed, and any necessary IRB and/or ethics committee approvals have been obtained.
Yes
The details of the IRB/oversight body that provided approval or exemption for the research described are given below:
The study was approved by the Ethics Committee of the University of Tsukuba (approval no. 1414) in accordance with the Declaration of Helsinki. Because the claims data were anonymized before the researchers received them, individual participants consent was waived according to the ethical guidelines for medical and health research involving human subjects.
I confirm that all necessary patient/participant consent has been obtained and the appropriate institutional forms have been archived, and that any patient/participant/sample identifiers included were not known to anyone (e.g., hospital staff, patients or participants themselves) outside the research group so cannot be used to identify individuals.
Yes
I understand that all clinical trials and any other prospective interventional studies must be registered with an ICMJE-approved registry, such as ClinicalTrials.gov. I confirm that any such study reported in the manuscript has been registered and the trial registration ID is provided (note: if posting a prospective study registered retrospectively, please provide a statement in the trial ID field explaining why the study was not registered in advance).
Yes
I have followed all appropriate research reporting guidelines, such as any relevant EQUATOR Network research reporting checklist(s) and other pertinent material, if applicable.
Yes
We obtained data from Medical Data Vision Co., Ltd. (MDV) and are not allowed to share these data with other parties. Researchers who meet the criteria for access can acquire de-identified participant data from MDV (https://en.mdv.co.jp).
更多查看译文
关键词
unplanned readmission,logistic regression models,validation study,machine-learning machine-learning
AI 理解论文
溯源树
样例
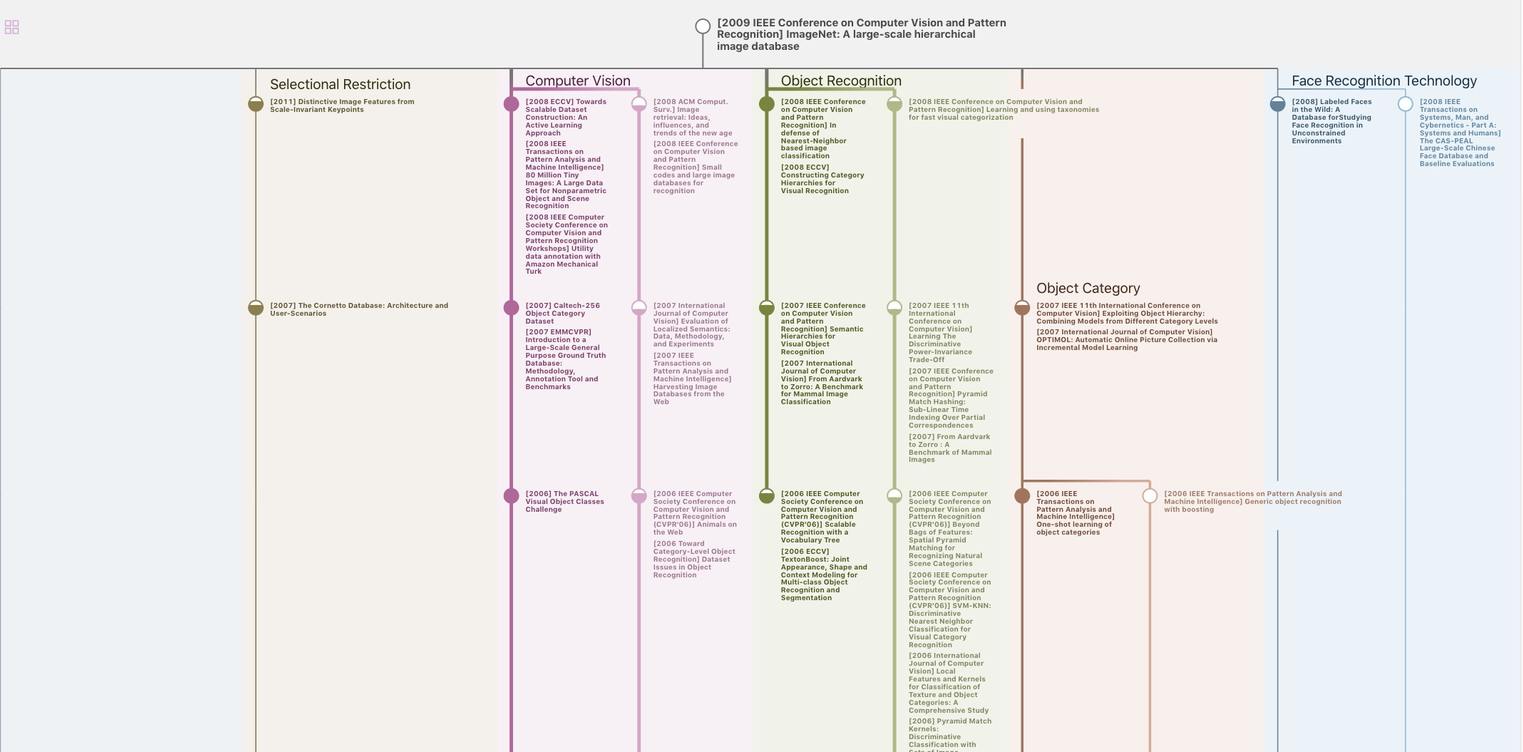
生成溯源树,研究论文发展脉络
Chat Paper
正在生成论文摘要