A Mobile 3-D Object Recognition Processor With Deep-Learning-Based Monocular Depth Estimation
IEEE Micro(2023)
摘要
A 3-D object recognition system is a heavy task that consumes high sensor power and requires complex 3-D data processing. In this article, the proposed processor produces 3-D red, green, blue, and depth (RGB-D) data from an RGB image through a deep learning-based monocular depth estimation, and then its RGB-D data are sporadically calibrated with low-resolution depth data from a low-power depth sensor, lowering the sensor power by 27.3 times. Then, the proposed processor accelerates various convolution operations in the system by integrating the in-out skipping-based bit-slice-level computing processing elements and flexibly allocating workloads considering data properties. Moreover, the point feature (PF) aggregator is designed close to the global memory to support the PF reuse algorithm’s data aggregation. Additionally, the window-based search algorithm and its memory management are presented for efficient point processing in the point processing unit. Consequently, the 210-mW and 34-frames-per-second end-to-end 3-D object recognition processor is successfully demonstrated.
更多查看译文
关键词
monocular depth estimation,deep-learning-based deep-learning-based,recognition
AI 理解论文
溯源树
样例
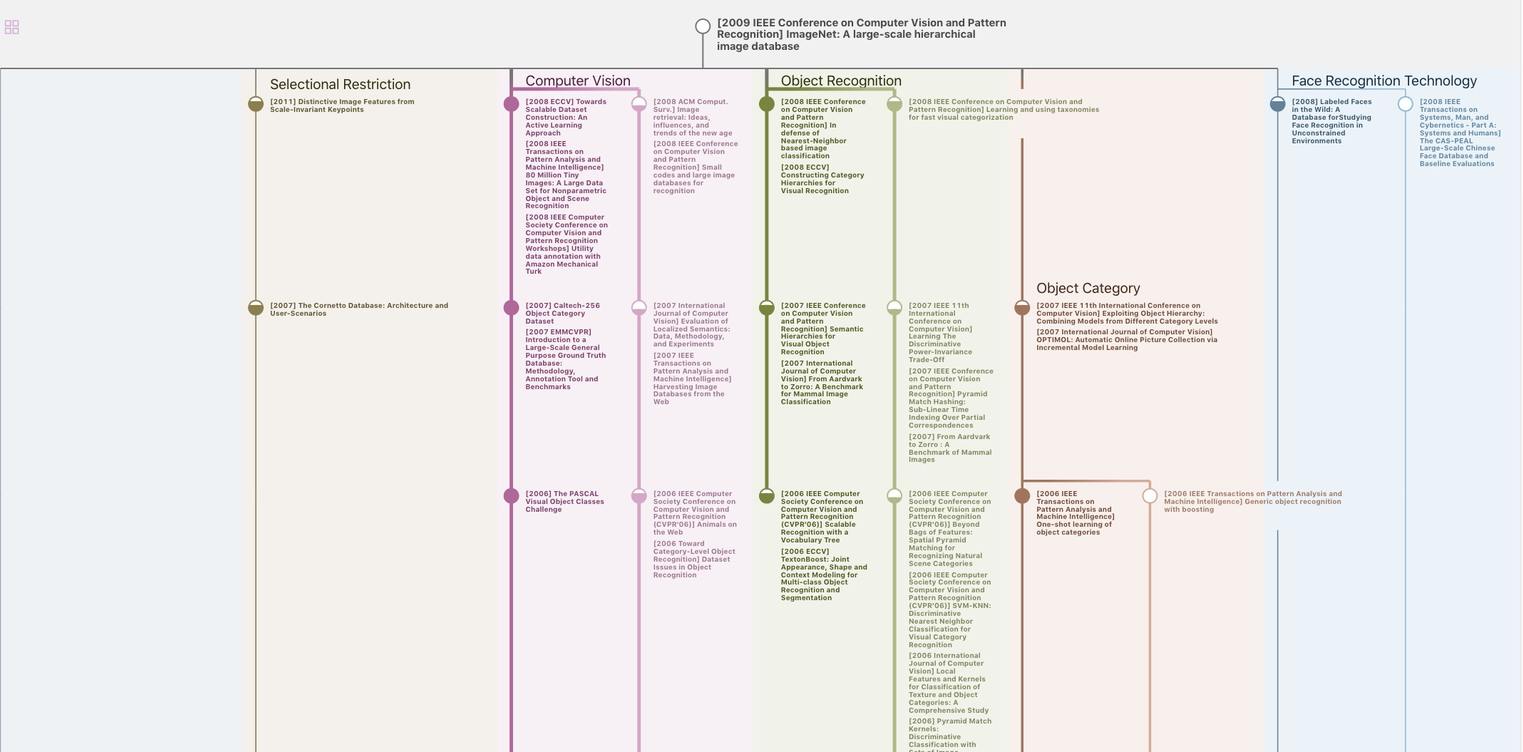
生成溯源树,研究论文发展脉络
Chat Paper
正在生成论文摘要