Evolving Fuzzy Systems in Taxi Demand Forecasting and Classification
Transactions on Fuzzy Sets and Systems(2023)
摘要
This work presents an approach to taxi demand forecasting and classification. The proposed approach uses historical data from taxi rides and meteorological data. The Kruskal-Wallis variable ranking method is used to identify the most relevant variables. The selected variables are used as input to an evolving fuzzy system to perform the prediction. Once the forecast is made, the demand results are classified by value ranges. Those ranges are also identified by colors that compose a heatmap, displayed at each time interval. In this work, to perform the prediction, four evolving systems are evaluated: Autonomous Learning Multi-Model (ALMMo); evolving Multivariable Gaussian Fuzzy Modeling System (eMG); evolving Fuzzy with Multivariable Gaussian Participatory Learning and Recursive Maximum Correntropy eFCE and; evolving Neo-Fuzzy Neuron (eNFN). Computational experiments were carried out to evaluate the evolving systems in predicting Pick-Up and Drop-Off, at intervals of 15 and 30 minutes, for 86 zones in New York, covering the period from 01/01/2018 to 31/ 10/2018. The results obtained by the evolving systems are compared with each other and state of the art. Among the evolving models, ALMMo presented the best results compared to the state of the art and other evolving models. Performance obtained by the evolving models suggests that the proposed approach is promising an alternative to forecasting and classifying passenger demand.
更多查看译文
关键词
forecast,classification,fuzzy systems,evolving systems,taxi demand
AI 理解论文
溯源树
样例
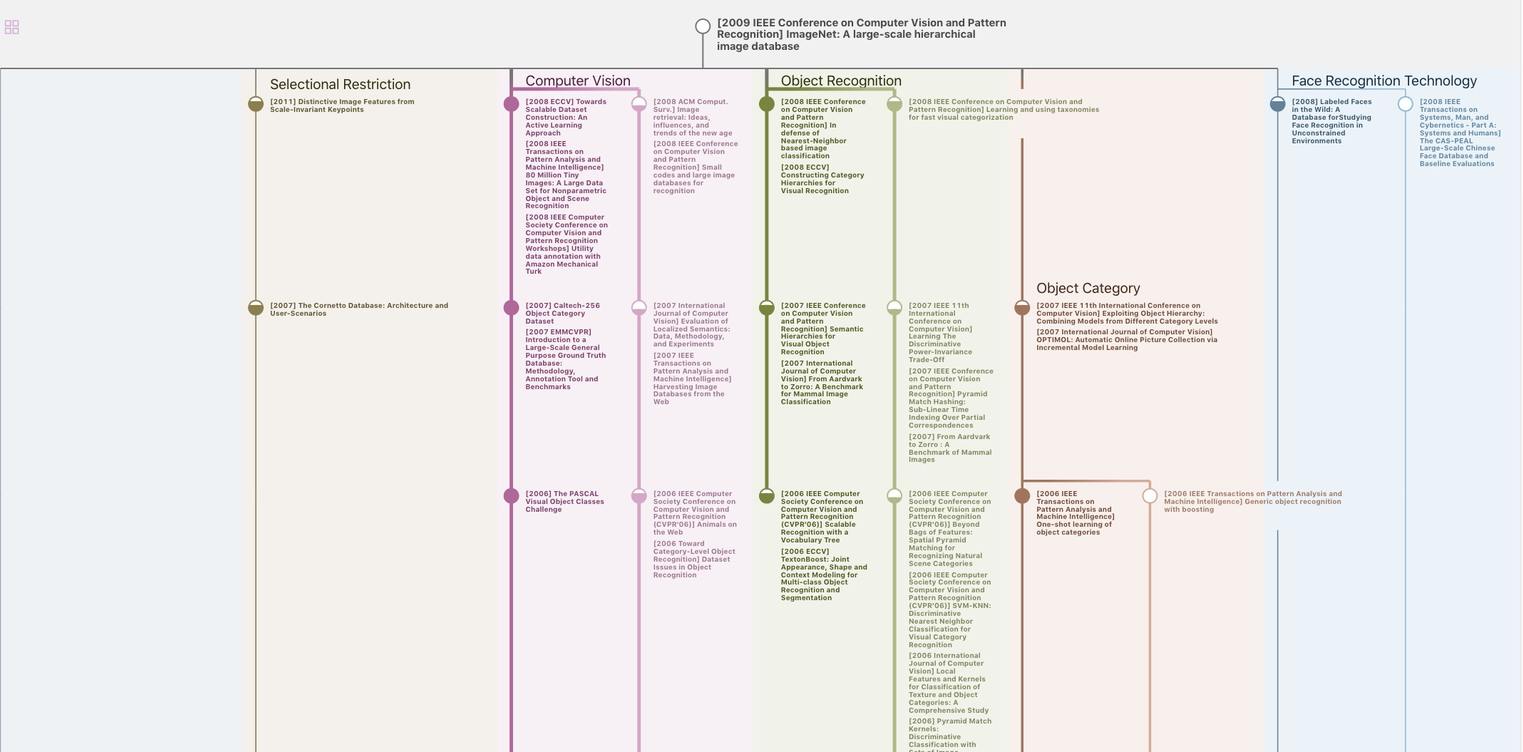
生成溯源树,研究论文发展脉络
Chat Paper
正在生成论文摘要