Locking and Quacking: Stacking Bayesian Model Predictions by Log-Pooling and Superposition
arxiv(2023)
Abstract
Combining predictions from different models is a central problem in Bayesian inference and machine learning more broadly. Currently, these predictive distributions are almost exclusively combined using linear mixtures such as Bayesian model averaging, Bayesian stacking, and mixture of experts. Such linear mixtures impose idiosyncrasies that might be undesirable for some applications, such as multi-modality. While there exist alternative strategies (e.g. geometric bridge or superposition), optimising their parameters usually involves computing an intractable normalising constant repeatedly. We present two novel Bayesian model combination tools. These are generalisations of model stacking, but combine posterior densities by log-linear pooling (locking) and quantum superposition (quacking). To optimise model weights while avoiding the burden of normalising constants, we investigate the Hyvarinen score of the combined posterior predictions. We demonstrate locking with an illustrative example and discuss its practical application with importance sampling.
MoreTranslated text
AI Read Science
Must-Reading Tree
Example
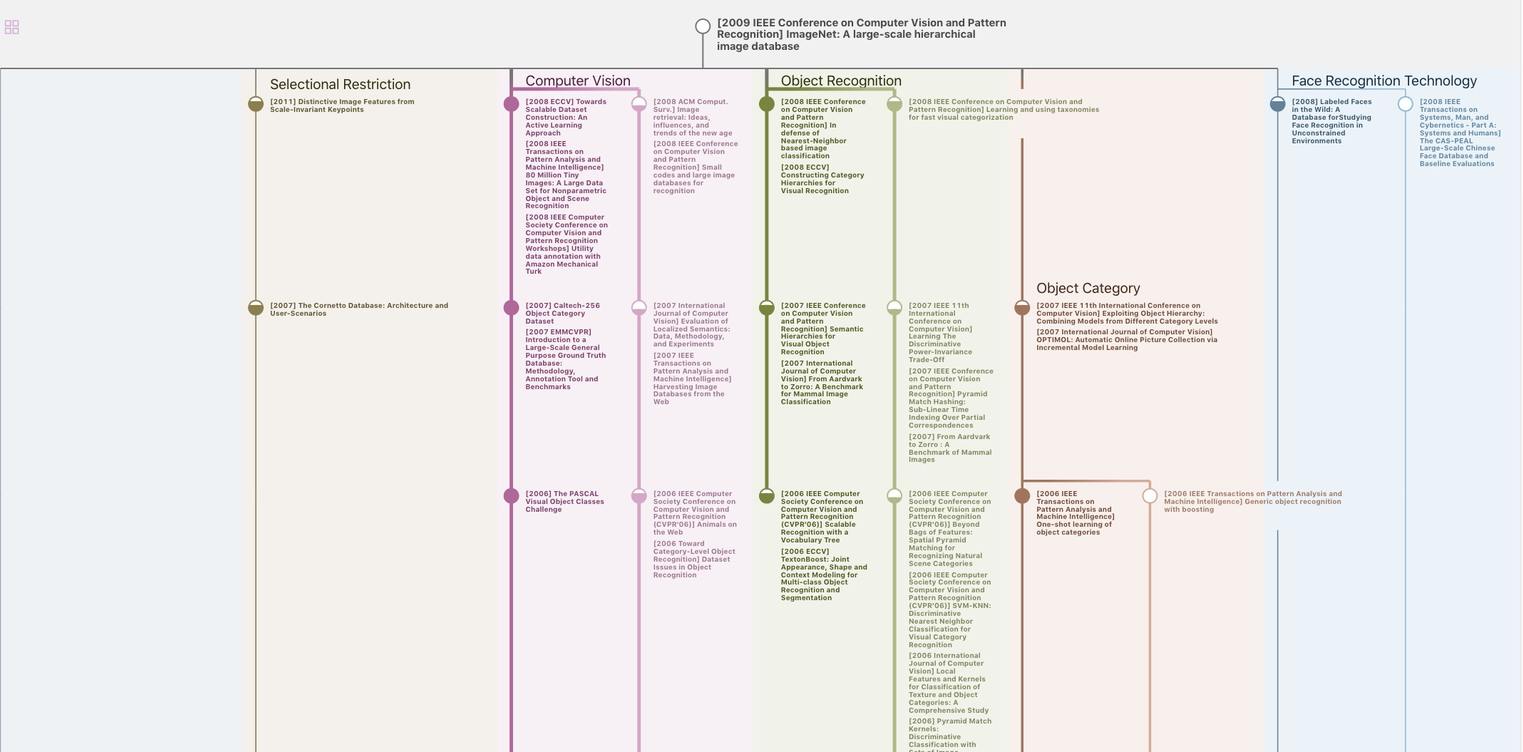
Generate MRT to find the research sequence of this paper
Chat Paper
Summary is being generated by the instructions you defined