基于粒的标记增强标记分布学习
Caai Transactions on Intelligent Systems(2023)
Abstract
标记分布学习能有效求解多标记学习任务,然而分类器构造以获得大规模具有更强监督信息的标注为前提,在许多应用中难以满足.一种替代的方案是以标记增强的方式从传统逻辑形式的标注中挖掘出隐含的数值型标记的重要程度.现有的标记增强方法大多假设增强后的标记需要在所有示例上保持原有逻辑标记的相关性,不能有效保持局部标记相关性.基于粒计算理论,提出了一种适用于标记分布学习的粒化标记增强学习方法.该方法通过k均值聚类构造具有局部相关性语义的信息粒,并在粒的抽象层面上,分别在图上依据逻辑标记的特性和属性空间的拓扑性质完成粒内示例的标记转化.最后,将得到的标记分布在示例层面进行融合,得到描述整个数据集标记重要程度的数值型标记.大量比较研究表明,所提出的模型可以显著地提升多标记学习的性能.
MoreTranslated text
Key words
granular computing,label distribution learning,label enhancement,multi-label,uncertainty,local label correlation,clustering,topology
求助PDF
上传PDF
View via Publisher
AI Read Science
AI Summary
AI Summary is the key point extracted automatically understanding the full text of the paper, including the background, methods, results, conclusions, icons and other key content, so that you can get the outline of the paper at a glance.
Example
Background
Key content
Introduction
Methods
Results
Related work
Fund
Key content
- Pretraining has recently greatly promoted the development of natural language processing (NLP)
- We show that M6 outperforms the baselines in multimodal downstream tasks, and the large M6 with 10 parameters can reach a better performance
- We propose a method called M6 that is able to process information of multiple modalities and perform both single-modal and cross-modal understanding and generation
- The model is scaled to large model with 10 billion parameters with sophisticated deployment, and the 10 -parameter M6-large is the largest pretrained model in Chinese
- Experimental results show that our proposed M6 outperforms the baseline in a number of downstream tasks concerning both single modality and multiple modalities We will continue the pretraining of extremely large models by increasing data to explore the limit of its performance
Upload PDF to Generate Summary
Must-Reading Tree
Example
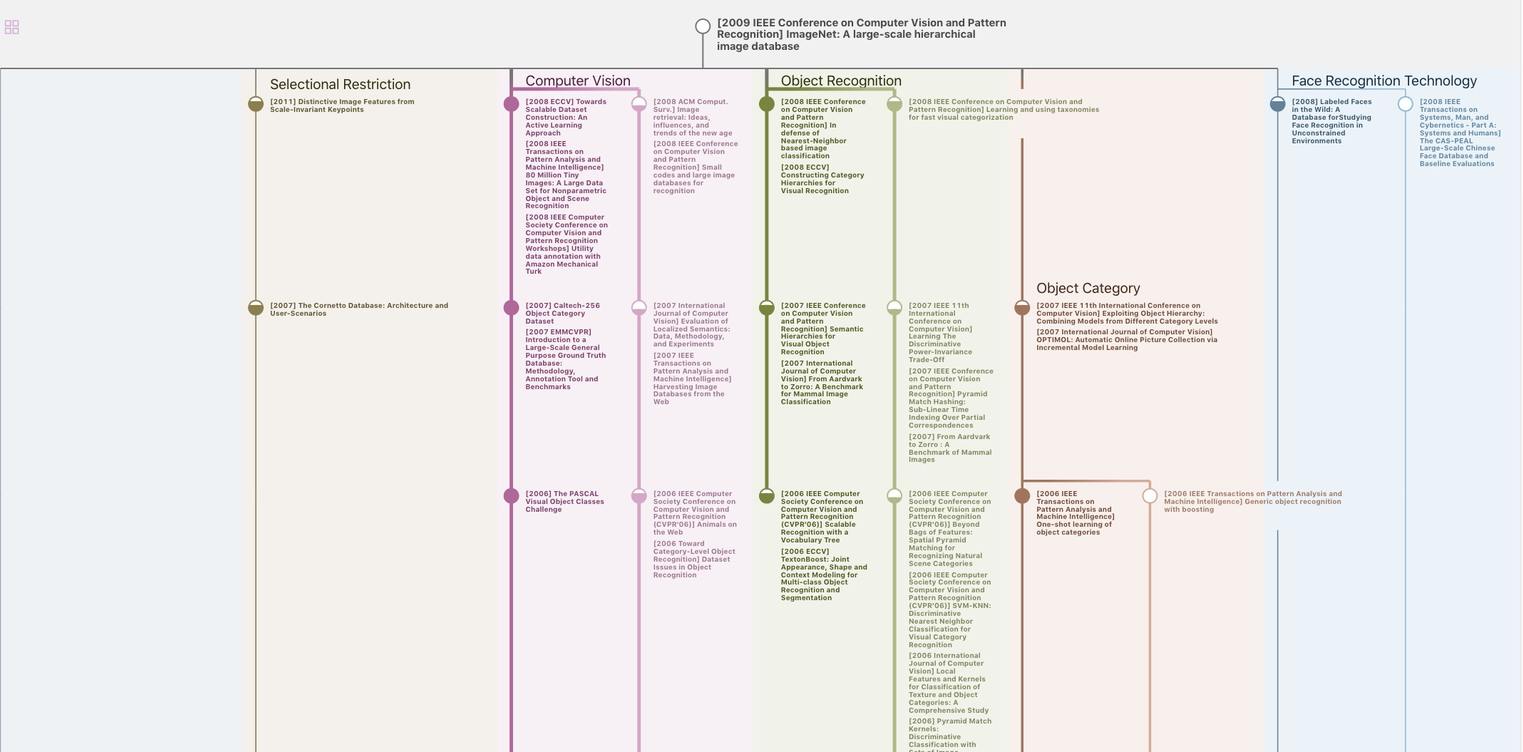
Generate MRT to find the research sequence of this paper
Related Papers
2019
被引用96 | 浏览
2021
被引用1 | 浏览
Data Disclaimer
The page data are from open Internet sources, cooperative publishers and automatic analysis results through AI technology. We do not make any commitments and guarantees for the validity, accuracy, correctness, reliability, completeness and timeliness of the page data. If you have any questions, please contact us by email: report@aminer.cn
Chat Paper
GPU is busy, summary generation fails
Rerequest