Accelerated Algorithms for $$\alpha $$ -Happiness Query
Web and Big Data(2023)
摘要
Extracting a good representative subset of tuples that meets the user’s needs from a large database is an important problem in multi-criteria decision making. Many queries have been proposed for this purpose, including the top-k query and the skyline query. Unfortunately, these traditional queries either ask the user to specify their needs explicitly or overwhelm users with a large output size. Recently, an
$$\alpha $$
-happiness query was proposed, which overcomes the deficiencies of existing queries: users do not need to specify any preference, while they can obtain a small set of tuples such that users are happy with the results, i.e., their favorite tuples in the returned subset is guaranteed to be not much worse than their favorite tuples in the whole database. In this paper, we study the
$$\alpha $$
-happiness query. Inspired by the techniques of incremental convex hull computation, we develop two accelerated algorithms, which maintain useful information to avoid redundant computation, in both 2-dimensional and d-dimensional space (
$$d > 2$$
). We performed extensive experiments, comparing against the best-known method under various settings on both real and synthetic datasets. Our superiority is demonstrated: we can achieve up to two orders and 7 times of improvements in execution times in 2-dimensional and d-dimensional space, respectively.
更多查看译文
关键词
-happiness,Incremental convex hull,Decision making
AI 理解论文
溯源树
样例
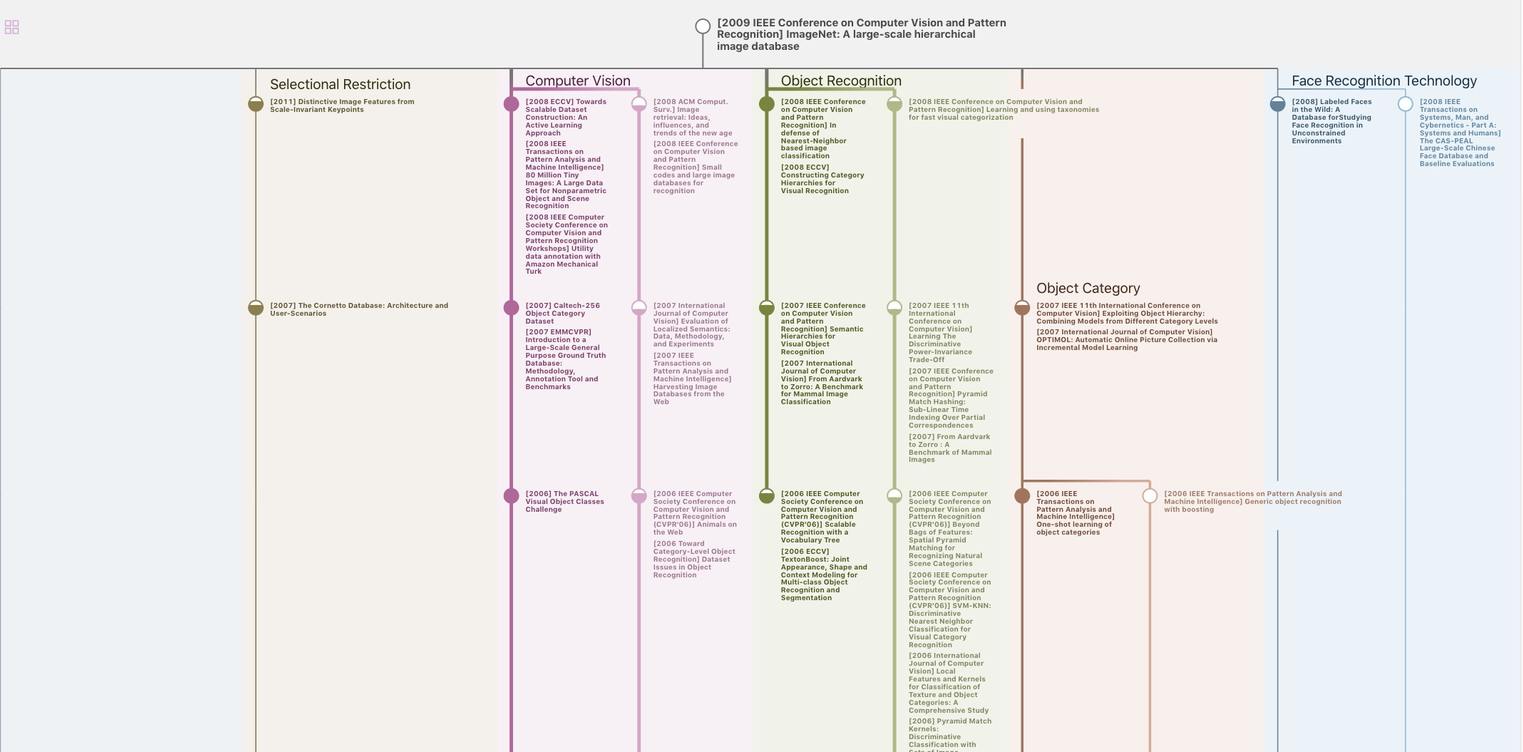
生成溯源树,研究论文发展脉络
Chat Paper
正在生成论文摘要