Who Broke Amazon Mechanical Turk? An Analysis of Crowdsourcing Data Quality over Time
PROCEEDINGS OF THE 15TH ACM WEB SCIENCE CONFERENCE, WEBSCI 2023(2023)
摘要
We present the results of a survey fielded in June of 2022 as a lens to examine recent data reliability issues on Amazon Mechanical Turk. We contrast bad data from this survey with bad data from the same survey fielded among US workers in October 2013, April 2018, and February 2019. Application of an established data cleaning scheme reveals that unusable data has risen from a little over 2% in 2013 to almost 90% in 2022. Through symptomatic diagnosis, we attribute the data reliability drop not to an increase in bad faith work, but rather to a continuum of English proficiency levels. A qualitative analysis of workers' responses to open-ended questions allows us to distinguish between low fluency workers, ultra-low fluency workers, satisficers, and bad faith workers. We go on to show the effects of the new low fluency work on Likert scale data and on the study's qualitative results. Attention checks are shown to be much less effective than they once were at identifying survey responses that should be discarded.
更多查看译文
关键词
Crowdsourcing,data quality,data cleaning,Mechanical Turk
AI 理解论文
溯源树
样例
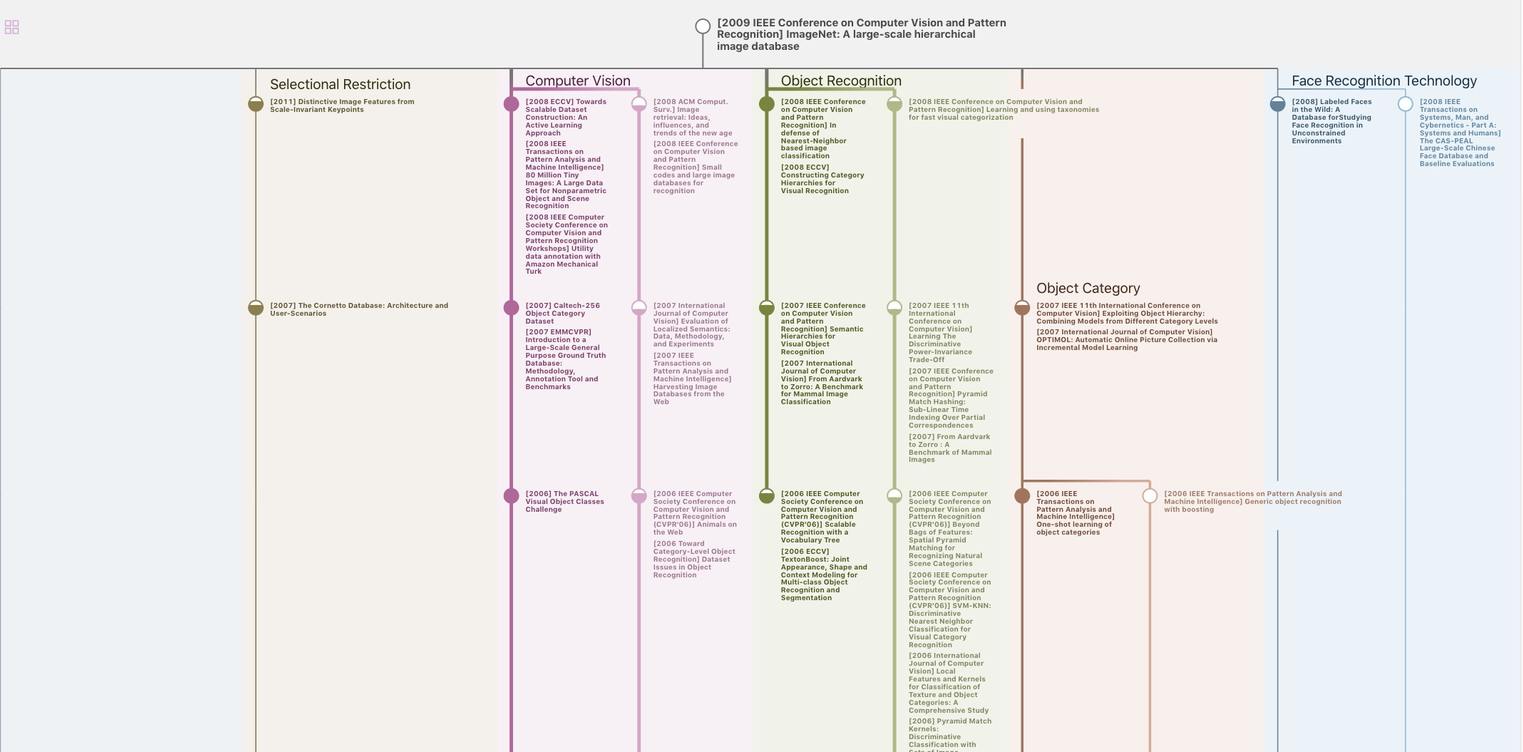
生成溯源树,研究论文发展脉络
Chat Paper
正在生成论文摘要