面向点云补全的鲁棒图关注网络
Journal of China Jiliang University(2023)
AI Read Science
Must-Reading Tree
Example
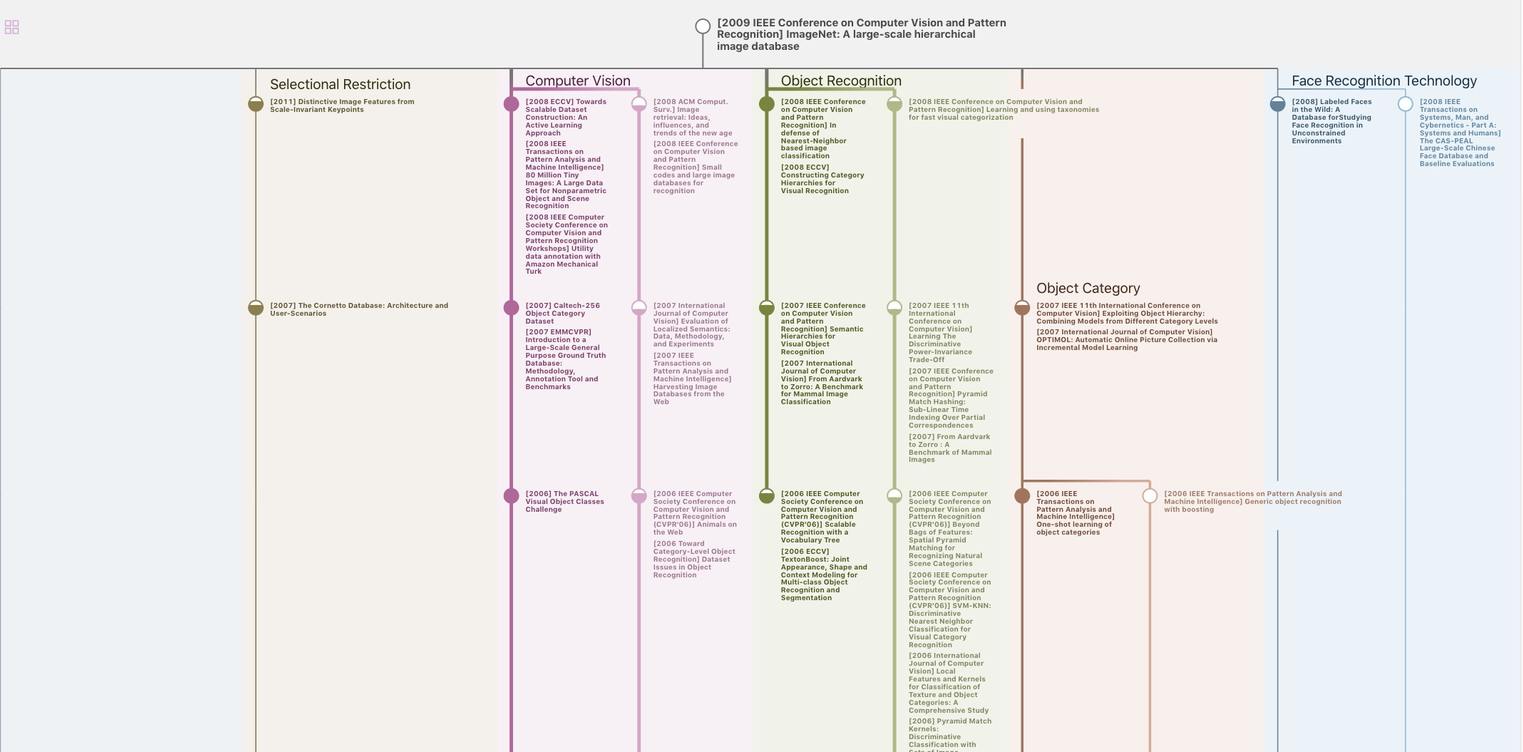
Generate MRT to find the research sequence of this paper
Chat Paper
Summary is being generated by the instructions you defined
Journal of China Jiliang University(2023)