A Hybrid Feature-Extracted Deep CNN with Reduced Parameters Substitutes an End-to-End CNN for the Recognition of Spoken Bengali Digits.
Multimedia tools and applications(2023)
摘要
Speech Recognition (SR) is an emerging field in the native language nowadays. Recognizing isolated words in the local language helps people use smartphones and electronic gadgets without technical or educational knowledge. This paper proposes a novel deep Convolutional Neural Network (CNN) architecture to classify ten spoken Bengali numerals. The proposed model generates almost similar prediction accuracy as compared to an end-to-end CNN with nine times fewer parameters has been trained. Here, the raw audio samples are pre-processed, and then a unique hybrid feature of Mel Frequency Cepstral Coefficients (MFCC), Spectral Sub-band Energy (SSE), and Log Spectral Sub-band Energy (LSSE) have been extracted frame-wise and engendered into a vector. Finally, these vectors are fed to the proposed architecture of a one-dimensional CNN and achieve the highest test accuracy of 98.52%. The model has been trained for our created speech corpus of 14000 spoken Bengali digits and 30000 spoken English digits from the audio-MNIST dataset. The proposed neural model generates high prediction accuracy with a few times fewer parameters to be trained, generating low computational costs. The outcome of the proposed model is compared with several pre-trained deep learning models; the result shows the model's superiority. Source Code: https://github.com/BachchuPaul/Bengali-Isolated-Spoken-Digit .
更多查看译文
关键词
Hybrid feature,Mel frequency cepstral coefficients,Sub-band energy,Isolated word,One-dimensional convolution neural network,Hyper-parameter tuning,Max pooling
AI 理解论文
溯源树
样例
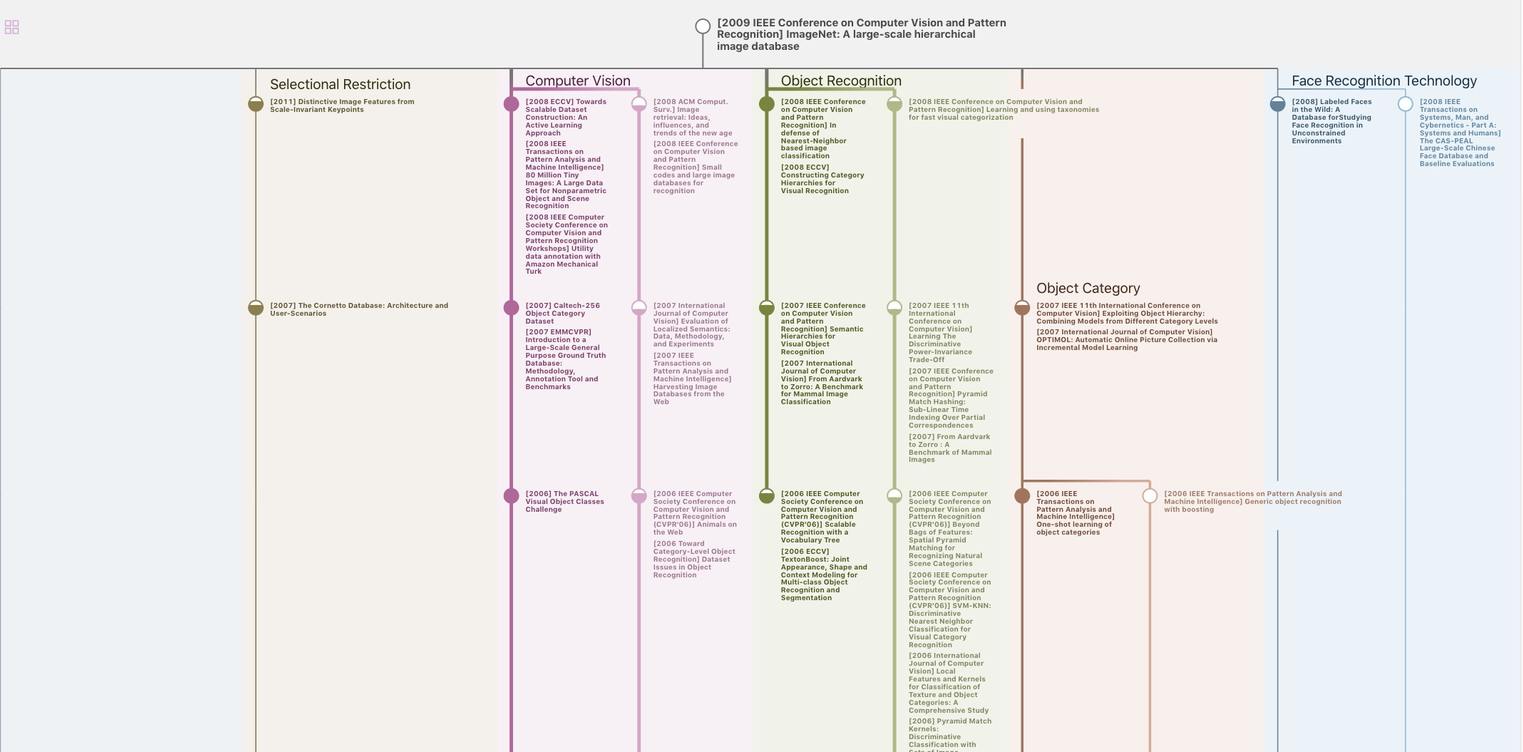
生成溯源树,研究论文发展脉络
Chat Paper
正在生成论文摘要