A GAN-based method for 3D lung tumor reconstruction boosted by a knowledge transfer approach
Multimedia Tools and Applications(2023)
摘要
Three-dimensional (3D) image reconstruction of tumors has been one of the most effective techniques for accurately visualizing tumor structures and treatment with high resolution, which requires a set of two-dimensional medical images such as CT slices. In this paper we propose a novel method based on generative adversarial networks (GANs) for 3D lung tumor reconstruction by CT images. The proposed method consists of three stages: lung segmentation, tumor segmentation and 3D lung tumor reconstruction. Lung and tumor segmentation are performed using snake optimization and Gustafson-Kessel (GK) clustering. In the 3D reconstruction part first, features are extracted using the pre-trained VGG model from the tumors that detected in 2D CT slices. Then, a sequence of extracted features is fed into an LSTM to output compressed features. Finally, the compressed feature is used as input for GAN, where the generator is responsible for high-level reconstructing the 3D image of the lung tumor. The main novelty of this paper is the use of GAN to reconstruct a 3D lung tumor model for the first time, to the best of our knowledge. Also, we used knowledge transfer to extract features from 2D images to speed up the training process. The results obtained from the proposed model on the LUNA dataset showed better results than state of the art. According to HD and ED metrics, the proposed method has the lowest values of 3.02 and 1.06, respectively, as compared to those of other methods. The experimental results show that the proposed method performs better than previous similar methods and it is useful to help practitioners in the treatment process.
更多查看译文
关键词
Lung cancer,3D tumor reconstruction,LSTM,Generative adversarial network,VGGNet
AI 理解论文
溯源树
样例
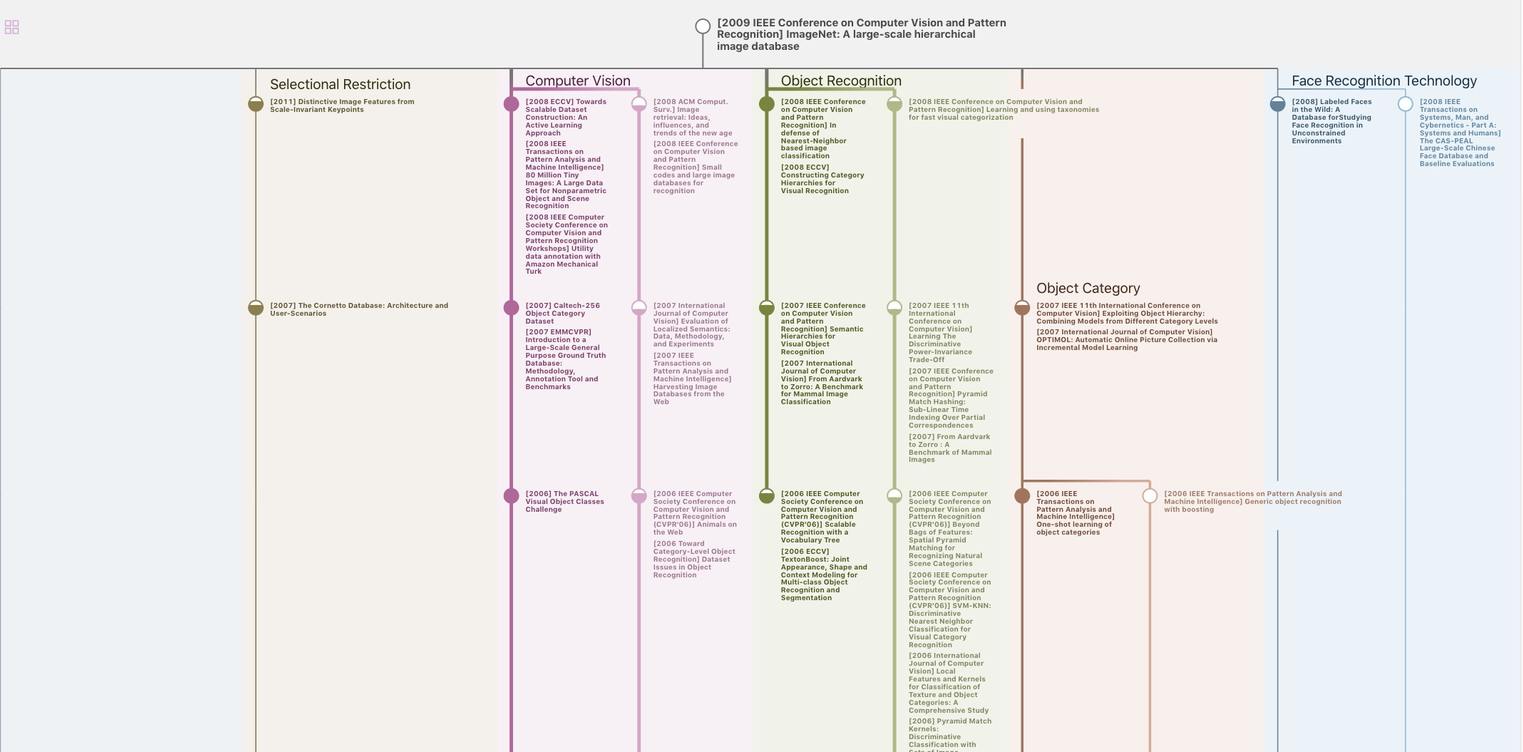
生成溯源树,研究论文发展脉络
Chat Paper
正在生成论文摘要