Long-Term Value of Exploration: Measurements, Findings and Algorithms
PROCEEDINGS OF THE 17TH ACM INTERNATIONAL CONFERENCE ON WEB SEARCH AND DATA MINING, WSDM 2024(2024)
摘要
Effective exploration is believed to positively influence the longterm user experience on recommendation platforms. Determining its exact benefits, however, has been challenging. Regular A/B tests on exploration often measure neutral or even negative engagement metrics while failing to capture its long-term benefits. We here introduce new experiment designs to formally quantify the long-term value of exploration by examining its effects on content corpus, and connecting content corpus growth to the long-term user experience from real-world experiments. Once established the values of exploration, we investigate the Neural Linear Bandit algorithm as a general framework to introduce exploration into any deep learning based ranking systems. We conduct live experiments on one of the largest short-form video recommendation platforms that serves billions of users to validate the new experiment designs, quantify the long-term values of exploration, and to verify the effectiveness of the adopted neural linear bandit algorithm for exploration.
更多查看译文
关键词
Recommendation systems,Experiment Design,Exploration
AI 理解论文
溯源树
样例
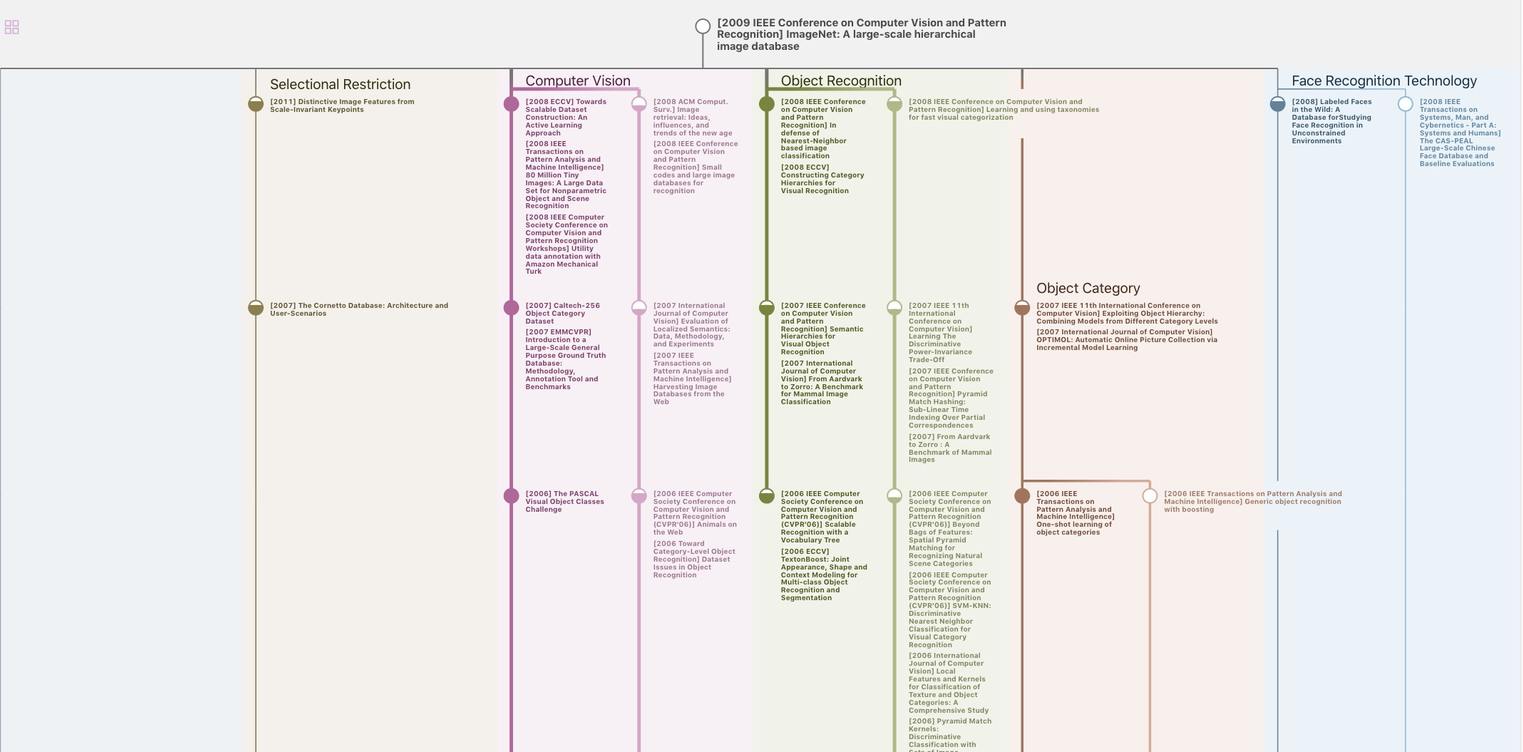
生成溯源树,研究论文发展脉络
Chat Paper
正在生成论文摘要