Contrastive Domain Generalization via Logit Attribution Matching
CoRR(2023)
摘要
Domain Generalization (DG) is an important open problem in machine learning. Deep models are susceptible to domain shifts of even minute degrees, which severely compromises their reliability in real applications. To alleviate the issue, most existing methods enforce various invariant constraints across multiple training domains. However,such an approach provides little performance guarantee for novel test domains in general. In this paper, we investigate a different approach named Contrastive Domain Generalization (CDG), which exploits semantic invariance exhibited by strongly contrastive data pairs in lieu of multiple domains. We present a causal DG theory that shows the potential capability of CDG; together with a regularization technique, Logit Attribution Matching (LAM), for realizing CDG. We empirically show that LAM outperforms state-of-the-art DG methods with only a small portion of paired data and that LAM helps models better focus on semantic features which are crucial to DG.
更多查看译文
关键词
contrastive domain generalization,logit attribution matching
AI 理解论文
溯源树
样例
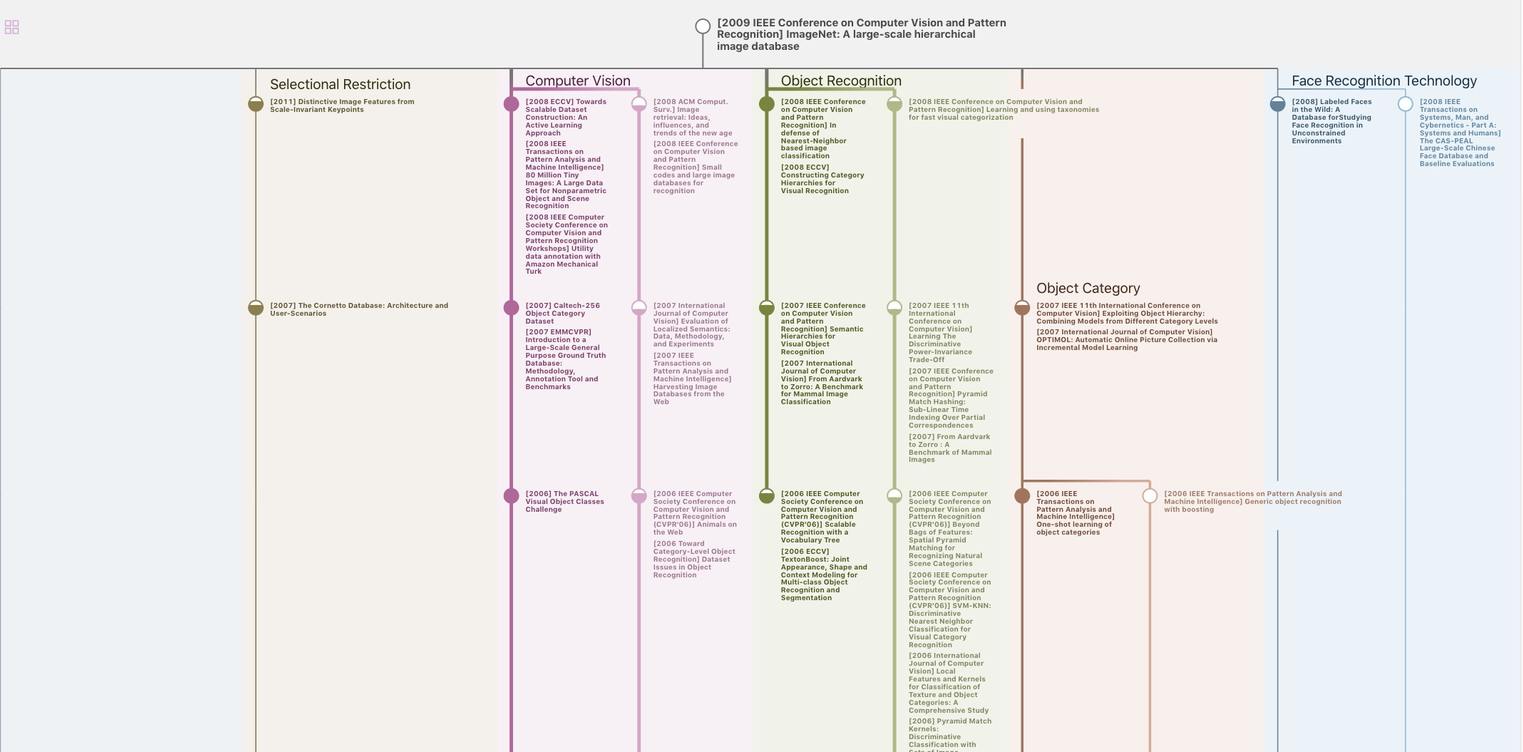
生成溯源树,研究论文发展脉络
Chat Paper
正在生成论文摘要