On the Computational Cost of Stochastic Security
CoRR(2023)
摘要
We investigate whether long-run persistent chain Monte Carlo simulation of Langevin dynamics improves the quality of the representations achieved by energy-based models (EBM). We consider a scheme wherein Monte Carlo simulation of a diffusion process using a trained EBM is used to improve the adversarial robustness and the calibration score of an independent classifier network. Our results show that increasing the computational budget of Gibbs sampling in persistent contrastive divergence improves the calibration and adversarial robustness of the model, elucidating the practical merit of realizing new quantum and classical hardware and software for efficient Gibbs sampling from continuous energy potentials.
更多查看译文
关键词
security,computational cost
AI 理解论文
溯源树
样例
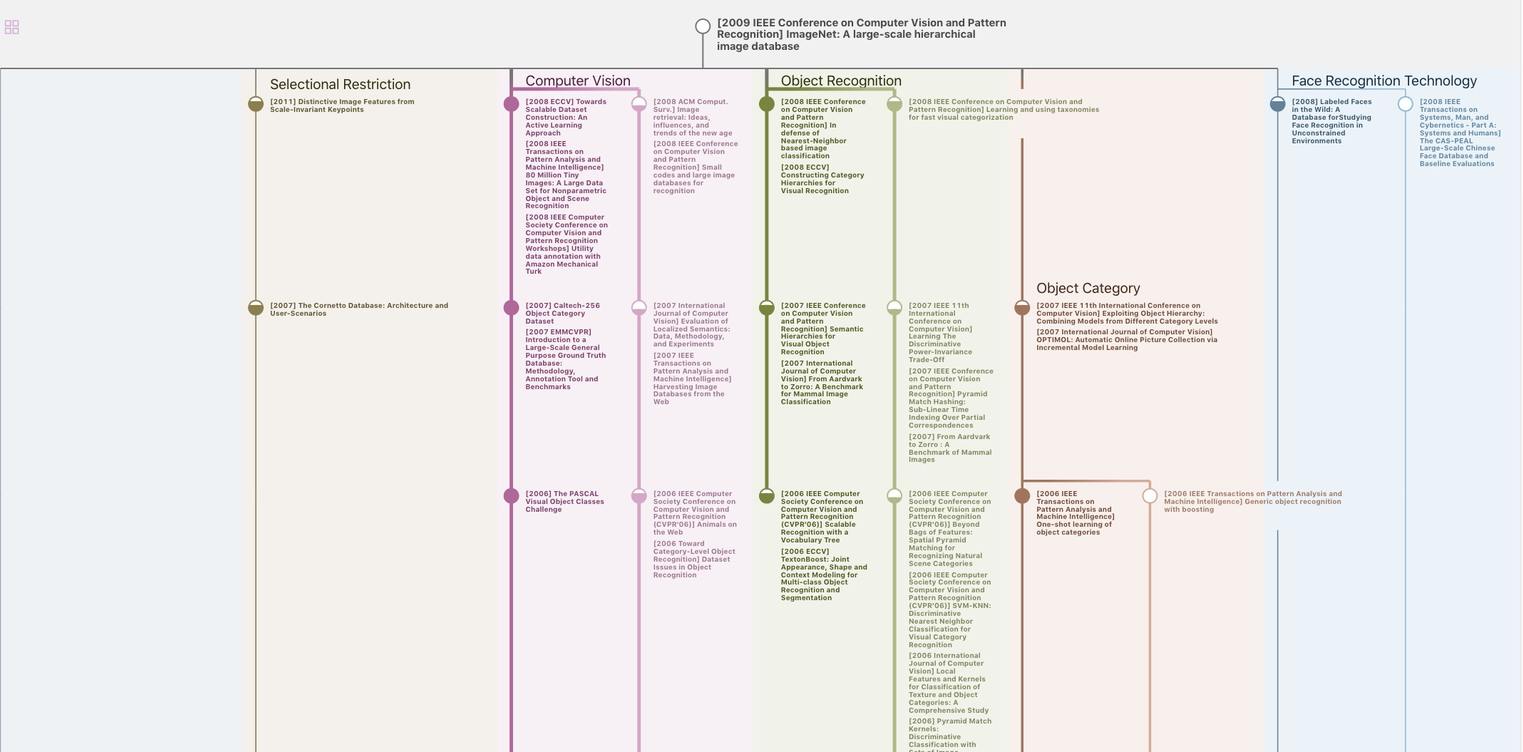
生成溯源树,研究论文发展脉络
Chat Paper
正在生成论文摘要