Bayesian Inference and Global Sensitivity Analysis for Ambient Solar Wind Prediction
SPACE WEATHER-THE INTERNATIONAL JOURNAL OF RESEARCH AND APPLICATIONS(2023)
Abstract
The ambient solar wind plays a significant role in propagating interplanetary coronal mass ejections and is an important driver of space weather geomagnetic storms. A computationally efficient and widely used method to predict the ambient solar wind radial velocity near Earth involves coupling three models: Potential Field Source Surface, Wang-Sheeley-Arge (WSA), and Heliospheric Upwind eXtrapolation. However, the model chain has 11 uncertain parameters that are mainly non-physical due to empirical relations and simplified physics assumptions. We, therefore, propose a comprehensive uncertainty quantification (UQ) framework that is able to successfully quantify and reduce parametric uncertainties in the model chain. The UQ framework utilizes variance-based global sensitivity analysis followed by Bayesian inference via Markov chain Monte Carlo to learn the posterior densities of the most influential parameters. The sensitivity analysis results indicate that the five most influential parameters are all WSA parameters. Additionally, we show that the posterior densities of such influential parameters vary greatly from one Carrington rotation to the next. The influential parameters are trying to overcompensate for the missing physics in the model chain, highlighting the need to enhance the robustness of the model chain to the choice of WSA parameters. The ensemble predictions generated from the learned posterior densities significantly reduce the uncertainty in solar wind velocity predictions near Earth.
MoreTranslated text
Key words
ambient solar wind,uncertainty quantification,sensitivity analysis,Bayesian inference,Monte Carlo
AI Read Science
Must-Reading Tree
Example
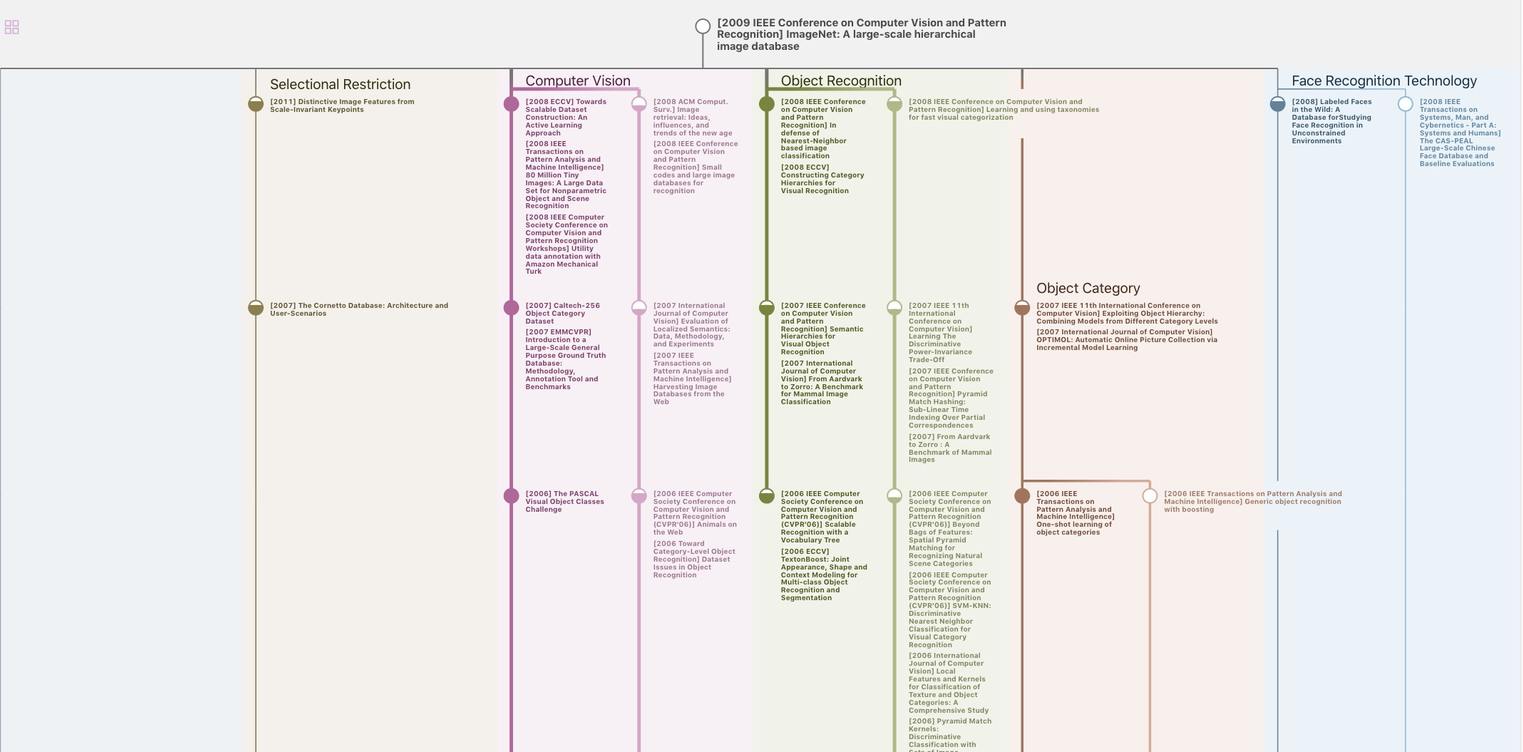
Generate MRT to find the research sequence of this paper
Chat Paper
Summary is being generated by the instructions you defined