FedAds: A Benchmark for Privacy-Preserving CVR Estimation with Vertical Federated Learning
PROCEEDINGS OF THE 46TH INTERNATIONAL ACM SIGIR CONFERENCE ON RESEARCH AND DEVELOPMENT IN INFORMATION RETRIEVAL, SIGIR 2023(2023)
摘要
Conversion rate (CVR) estimation aims to predict the probability of conversion event after a user has clicked an ad. Typically, online publisher has user browsing interests and click feedbacks, while demand-side advertising platform collects users' post-click behaviors such as dwell time and conversion decisions. To estimate CVR accurately and protect data privacy better, vertical federated learning (vFL) is a natural solution to combine two sides' advantages for training models, without exchanging raw data. Both CVR estimation and applied vFL algorithms have attracted increasing research attentions. However, standardized and systematical evaluations are missing: due to the lack of standardized datasets, existing studies adopt public datasets to simulate a vFL setting via hand-crafted feature partition, which brings challenges to fair comparison. We introduce FedAds, the first benchmark for CVR estimation with vFL, to facilitate standardized and systematical evaluations for vFL algorithms. It contains a large-scale real world dataset collected from Alibaba's advertising platform, as well as systematical evaluations for both effectiveness and privacy aspects of various vFL algorithms. Besides, we also explore to incorporate unaligned data in vFL to improve effectiveness, and develop perturbation operations to protect privacy well. We hope that future research work in vFL and CVR estimation benefits from the FedAds benchmark.
更多查看译文
关键词
Ad Ranking,Vertical Federated Learning,Deep Generative Model
AI 理解论文
溯源树
样例
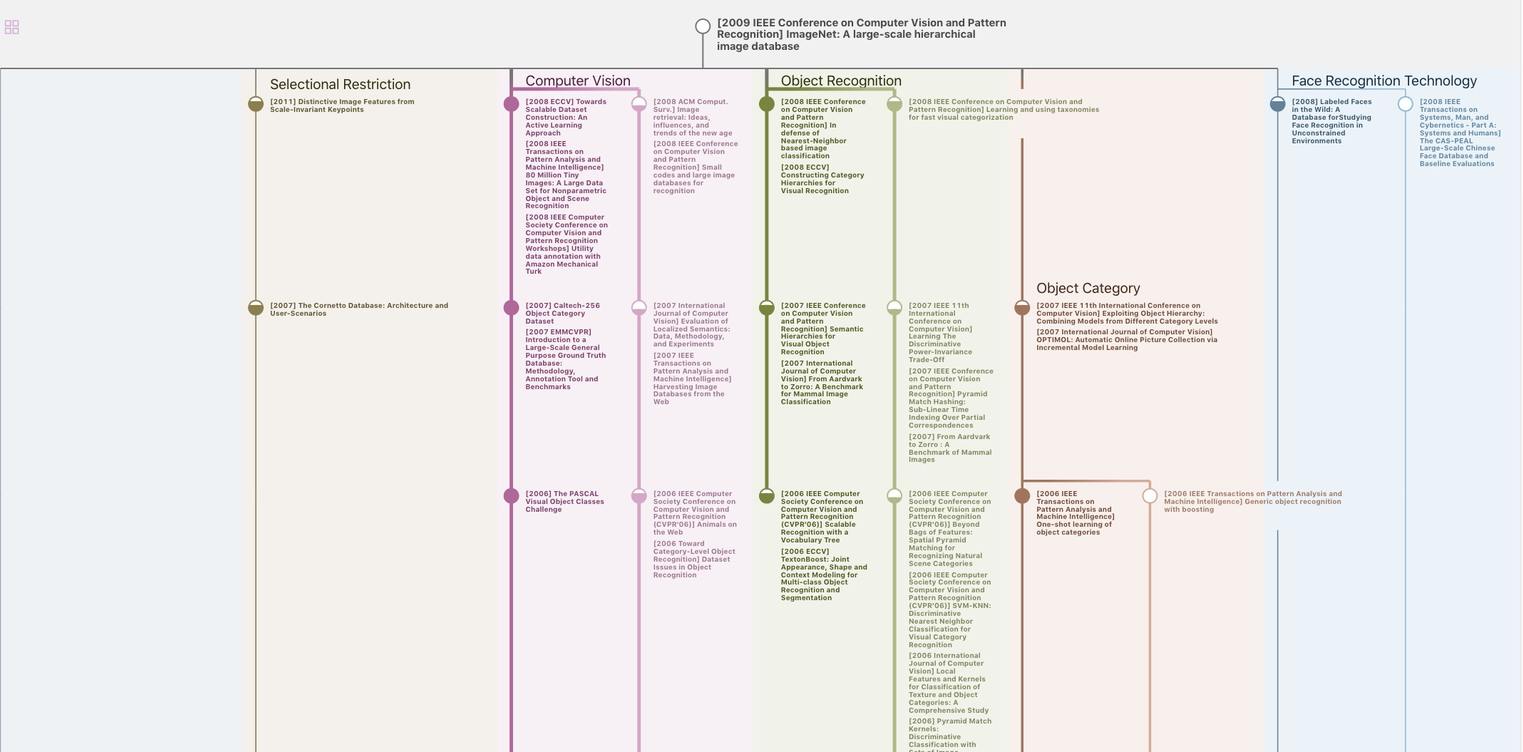
生成溯源树,研究论文发展脉络
Chat Paper
正在生成论文摘要