Kernel-based Joint Independence Tests for Multivariate Stationary and Non-stationary Time Series
Royal Society open science(2023)
摘要
Multivariate time-series data that capture the temporal evolution of interconnected systems are ubiquitous in diverse areas. Understanding the complex relationships and potential dependencies among co-observed variables is crucial for the accurate statistical modelling and analysis of such systems. Here, we introduce kernel-based statistical tests of joint independence in multivariate time series by extending the d-variable Hilbert-Schmidt independence criterion to encompass both stationary and non-stationary processes, thus allowing broader real-world applications. By leveraging resampling techniques tailored for both single- and multiple-realization time series, we show how the method robustly uncovers significant higher-order dependencies in synthetic examples, including frequency mixing data and logic gates, as well as real-world climate, neuroscience and socio-economic data. Our method adds to the mathematical toolbox for the analysis of multivariate time series and can aid in uncovering high-order interactions in data.
更多查看译文
关键词
multivariate time-series analysis,joint independence test,higher-order interaction,kernel method
AI 理解论文
溯源树
样例
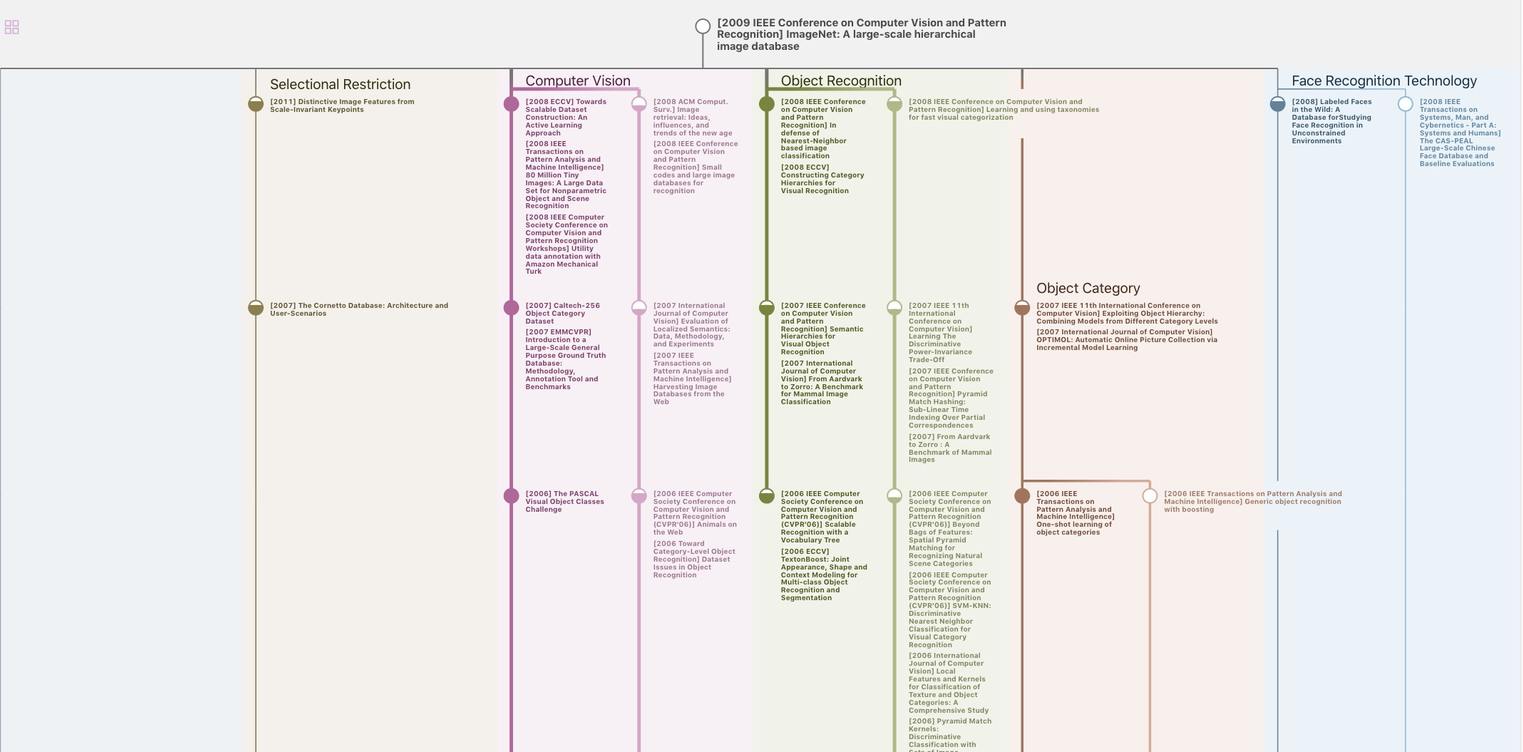
生成溯源树,研究论文发展脉络
Chat Paper
正在生成论文摘要