NLG Evaluation Metrics Beyond Correlation Analysis: An Empirical Metric Preference Checklist
conf_acl(2023)
摘要
In this study, we analyze NLG automatic metrics based on whether human evaluation aspect is used as context or objective to compute the metrics: (i) Task-agnostic and (ii) Human-aligned. Task-agnostic metrics, such as Perplexity, BLEU, BERTScore, are cost-effective and highly adaptable to diverse NLG tasks, yet they have a weak correlation with human. Human-aligned metrics (CTC, CtrlEval, UniEval) improves correlation level by incorporating desirable human-like qualities as training objective. However, their effectiveness at discerning system-level performance and quality of system outputs remains unclear. We present metric preference checklist as a framework to assess the discriminative power of automatic metrics in three NLG tasks: Text Summarization, Dialogue Response Generation, and Controlled Generation. We show that multi-aspect human-aligned metric (UniEval) is not necessarily dominant over single-aspect human-aligned metrics (CTC, CtrlEval) and task-agnostic metrics (BLEU, BERTScore), particularly when a disagreement between human evaluation aspects is present. We also show particular use cases in which automatic metrics provide a better guidance than human on discriminating system-level performance. Our proposed framework provides access: (i) for verifying whether automatic metrics are faithful to human preference, regardless their correlation level to human; and (ii) for scrutinizing the strengths and limitations of NLG systems, which are often obscured by a standard averaging method of evaluation scores.
更多查看译文
关键词
nlg evaluation metrics,empirical metrics,correlation analysis
AI 理解论文
溯源树
样例
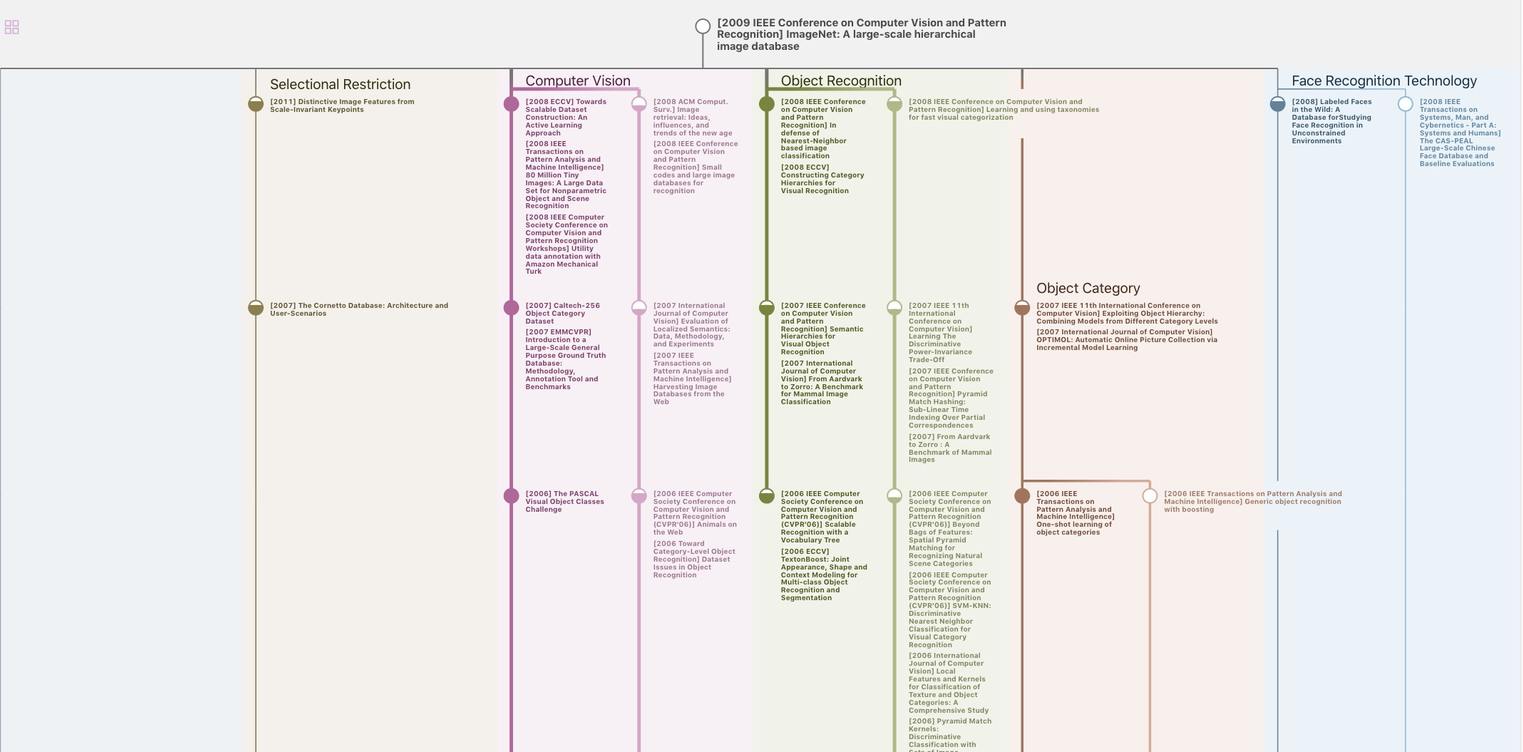
生成溯源树,研究论文发展脉络
Chat Paper
正在生成论文摘要