CoEdge: A Cooperative Edge System for Distributed Real-Time Deep Learning Tasks
PROCEEDINGS OF THE 2023 THE 22ND INTERNATIONAL CONFERENCE ON INFORMATION PROCESSING IN SENSOR NETWORKS, IPSN 2023(2023)
摘要
Recent years have witnessed the emergence of a new class of cooperative edge systems in which a large number of edge nodes can collaborate through local peer-to-peer connectivity. In this paper, we propose CoEdge, a novel cooperative edge system that can support concurrent data/compute-intensive deep learning (DL) models for distributed real-time applications such as city-scale traffic monitoring and autonomous driving. First, CoEdge includes a hierarchical DL task scheduling framework that dispatches DL tasks to edge nodes based on their computational profiles, communication overhead, and real-time requirements. Second, CoEdge can dramatically increase the execution efficiency of DL models by batching sensor data and aggregating the inferences of the same model. Finally, we propose a new edge containerization approach that enables an edge node to execute concurrent DL tasks by partitioning the CPU and GPU workloads into different containers. We extensively evaluate CoEdge on a self-deployed smart lamppost testbed on a university campus. Our results show that CoEdge can achieve up to 82.32% reduction on deadline missing rate compared to baselines.
更多查看译文
关键词
Smart City,Edge Computing,Distributed Deep Learning System,Real-time Scheduling,Edge Containerization
AI 理解论文
溯源树
样例
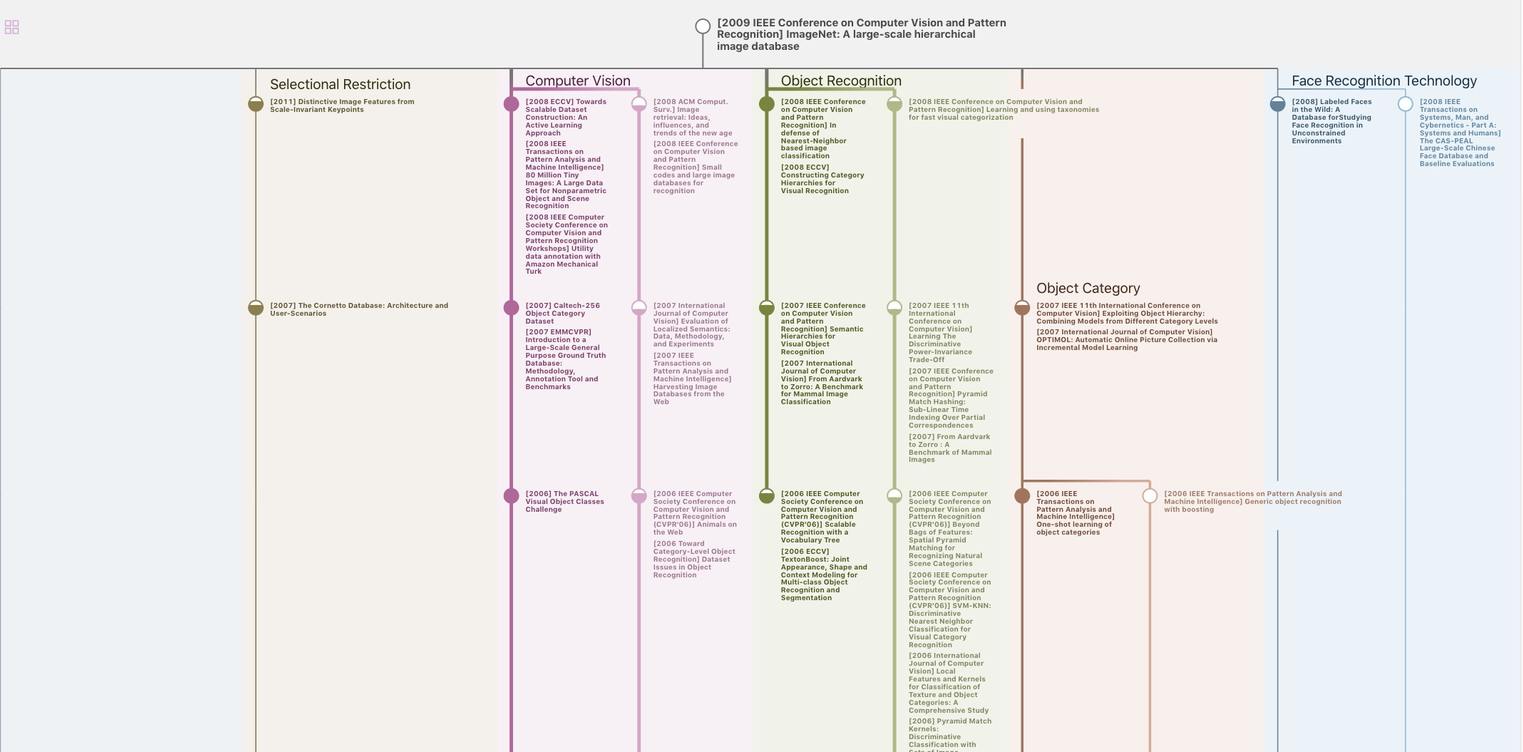
生成溯源树,研究论文发展脉络
Chat Paper
正在生成论文摘要