Poster Abstract: Safety Guaranteed Preference Learning Approach for Autonomous Vehicles.
HSCC '23: Proceedings of the 26th ACM International Conference on Hybrid Systems: Computation and Control(2023)
摘要
In this work, we propose a safety-guaranteed personalization for autonomous vehicles by incorporating Signal Temporal Logic (STL) into preference learning problem. We propose a new variant of STL called Parametric Weighted Signal Temporal Logic with a new quantitative semantics, namely weighted robustness. Given a set of pairwise preferences, and by using gradient-based optimization methods, we learn a set of valuations for weights that reflect preferences such that preferred ones have greater weighted robustness value than their non-preferred matches. Traditional STL formulas fail to incorporate preferences due its complex nature. Our initial results with data from a human-subject on an intersection with stop sign driving scenario, in which the participant is asked their preferred driving behavior from pairs of vehicle trajectories, indicate that we can learn a new weighted STL formula that captures preferences while also encoding correctness.
更多查看译文
AI 理解论文
溯源树
样例
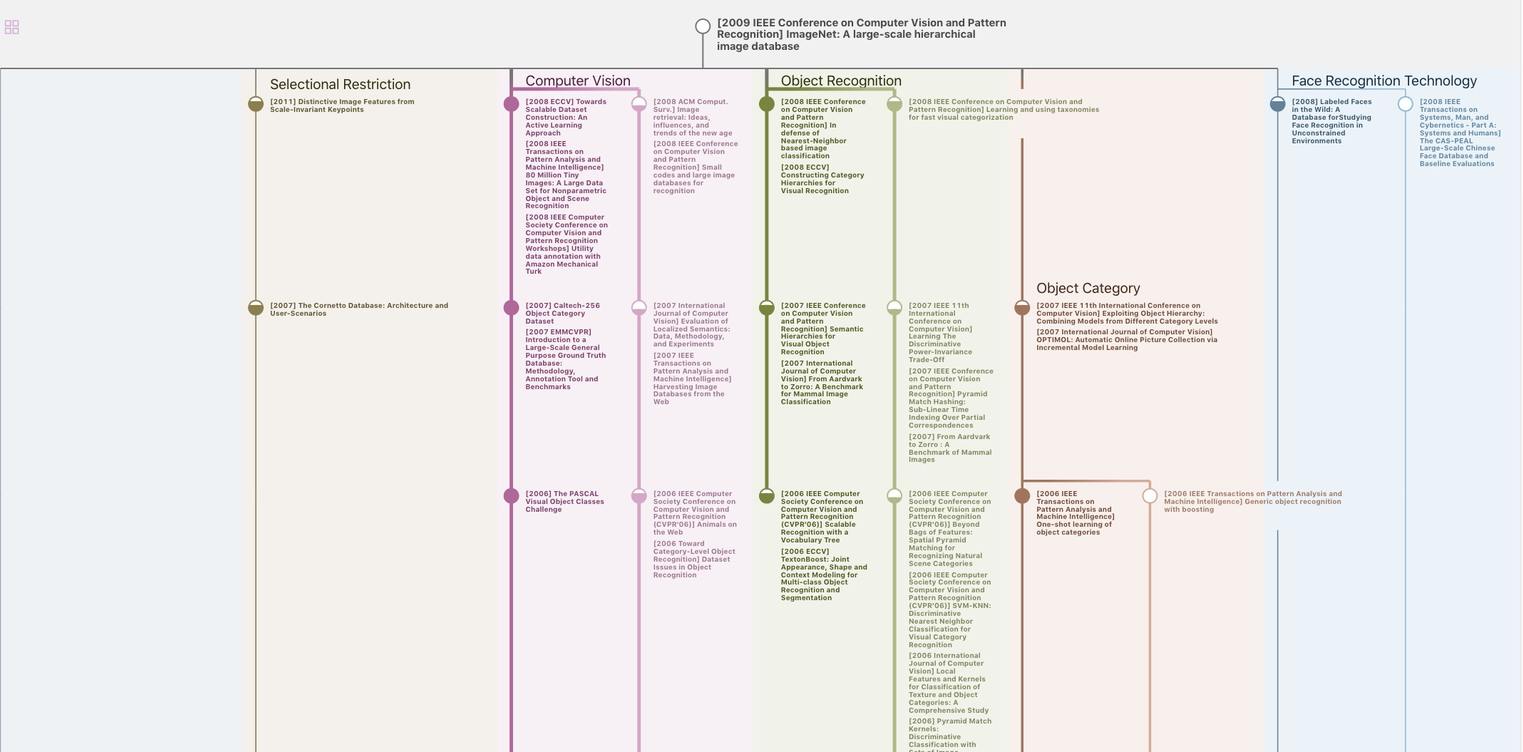
生成溯源树,研究论文发展脉络
Chat Paper
正在生成论文摘要