Self-supervised Pre-training and Semi-supervised Learning for Extractive Dialog Summarization
COMPANION OF THE WORLD WIDE WEB CONFERENCE, WWW 2023(2023)
摘要
Language model pre-training has led to state-of-the-art performance in text summarization. While a variety of pre-trained transformer models are available nowadays, they are mostly trained on documents. In this study we introduce self-supervised pre-training to enhance the BERT model's semantic and structural understanding of dialog texts from social media. We also propose a semi-supervised teacher-student learning framework to address the common issue of limited available labels in summarization datasets. We empirically evaluate our approach on extractive summarization task with the TWEETSUMM corpus, a recently introduced dialog summarization dataset from Twitter customer care conversations and demonstrate that our self-supervised pre-training and semi-supervised teacher-student learning are both beneficial in comparison to other pre-trained models. Additionally, we compare pre-training and teacher-student learning in various low data-resource settings, and find that pre-training outperforms teacher-student learning and the differences between the two are more significant when the available labels are scarce.
更多查看译文
关键词
summarization,twitter,dialog,self-supervised pre-training,semi-supervised learning
AI 理解论文
溯源树
样例
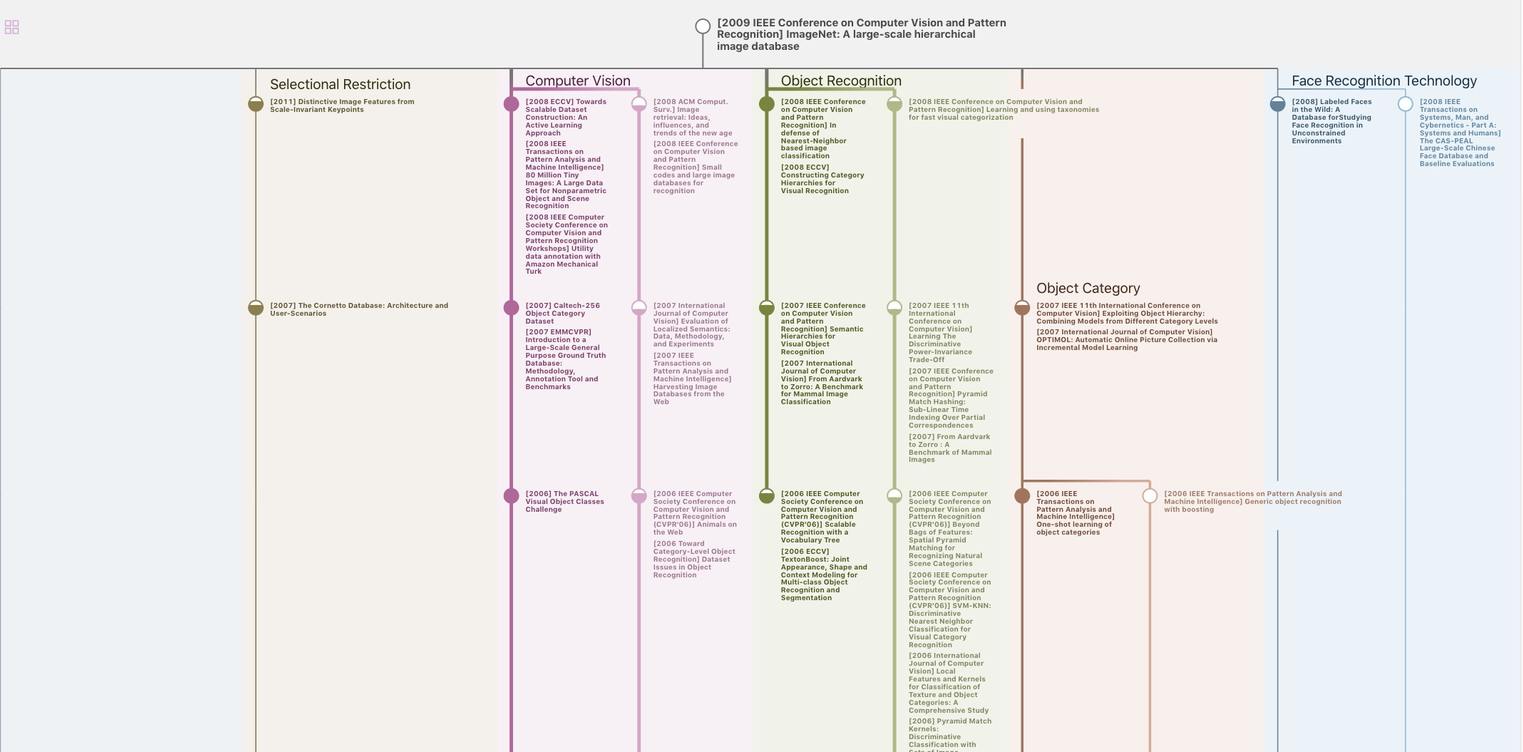
生成溯源树,研究论文发展脉络
Chat Paper
正在生成论文摘要