Trust the Process: Analyzing Prospective Provenance for Data Cleaning
COMPANION OF THE WORLD WIDE WEB CONFERENCE, WWW 2023(2023)
摘要
In the feld of data-driven research and analysis, the quality of results largely depends on the quality of the data used. Data cleaning is a crucial step in improving the quality of data. Still, it is equally important to document the steps made during the data cleaning process to ensure transparency and enable others to assess the quality of the resulting data. While provenance models such as W3C PROV have been introduced to track changes and events related to any entity, their use in documenting the provenance of datacleaning workfows can be challenging, particularly when mixing diferent types of documents or entities in the model. To address this, we propose a conceptual model and analysis that breaks down data-cleaning workfows into process abstraction and workfow recipes, refning operations to the column level. This approach provides users with detailed provenance information, enabling transparency, auditing, and support for data cleaning workfow improvements. Our model has several features that allow static analysis, e.g., to determine the minimal input schema and expected output schema for running a recipe, to identify which steps violate the column schema requirement constraint, and to assess the reusability of a recipe on a new dataset. We hope that our model and analysis will contribute to making data processing more transparent, accessible, and reusable.
更多查看译文
关键词
Data cleaning,transparency,provenance,workfow,provenance analysis
AI 理解论文
溯源树
样例
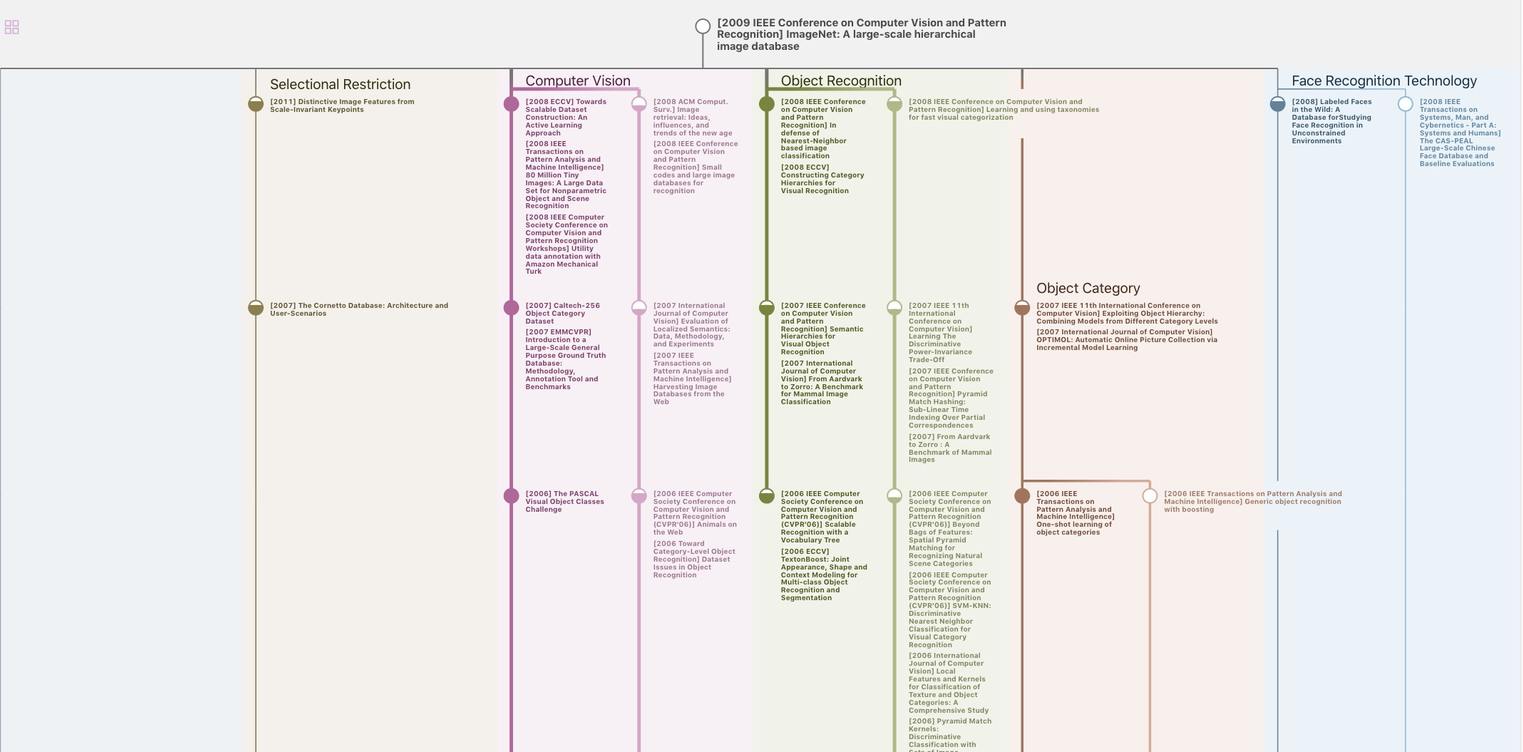
生成溯源树,研究论文发展脉络
Chat Paper
正在生成论文摘要