Understanding the Impact of Label Skewness and Optimization on Federated Learning for Text Classification
COMPANION OF THE WORLD WIDE WEB CONFERENCE, WWW 2023(2023)
摘要
Federated Learning (FL), also known as collaborative learning, is a distributed machine learning approach that collaboratively learns a shared prediction model without explicitly sharing private data. When dealing with sensitive data, privacy measures need to be carefully considered. Optimizers have a massive role in accelerating the learning process given the high dimensionality and non-convexity of the search space. The data partitioning in FL can be assumed to be either IID (independent and identically distributed) or non-IID. In this paper, we experiment with the impact of applying different adaptive optimization methods for FL frameworks in both IID and non-IID setups. We analyze the effects of label and quantity skewness, learning rate, and local client training on the learning process of optimizers as well as the overall performance of the global model. We evaluate the FL hyperparameter settings on biomedical text classification tasks on two datasets ADE V2 (Adverse Drug Efect: 2 classes) and Clinical-Trials (Reasons to stop trials: 17 classes).
更多查看译文
关键词
Federated Learning,Adaptive Optimizers,Privacy Secure Learning,non-IID,Text Classification
AI 理解论文
溯源树
样例
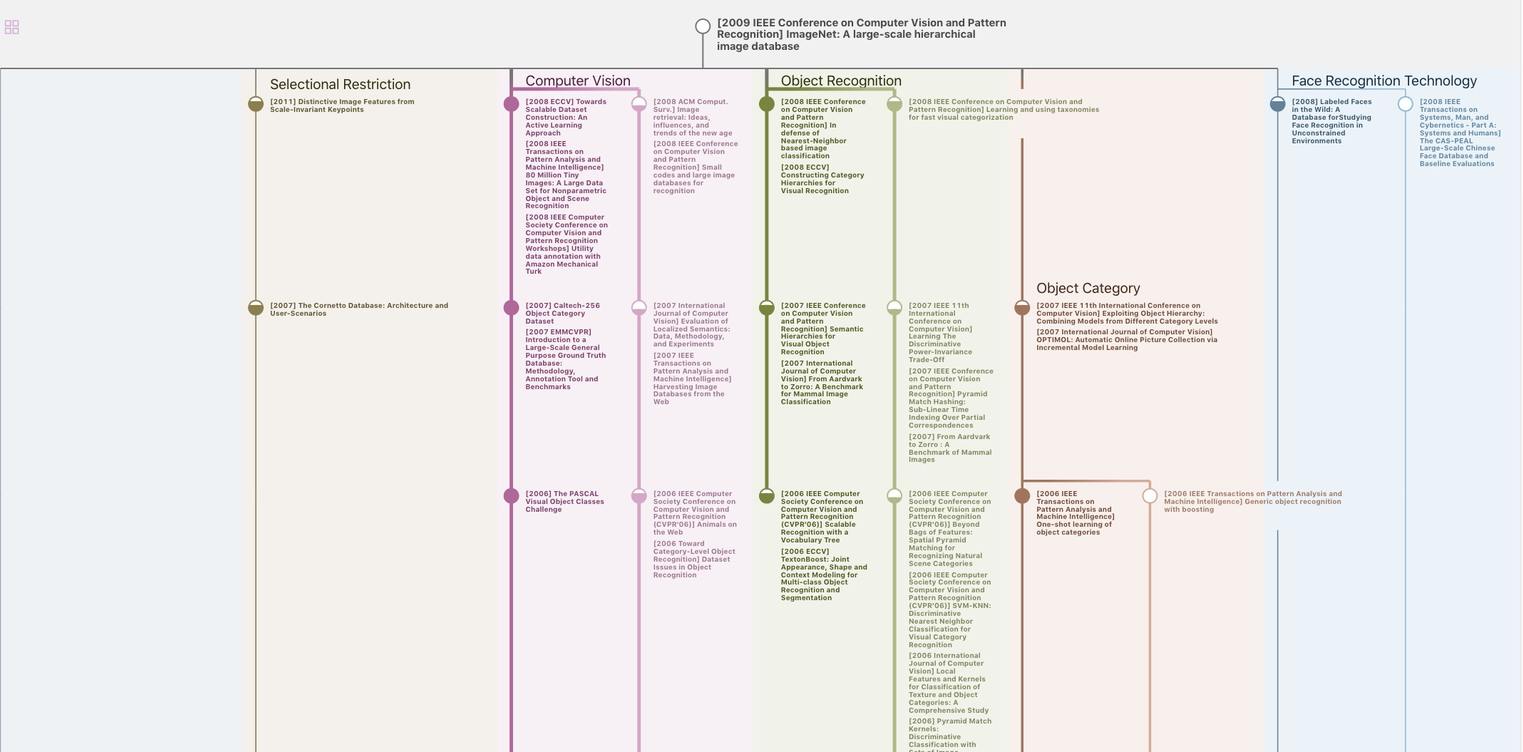
生成溯源树,研究论文发展脉络
Chat Paper
正在生成论文摘要