Semi-supervised medical imaging segmentation with soft pseudo-label fusion
Applied Intelligence(2023)
摘要
Segmentation is an essential task in modern medical imaging analysis. Since the scarcity of labeled pixel-level annotations often limits its wide applications, recent studies have proposed Semi-supervised Learning (SSL) frameworks to tackle this issue. Among them, the paradigm of pseudo-labeling, derived from SSL of natural images, has been popularly transferred on various medical datasets. Despite its promising results, we observe that many medical images’ regions are ambiguous, where pixels are challenging to be categorized as a specific class compared to natural images. Constructing hard pseudo-labels for these regions is consequently unintuitive and prone to be of low quality. To this end, we develop a novel SSL framework with the proposed Soft Pseudo-label Fusion strategy (called ”SPFSeg”). It can produce refined soft pseudo-labels, harboring the association knowledge between regions of interest (ROIs) and backgrounds while preserving the ”low-density” assumption of vanilla pseudo-labeling. These soft pseudo-labels can further establish potent supervision signals for unlabeled images, helping the segmentation model learn better feature representations. Through extensive experiments conducted on various datasets to evaluate the effectiveness of SPFSeg, our results manifest that its performance can surpass previous state-of-the-art semi-supervised frameworks on CXR-2014, ISIC-2017, and BUL-2020.
更多查看译文
关键词
Medical imaging segmentation,Semi-supervised learning,Soft pseudo-labeling
AI 理解论文
溯源树
样例
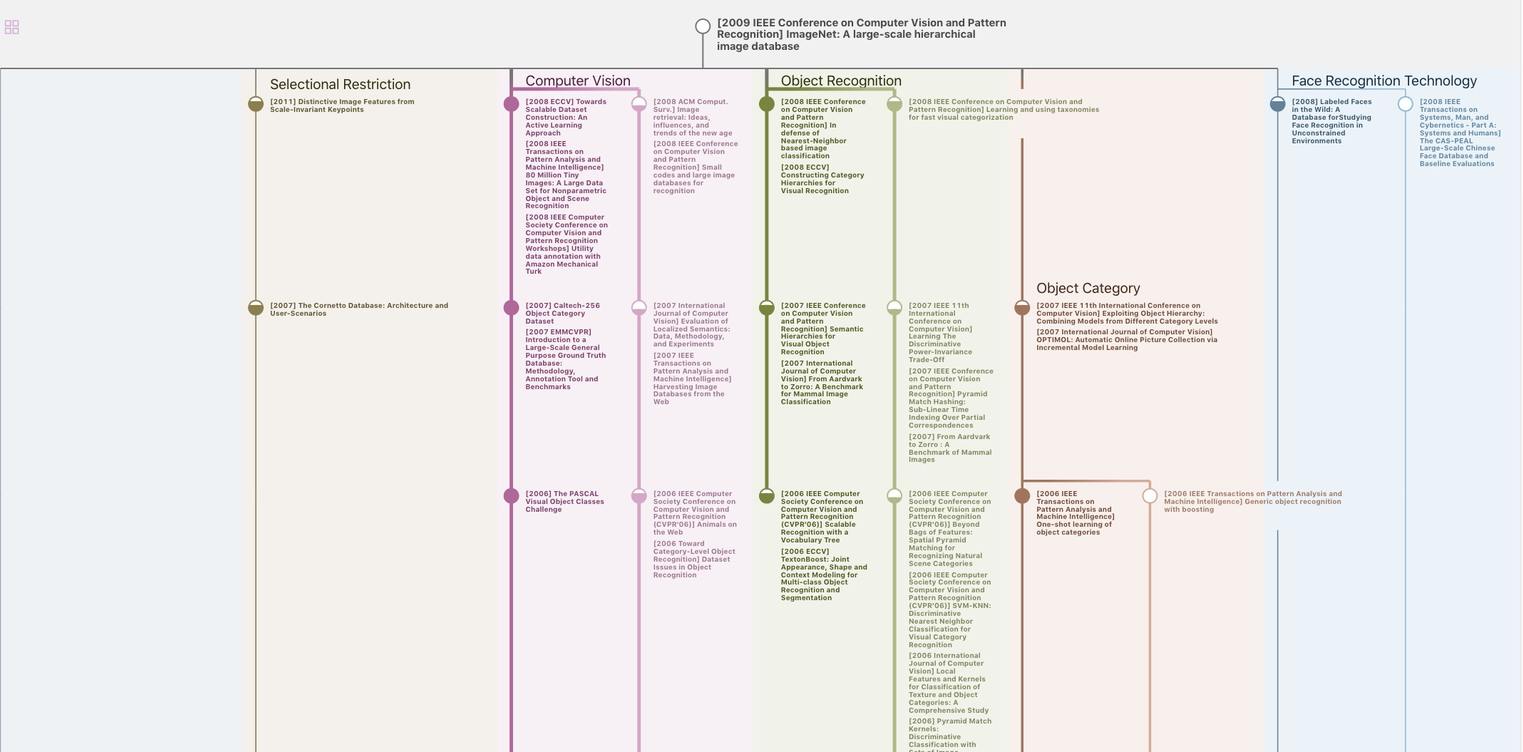
生成溯源树,研究论文发展脉络
Chat Paper
正在生成论文摘要