MEMER - Multimodal Encoder for Multi-signal Early-stage Recommendations
COMPANION OF THE WORLD WIDE WEB CONFERENCE, WWW 2023(2023)
摘要
Millions of content gets created daily on platforms like YouTube, Facebook, TikTok etc. Most of such large scale recommender systems are data demanding, thus taking substantial time for content embedding to mature. This problem is aggravated when there is no behavioral data available for new content. Poor quality recommendation for these items lead to user dissatisfaction and short content shelf-life. In this paper we propose a solution MEMER (Multimodal Encoder for Multi-signal Early-stage Recommendations), that utilises the multimodal semantic information of content and uses it to generate better quality embeddings for early-stage items. We demonstrate the flexibility of the framework by extending it to various explicit and implicit user actions. Using these learnt embeddings, we conduct offline and online experiments to verify its effectiveness. The predicted embeddings show significant gains in online early-stage experiments for both videos and images (videos: 44% relative gain in click through rate, 46% relative gain in explicit engagements, 9% relative gain in successful video play, 20% relative reduction in skips, images: 56% relative gain in explicit engagements). This also compares well against the performance of mature embeddings (83.3% RelaImpr (RI) [18] in Successful Video Play, 97.8% RelaImpr in Clicks).
更多查看译文
关键词
Recommendations,Early stage,Multimodal Semantic
AI 理解论文
溯源树
样例
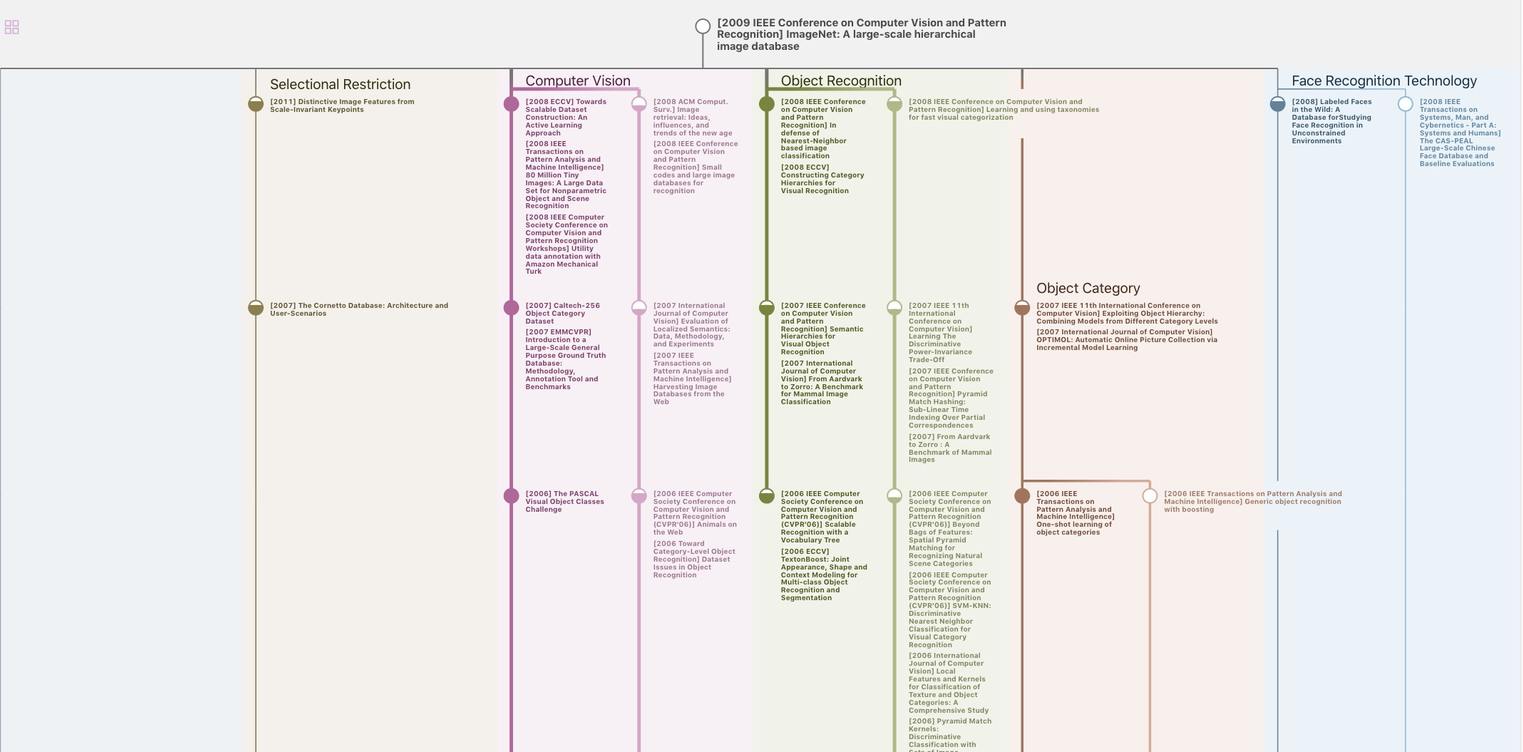
生成溯源树,研究论文发展脉络
Chat Paper
正在生成论文摘要