Efficient prediction of thermal history in wire and arc additive manufacturing combining machine learning and numerical simulation
The International Journal of Advanced Manufacturing Technology(2023)
摘要
Among metallic additive manufacturing technologies, wire and arc additive manufacturing (WAAM) has been recently adopted to manufacture large industrial components. In this process, controlling the temperature evolution is very important since it directly influences the quality of the deposited parts. Typically, the temperature history in WAAM can be obtained through experiments and/or numerical simulations, which are generally time-consuming and expensive. In this research, we developed a robust surrogate model (SM) for predicting the temperature history in WAAM based on the combination of machining learning (ML) and finite element (FE) simulation. The SM model was built to predict the temperature history in the WAAM of single weld tracks. For this purpose, the FE model was first developed and validated against experiments. The FE model was subsequently used to generate the data to train ML models based on feed-forward neural network (FFNN). The trained SM model can fast and accurately predict the temperature history in the cases which were not previously used for training with a very high accuracy of more than 99% and in a very short time with only 38 s (after being trained) as compared with 5 h for a FE model. The trained SM can be used for studies that require a large number of simulations such as uncertainty quantification or process optimization.
更多查看译文
关键词
Wire and arc additive manufacturing (WAAM),Surrogate model,Finite element simulation,Machine learning,Thermal history,316L
AI 理解论文
溯源树
样例
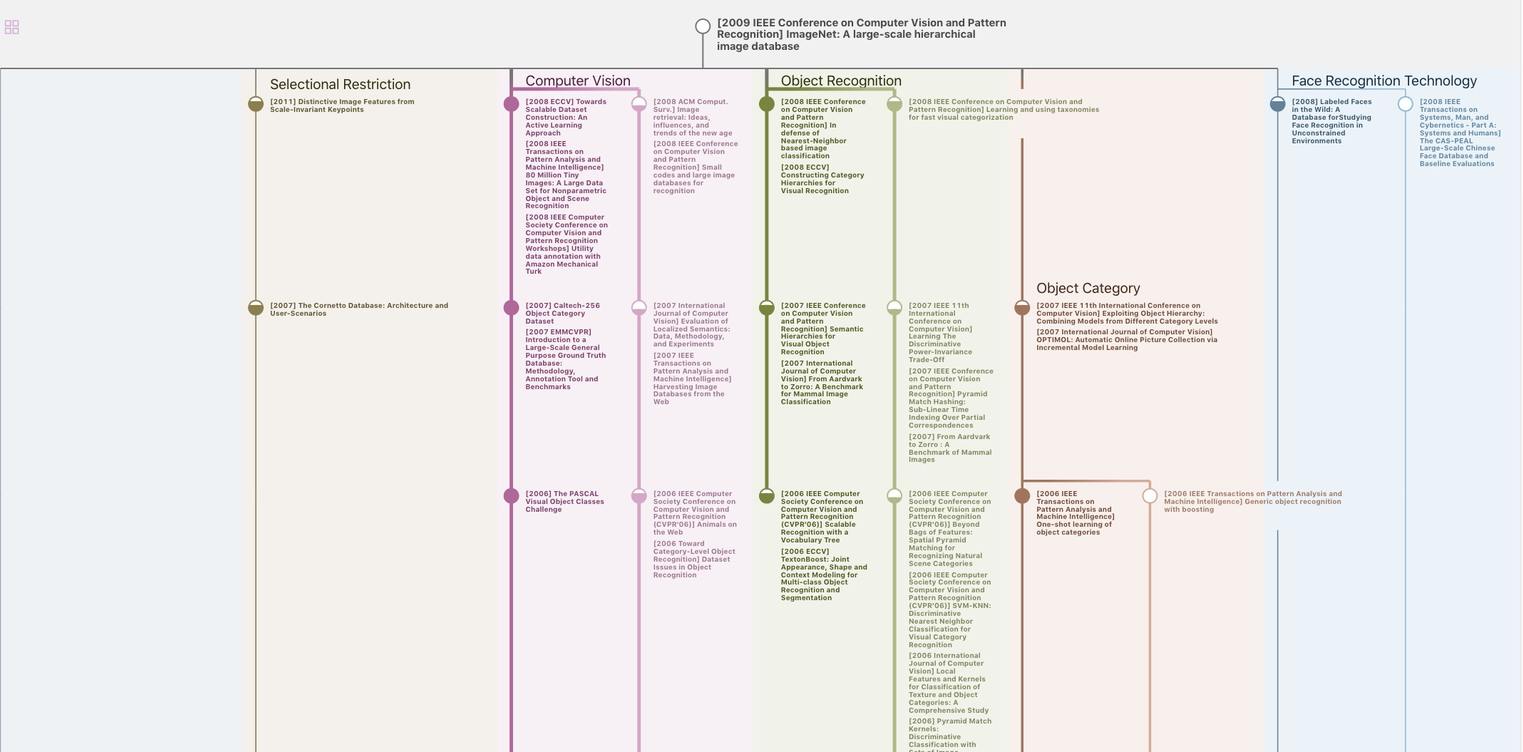
生成溯源树,研究论文发展脉络
Chat Paper
正在生成论文摘要