The Effect of Human Body Shadowing in ZigBee Radio Frequency Fingerprinting Identification
2022 International Conference on Artificial Intelligence of Things (ICAIoT)(2022)
摘要
Security is a major concern for the Internet of Medical Things (IoMT), however, many of these devices are limited in their capabilities. Physical layer (PHY) security measures can be used to prevent unauthorized access by exploiting intrinsic emitter characteristics such as Specific Emitter Identification (SEI), known as Radio Frequency Fingerprinting Identification (RFFI). RFFI at the IoMT is a promising approach to secure wearable Body Sensor Network (WBSN) devices. In this paper, we evaluated the effect of human body shadowing on Radio Frequency Fingerprinting Identification (RFFI) systems. Results show that shadowing has a serious impact on RFFI models. We also show that it can be mitigated by applying log-normal shadowing augmentation. Results obtained from simulations and experimental trials show that the classification accuracy increases when the multipath channel model and shadowing block size of 640 are used. A new system model for classifying devices using RFFI is then proposed. The proposed model achieved better classification accuracy when evaluated using unseen shadowed data.
更多查看译文
关键词
RF impairment,Deep learning,radio frequency fingerprint,emitter identification,ZigBee,device authentication
AI 理解论文
溯源树
样例
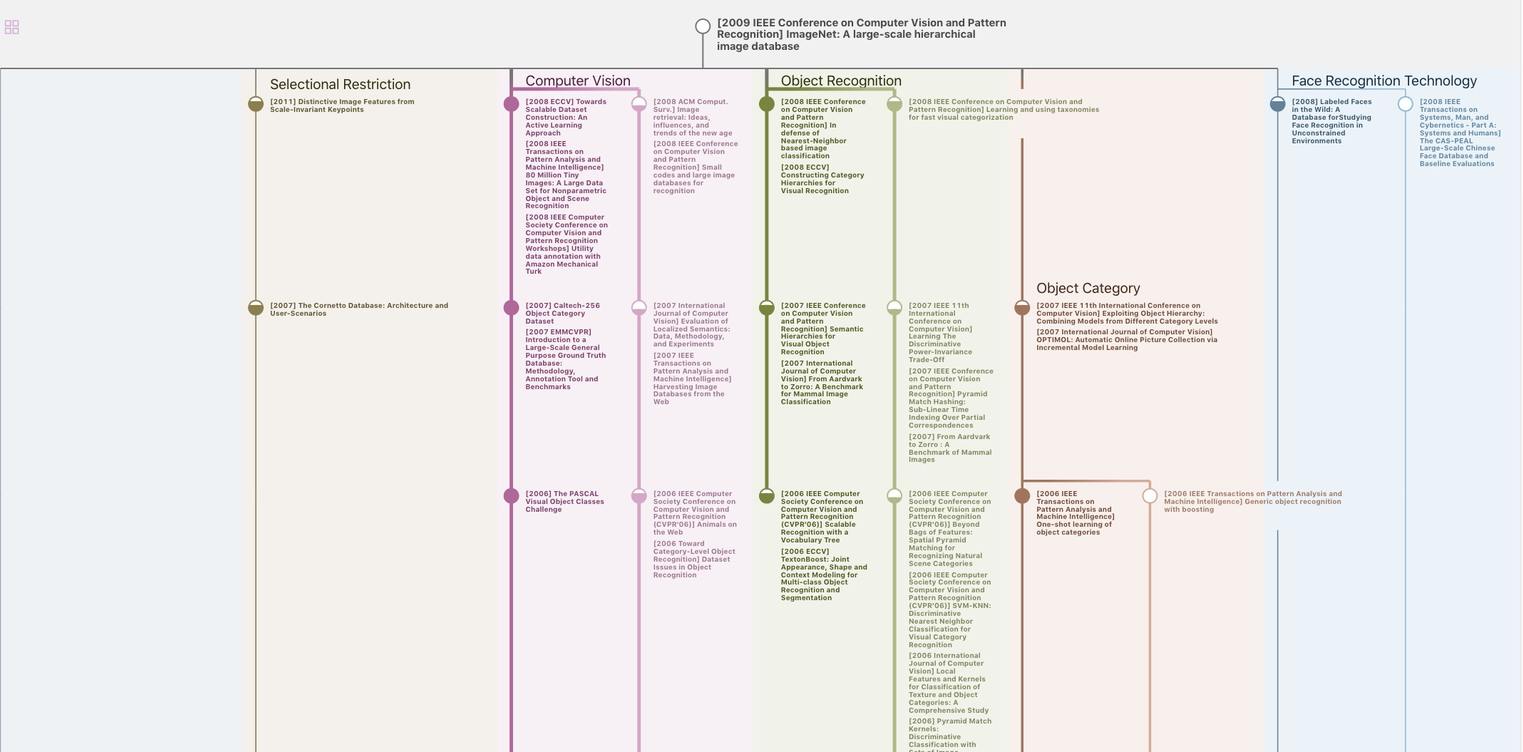
生成溯源树,研究论文发展脉络
Chat Paper
正在生成论文摘要