Emotion Recognition via Multiscale Feature Fusion Network and Attention Mechanism
IEEE Sensors Journal(2023)
摘要
Traditional manual feature-based machine learning methods and deep learning networks have been used for electroencephalogram (EEG)-based emotion recognition in recent years. However, some existing studies ignore the low signal-to-noise ratio and the fact that each subject has unique EEG traits, which suffer from low recognition accuracy and poor robustness. To solve these problems, we propose a novel attention mechanism-based multiscale feature fusion network (AM-MSFFN) that considers high-level features at different scales to improve the generalization of the model for different subjects. Specifically, we first utilize a spatial-temporal convolutional block to extract temporal and spatial features of EEG signals sequentially. Subsequently, considering the sampling rate of EEG signals, the multiscale separable convolutions are designed for capturing emotional state-related information, to better combine and output feature mapping relationships. Convolutional module attention mechanism (CBAM) is applied after point-wise convolution, to better handle EEG variations of different subjects and the key information which facilitates classification. In addition, we adopt a preprocessing module based on data augmentation and data alignment to improve the quality of the training samples. Moreover, ablation studies show that the proposed attention mechanism and multiscale separable convolution contribute significant and consistent gain to the performance of our AM-MSFFN model. To verify the effectiveness of the proposed algorithm, we conducted extensive experiments on the DEAP dataset and SEED. The average accuracies achieve 99.479% and 99.297% for arousal and valence, respectively. The results demonstrated the feasibility of the proposed method.
更多查看译文
关键词
Attention mechanism,deep learning,electroencephalogram (EEG),emotion recognition,feature fusion,spatial-temporal feature
AI 理解论文
溯源树
样例
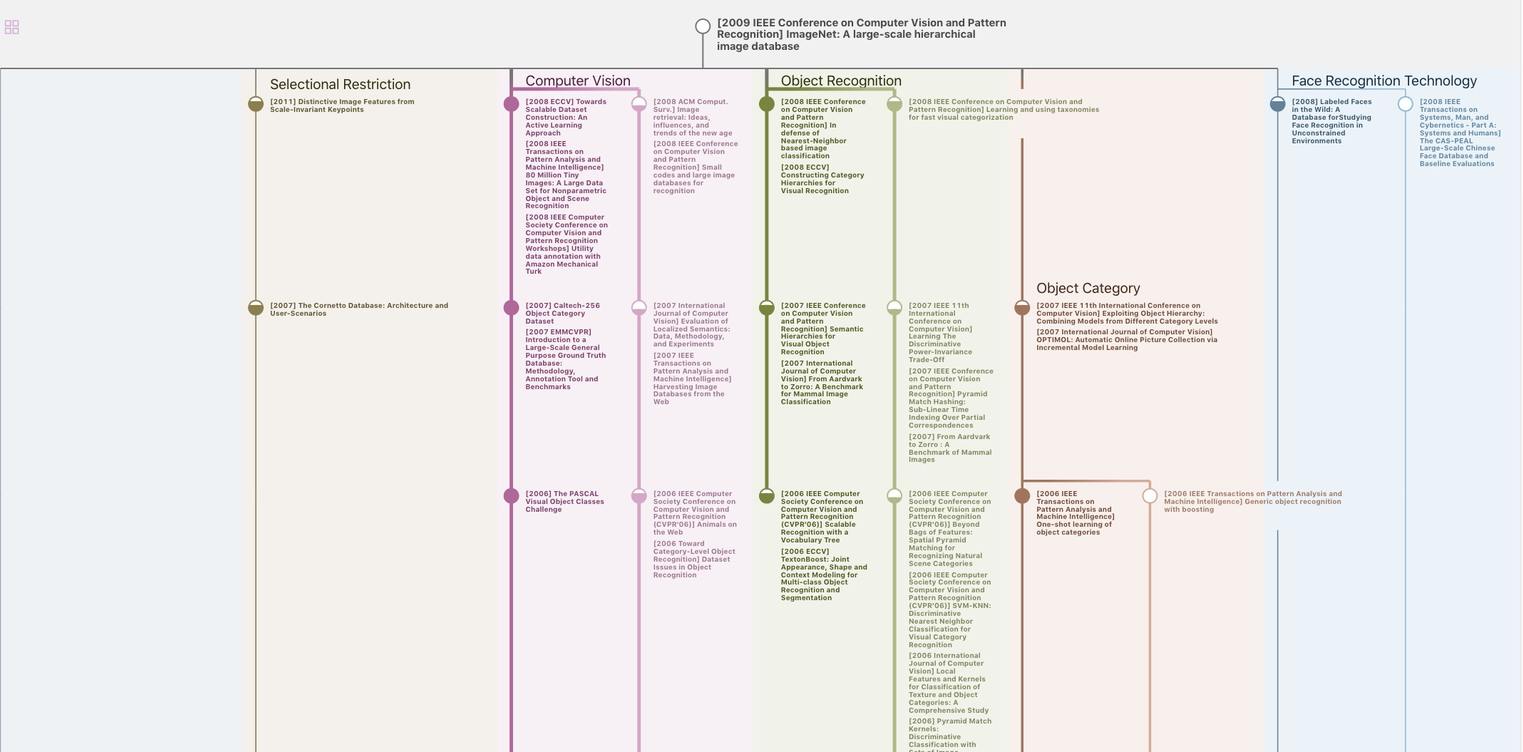
生成溯源树,研究论文发展脉络
Chat Paper
正在生成论文摘要