Vehicle Acoustic and Seismic Synchronization Signal Classification Using Long-Term Features
IEEE Sensors Journal(2023)
摘要
Field-vehicle-type recognition plays an essential role in border protection tasks. Acoustic and seismic sensors can effectively collect the signal of field vehicle targets in real-time. Most vehicle temporal signal classification algorithms are based on extracting and identifying handcrafted features. These algorithms focus on the signal’s frequency-domain characteristics and despise the signal’s time-domain characteristics. To extract appropriate features, this article proposes a long-term correlation feature network (LTCFN) to perform field vehicle acoustic and seismic signal classification. The model includes AlexNet-type feature extractor and an overall classifier implemented by a long-short term memory (LSTM) network. We present an intraframe network and fusion method for extracting feature vector from signals. Meanwhile, an interframe classifier is proposed first for analyzing the time correlation of the feature map and overall classification. The experiments illustrate that the LTCFN has excellent recognition performance and anti-noise ability. The classification accuracy of the LTCFN can be increased to 96%. This article also provides a new idea for ground target classification through interframe feature measurement.
更多查看译文
关键词
seismic synchronization signal classification,features,acoustic,long-term
AI 理解论文
溯源树
样例
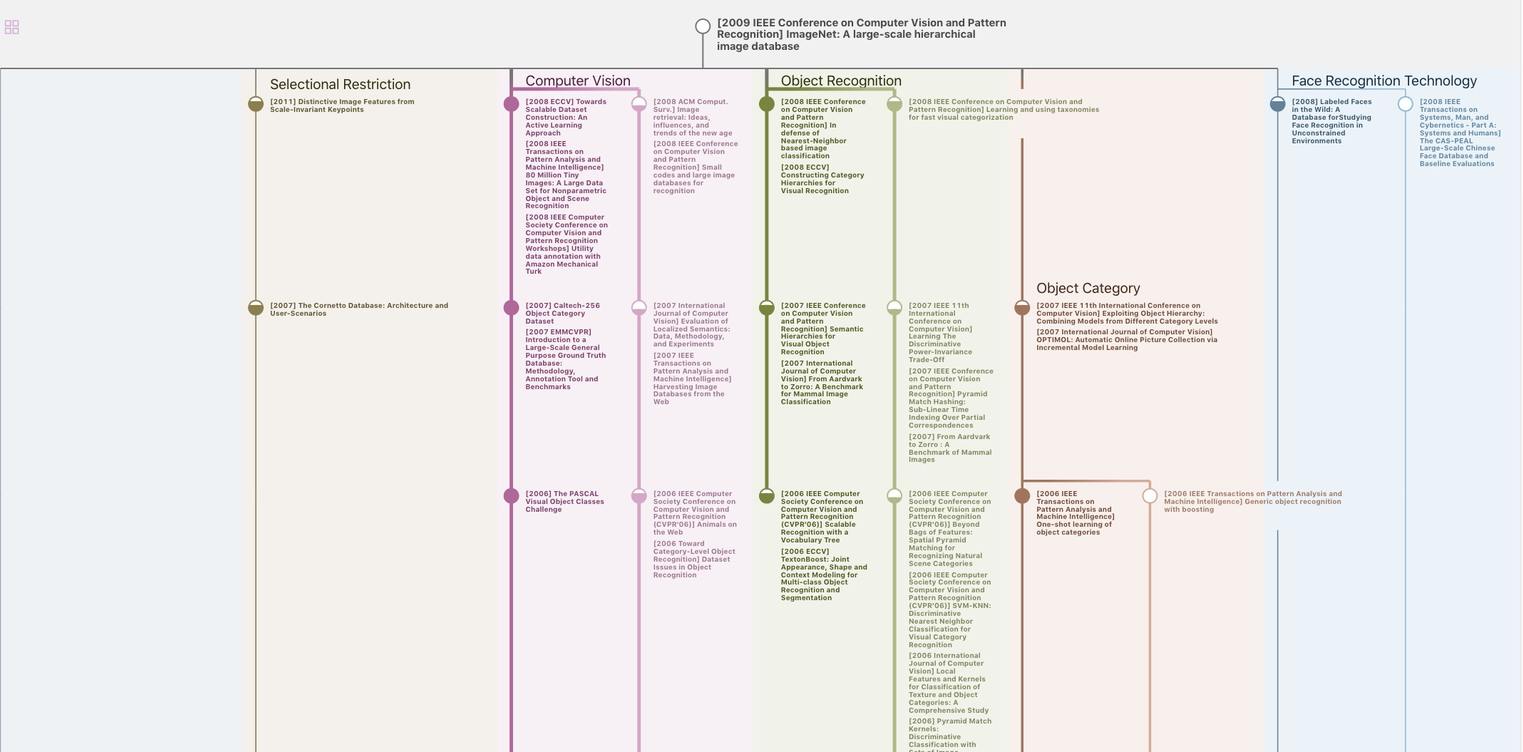
生成溯源树,研究论文发展脉络
Chat Paper
正在生成论文摘要