Fully Automatic Unsupervised Approach based on Adaptive Encoder Decoder Process for Forest Fire Image Denoising and Segmentation
2022 IEEE Information Technologies & Smart Industrial Systems (ITSIS)(2022)
摘要
Forest fire is a major problem that affect humans and natural resources. Early detecting forest fires in images depends on the appearance of the flame and smoke in the images, and their recognizing can help firefighters assessing the risk degree and monitor fires. Wildfire images resulting either from a fixed camera or a drone in motion are susceptible to noise. For good segmentation results a proper image analysis equipped with noise removal steps is often required. In this paper, a novel technique for denoising and segmenting forest fires images is proposed. The presented methodology consists of denoising images with a new convolutional auto-encoder architecture then segmenting the output image with an adaptive active contour model. The method shows good results quantitatively and visually. The proposed denoising auto-encoder was trained and validated using a small dataset containing 450 images and succeed by removing the noise and also improving the brightness in the forest fire image, which leads to very promising segmentation results with a region-based active contour method.
更多查看译文
关键词
forest fire image segmentation,denoising images,auto-encoders,unsupervised learning,active contour models
AI 理解论文
溯源树
样例
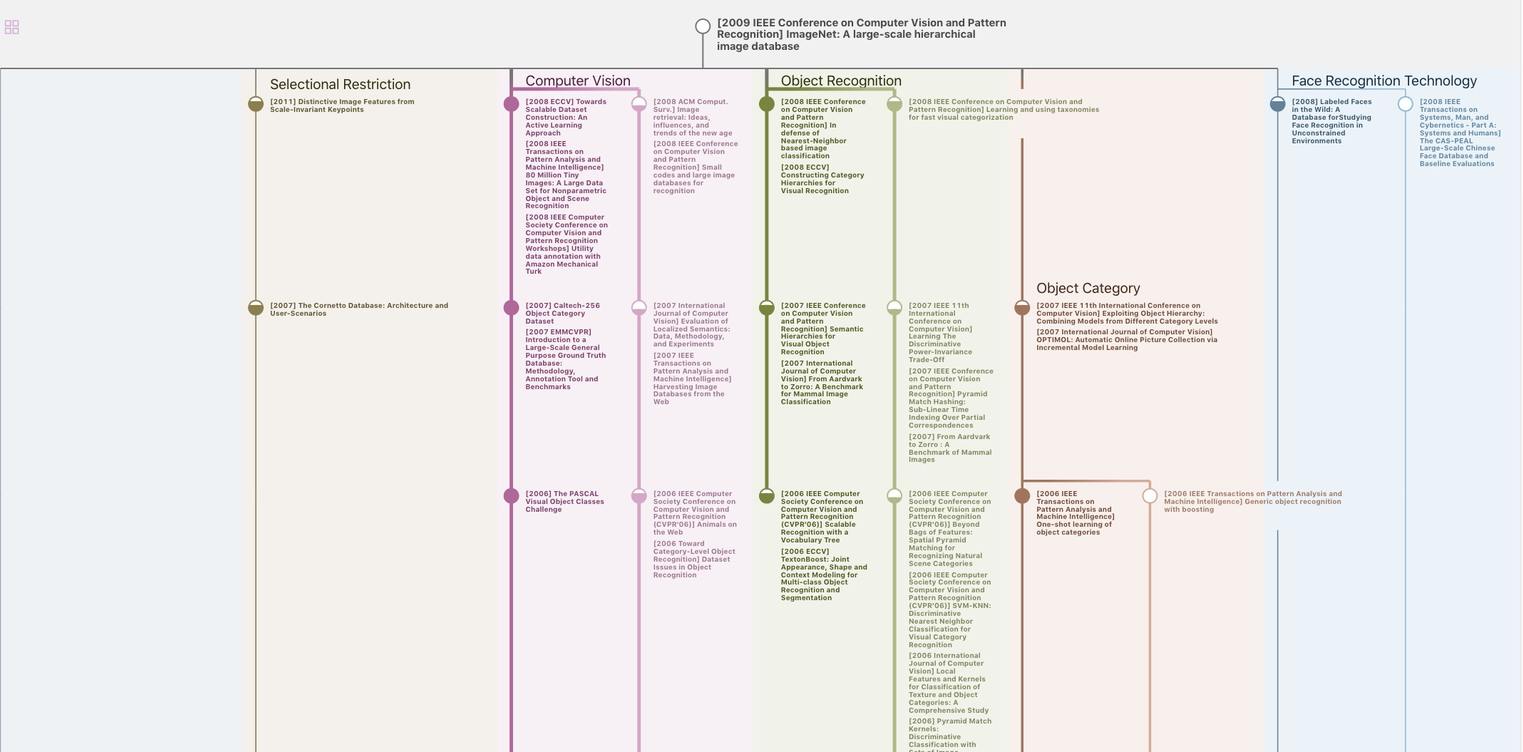
生成溯源树,研究论文发展脉络
Chat Paper
正在生成论文摘要