YOLOv4 and Branch Attention: an Improved Approach to Real-Time Object Detection
2022 IEEE Information Technologies & Smart Industrial Systems (ITSIS)(2022)
摘要
We propose a new enhanced YOLOv4-based model that outperforms the accuracy of YOLOv4 without considerably compromising its speed. We study the implementation of Selective Kernel attention mechanism and make extensive experiments to reliably optimize it. We explore several optimization techniques, mainly grouped, depth-wise separable and dilated convolution. We introduce selective kernel convolution in CSPdarknet53 which is based on multiple branches with different kernel sizes. Our implementation allows the neurons to dynamically adjust their receptive fields. This version of YOLOv4 enables the network to adaptively choose the appropriate kernel size according to the input. Experiments show that our model improved YOLOv4 by 1.8% with a negligible increase in inference time. We were also able to decrease the GFLOPs and parameters of YOLOv4 making the training process more efficient. Our model achieves 80.2% accuracy on the dataset Pascal VOC at a real time speed of 64 FPS on a single Tesla P100 GPU.
更多查看译文
关键词
object detection,CNN,YOLO,selective kernel
AI 理解论文
溯源树
样例
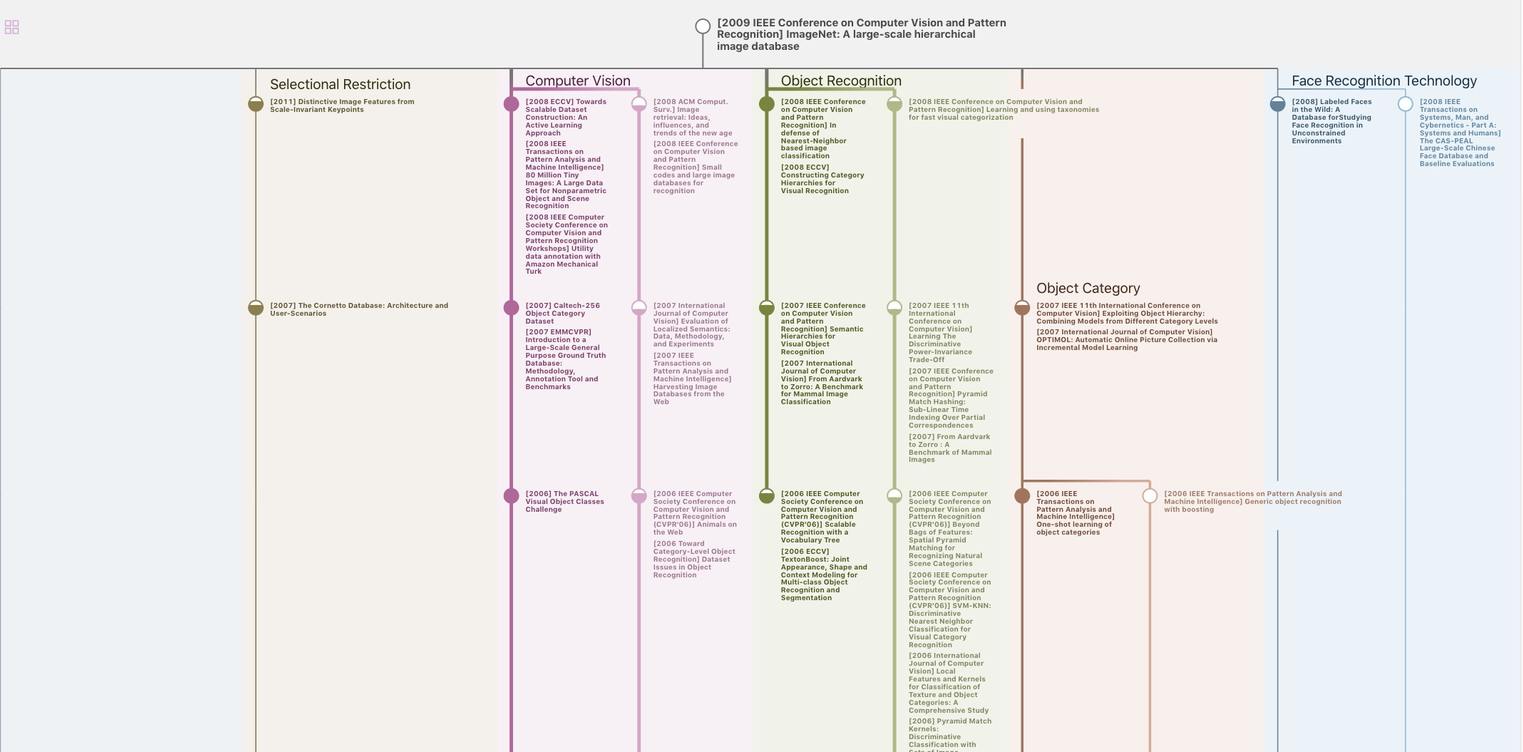
生成溯源树,研究论文发展脉络
Chat Paper
正在生成论文摘要