Test Input Selection for Deep Neural Network Enhancement Based on Multiple-Objective Optimization.
2023 IEEE INTERNATIONAL CONFERENCE ON SOFTWARE ANALYSIS, EVOLUTION AND REENGINEERING, SANER(2023)
摘要
Deep Neural Networks (DNNs) have been applied in many domains, such as autonomous driving and image recognition. However, due to the lower-than-expected performance of the DNN models in the real application, researchers are committed to sampling a test subset from test data with the limited labeling effort to retrain the DNN models for enhancement. Existing test input selection methods aim at selecting the test inputs according to the probability that is classified incorrectly by the DNN model. However, the test inputs selected by using existing methods might have similar features, making the DNN model unable to learn more diverse features when retraining. To address this limitation, this paper proposes Multiple-Objective Optimization-Based Test Input Selection (MOTS) to select more effective test subset to retrain the DNN model for enhancement. Different from existing works, this work not only considers the uncertainty of the test input but also takes the diversity of the test subset into account. Then MOTS uses a multiple-objective optimization algorithm NSGA-II to solve this problem, which ensures the test subset has more diverse features and is more helpful for retraining DNN models. This paper conducts the experiment on two popular DNN models and three widely-used datasets. The experiment results indicate that MOTS achieves 114%, 72%, 55%, 41% average accuracy improvement under four different sampling ratios 1%, 3%, 5%, 10% compared with five baseline methods. Therefore, MOTS is very effective in improving the quality of the DNN models compared with the state-of-the-art methods and the diversity of the test subset contributes greatly to the effectiveness of retraining DNN models.
更多查看译文
关键词
deep neural network testing,test input selection,retraining,multiple-objective optimization
AI 理解论文
溯源树
样例
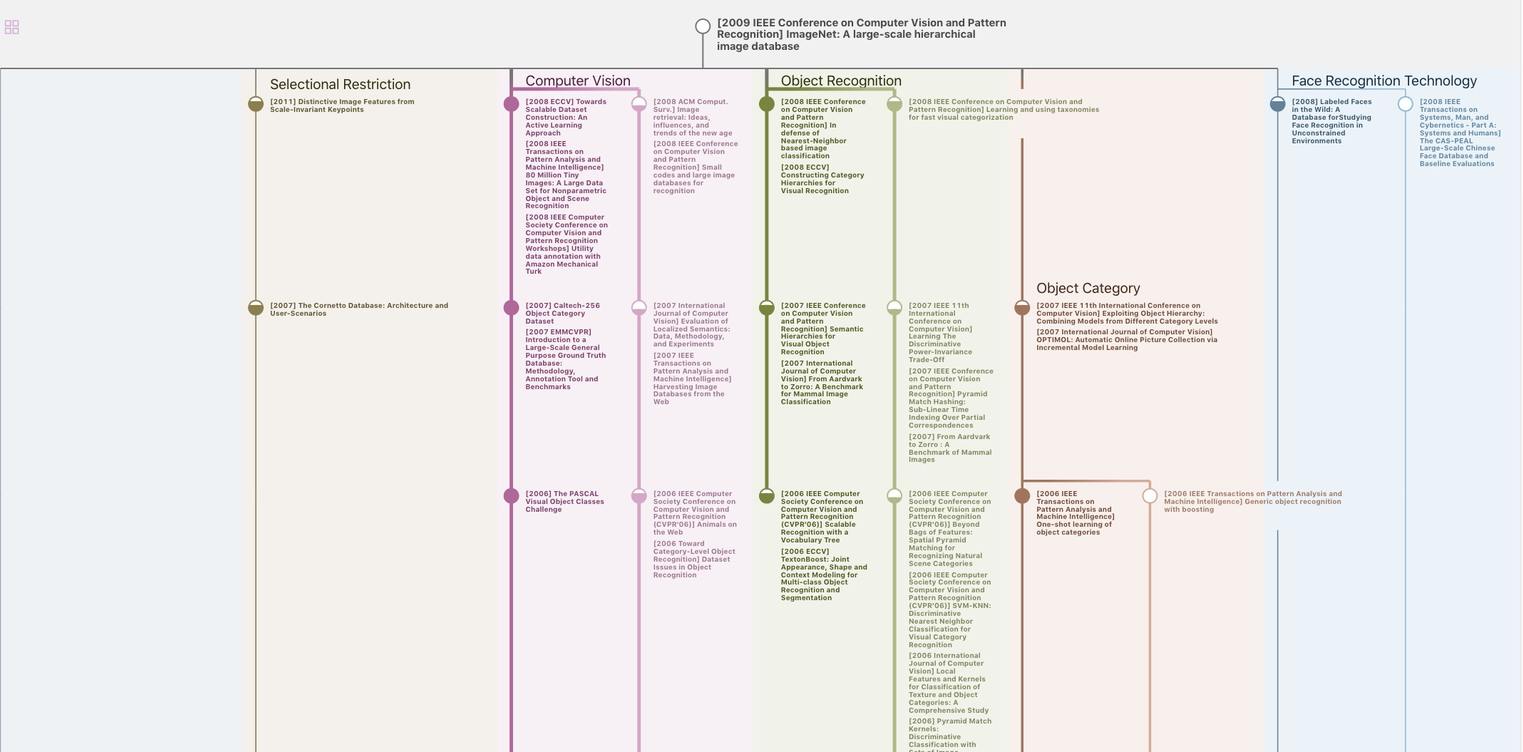
生成溯源树,研究论文发展脉络
Chat Paper
正在生成论文摘要