Survey of Malware Analysis through Control Flow Graph using Machine Learning
arXiv (Cornell University)(2023)
摘要
Malware is a significant threat to the security of computer systems and networks which requires sophisticated techniques to analyze the behavior and functionality for detection. Traditional signature-based malware detection methods have become ineffective in detecting new and unknown malware due to their rapid evolution. One of the most promising techniques that can overcome the limitations of signature-based detection is to use control flow graphs (CFGs). CFGs leverage the structural information of a program to represent the possible paths of execution as a graph, where nodes represent instructions and edges represent control flow dependencies. Machine learning (ML) algorithms are being used to extract these features from CFGs and classify them as malicious or benign. In this survey, we aim to review some state-of-the-art methods for malware detection through CFGs using ML, focusing on the different ways of extracting, representing, and classifying. Specifically, we present a comprehensive overview of different types of CFG features that have been used as well as different ML algorithms that have been applied to CFG-based malware detection. We provide an in-depth analysis of the challenges and limitations of these approaches, as well as suggest potential solutions to address some open problems and promising future directions for research in this field.
更多查看译文
关键词
malware analysis,control flow graph
AI 理解论文
溯源树
样例
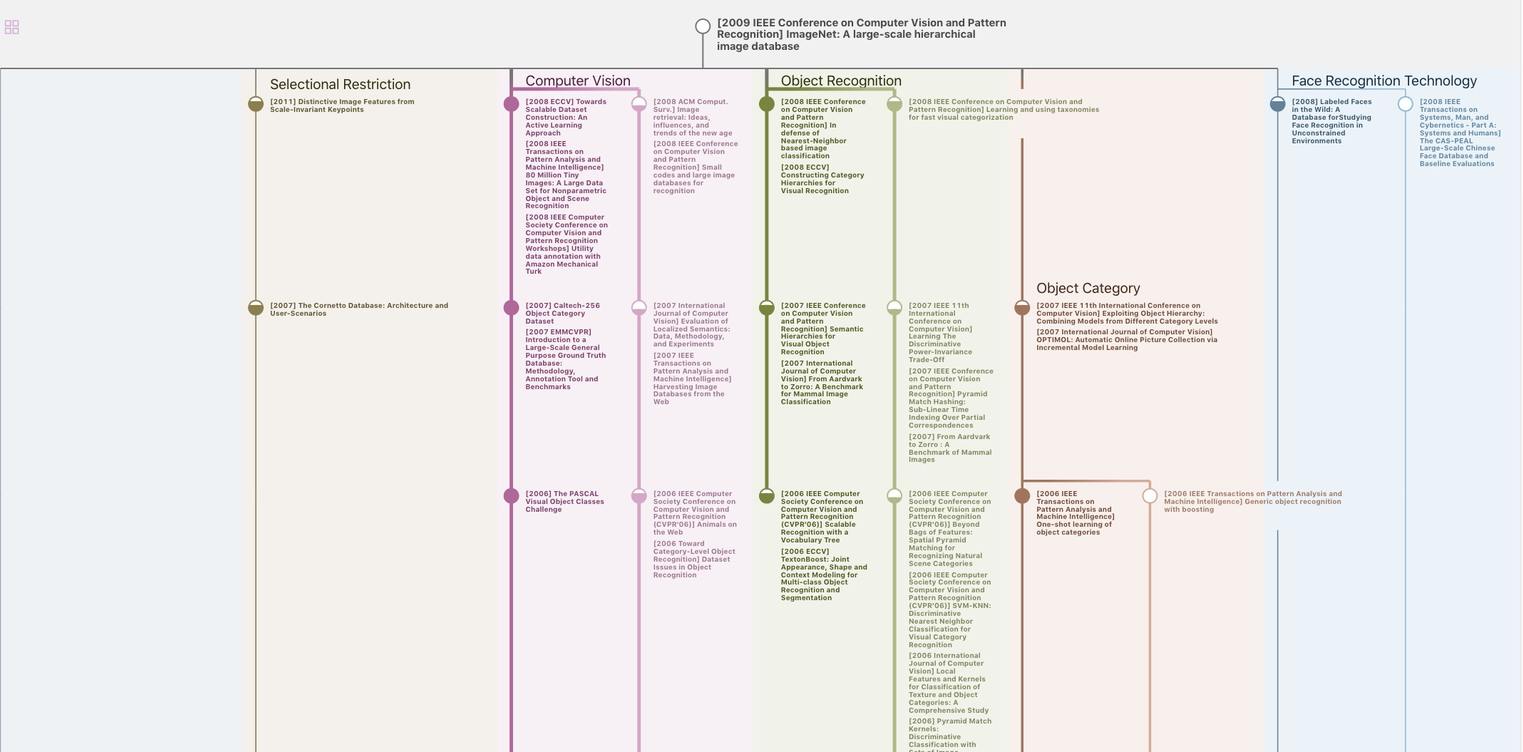
生成溯源树,研究论文发展脉络
Chat Paper
正在生成论文摘要