Physics-enhanced Gaussian Process Variational Autoencoder
CoRR(2023)
摘要
Variational autoencoders allow to learn a lower-dimensional latent space based on high-dimensional input/output data. Using video clips as input data, the encoder may be used to describe the movement of an object in the video without ground truth data (unsupervised learning). Even though the object's dynamics is typically based on first principles, this prior knowledge is mostly ignored in the existing literature. Thus, we propose a physics-enhanced variational autoencoder that places a physical-enhanced Gaussian process prior on the latent dynamics to improve the efficiency of the variational autoencoder and to allow physically correct predictions. The physical prior knowledge expressed as linear dynamical system is here reflected by the Green's function and included in the kernel function of the Gaussian process. The benefits of the proposed approach are highlighted in a simulation with an oscillating particle.
更多查看译文
关键词
gaussian,physics-enhanced
AI 理解论文
溯源树
样例
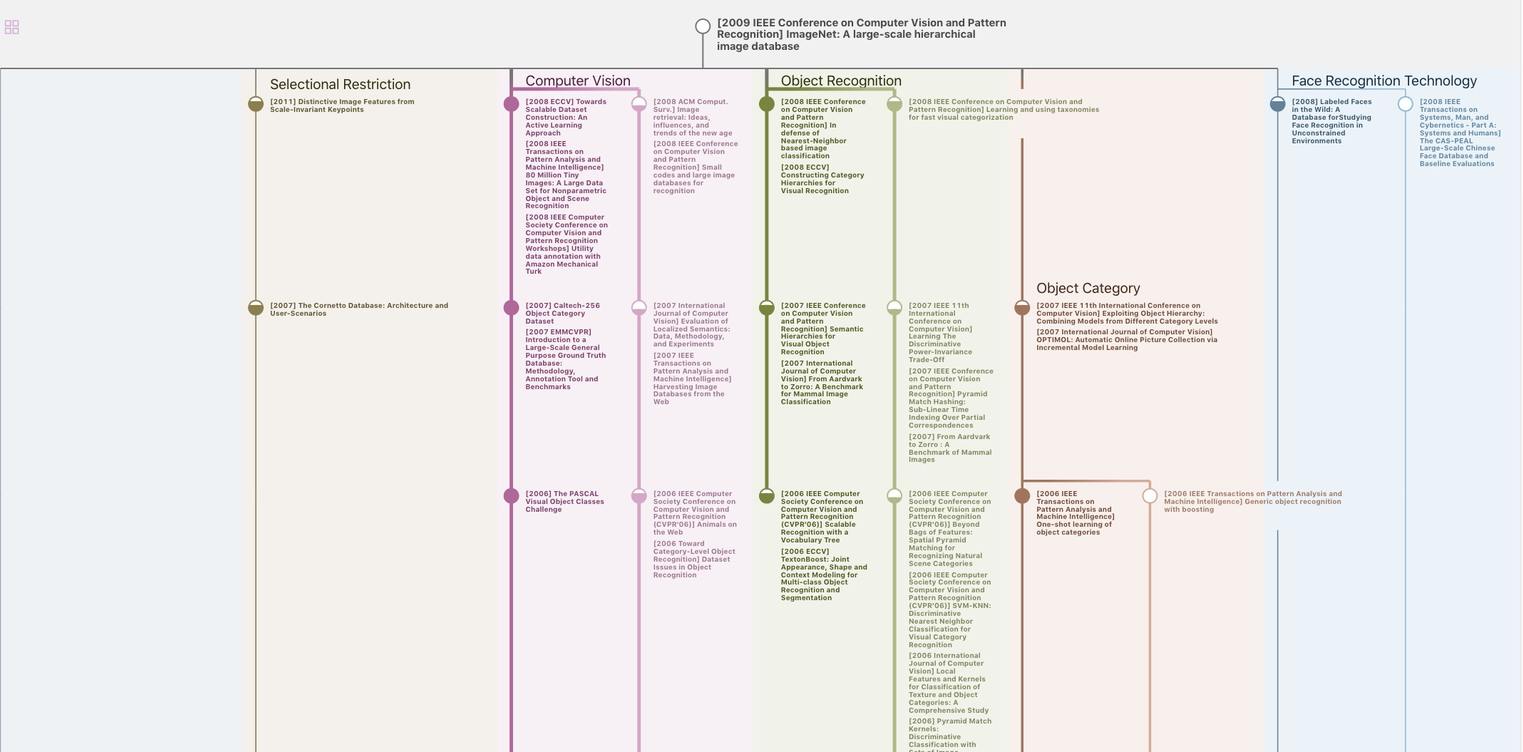
生成溯源树,研究论文发展脉络
Chat Paper
正在生成论文摘要