Toward Falsifying Causal Graphs Using a Permutation-Based Test
CoRR(2023)
摘要
Understanding the causal relationships among the variables of a system is paramount to explain and control its behaviour. Inferring the causal graph from observational data without interventions, however, requires a lot of strong assumptions that are not always realistic. Even for domain experts it can be challenging to express the causal graph. Therefore, metrics that quantitatively assess the goodness of a causal graph provide helpful checks before using it in downstream tasks. Existing metrics provide an absolute number of inconsistencies between the graph and the observed data, and without a baseline, practitioners are left to answer the hard question of how many such inconsistencies are acceptable or expected. Here, we propose a novel consistency metric by constructing a surrogate baseline through node permutations. By comparing the number of inconsistencies with those on the surrogate baseline, we derive an interpretable metric that captures whether the DAG fits significantly better than random. Evaluating on both simulated and real data sets from various domains, including biology and cloud monitoring, we demonstrate that the true DAG is not falsified by our metric, whereas the wrong graphs given by a hypothetical user are likely to be falsified.
更多查看译文
关键词
causal graphs,test,permutation-based
AI 理解论文
溯源树
样例
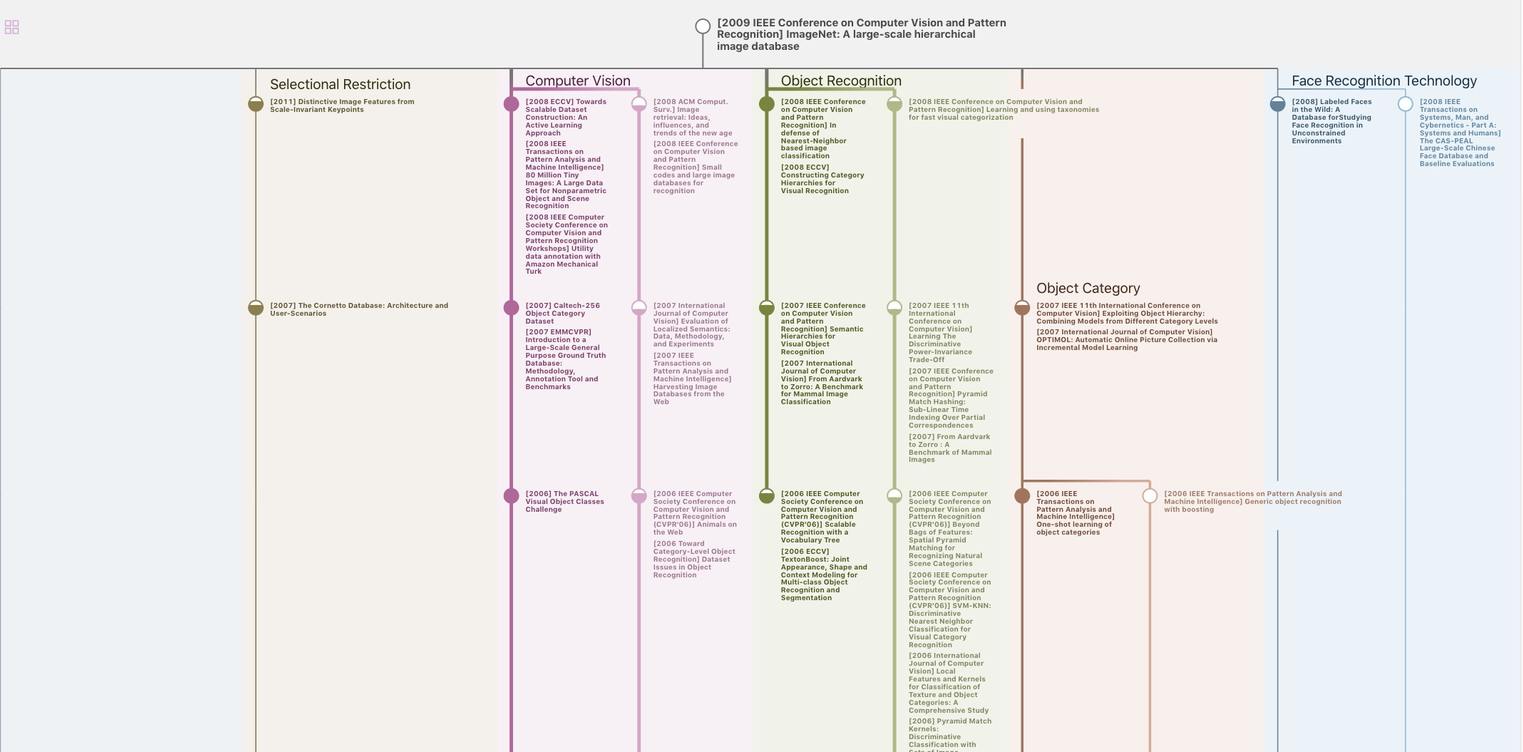
生成溯源树,研究论文发展脉络
Chat Paper
正在生成论文摘要