PEFAT: Boosting Semi-Supervised Medical Image Classification Via Pseudo-Loss Estimation and Feature Adversarial Training
CVPR 2023(2023)
摘要
Pseudo-labeling approaches have been proven beneficial for semi-supervised learning (SSL) schemes in computer vision and medical imaging. Most works are dedicated to finding samples with high-confidence pseudo-labels from the perspective of model predicted probability. Whereas this way may lead to the inclusion of incorrectly pseudo-labeled data if the threshold is not carefully adjusted. In addition, low-confidence probability samples are frequently disregarded and not employed to their full potential. In this paper, we propose a novel Pseudo-loss Estimation and Feature Adversarial Training semi-supervised framework, termed as PEFAT, to boost the performance of multi-class and multi-label medical image classification from the point of loss distribution modeling and adversarial training. Specifically, we develop a trustworthy data selection scheme to split a high-quality pseudo-labeled set, inspired by the dividable pseudo-loss assumption that clean data tend to show lower loss while noise data is the opposite. Instead of directly discarding these samples with low-quality pseudo-labels, we present a novel regularization approach to learn discriminate information from them via injecting adversarial noises at the feature-level to smooth the decision boundary. Experimental results on three medical and two natural image benchmarks validate that our PEFAT can achieve a promising performance and surpass other state-of-the-art methods. The code is available at https://github.com/maxwell0027/PEFAT.
更多查看译文
关键词
Medical and biological vision,cell microscopy
AI 理解论文
溯源树
样例
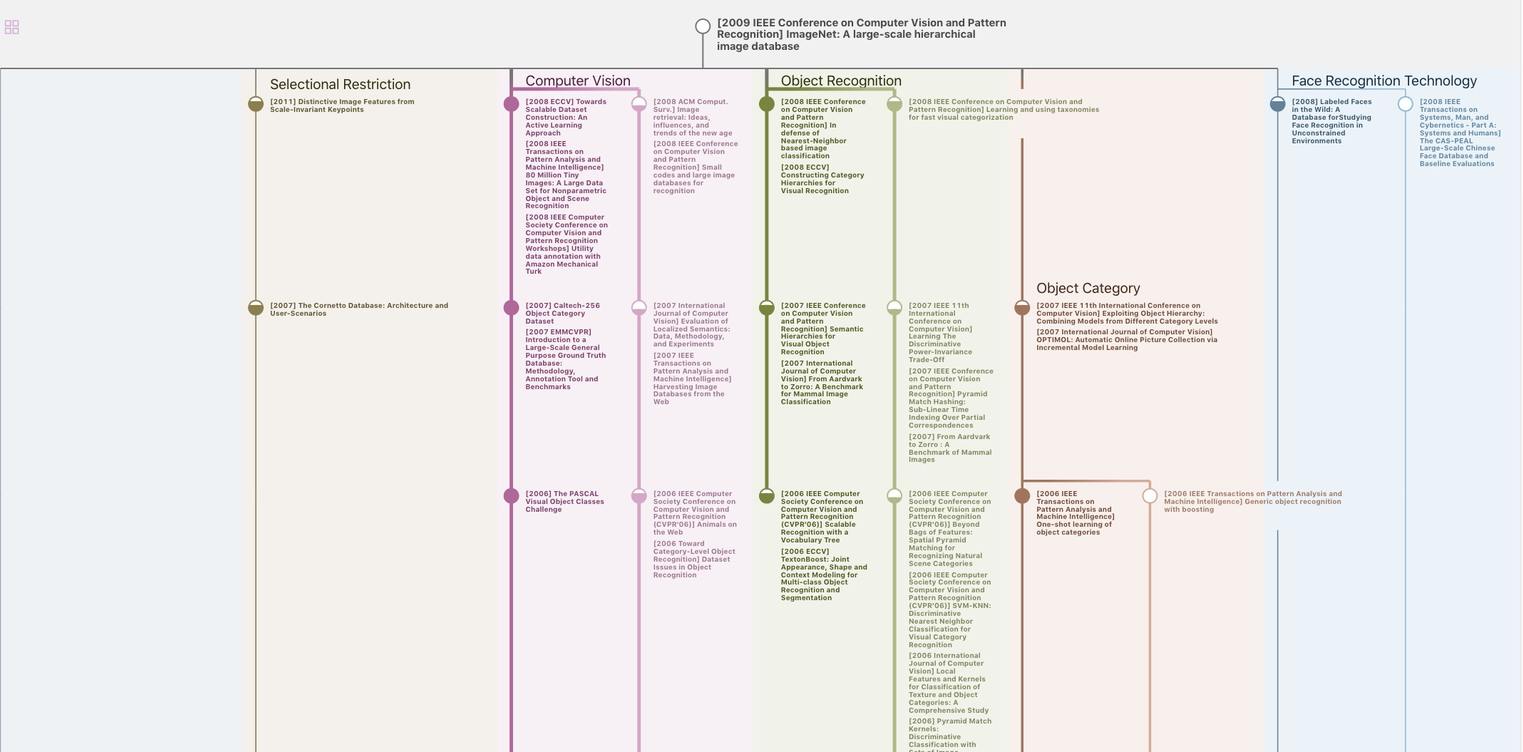
生成溯源树,研究论文发展脉络
Chat Paper
正在生成论文摘要