Probabilistic Knowledge Distillation of Face Ensembles
CVPR 2023(2023)
摘要
Mean ensemble (i.e. averaging predictions from multiple models) is a commonly-used technique in machine learning that improves the performance of each individual model. We formalize it as feature alignment for ensemble in open-set face recognition and generalize it into Bayesian Ensemble Averaging (BEA) through the lens of probabilistic modeling. This generalization brings up two practical benefits that existing methods could not provide: (1) the uncertainty of a face image can be evaluated and further decomposed into aleatoric uncertainty and epistemic uncertainty, the latter of which can be used as a measure for out-of-distribution detection of faceness; (2) a BEA statistic provably reflects the aleatoric uncertainty of a face image, acting as a measure for face image quality to improve recognition performance. To inherit the uncertainty estimation capability from BEA without the loss of inference efficiency, we propose BEA-KD, a student model to distill knowledge from BEA. BEA-KD mimics the overall behavior of ensemble members and consistently outperforms SOTA knowledge distillation methods on various challenging benchmarks.
更多查看译文
AI 理解论文
溯源树
样例
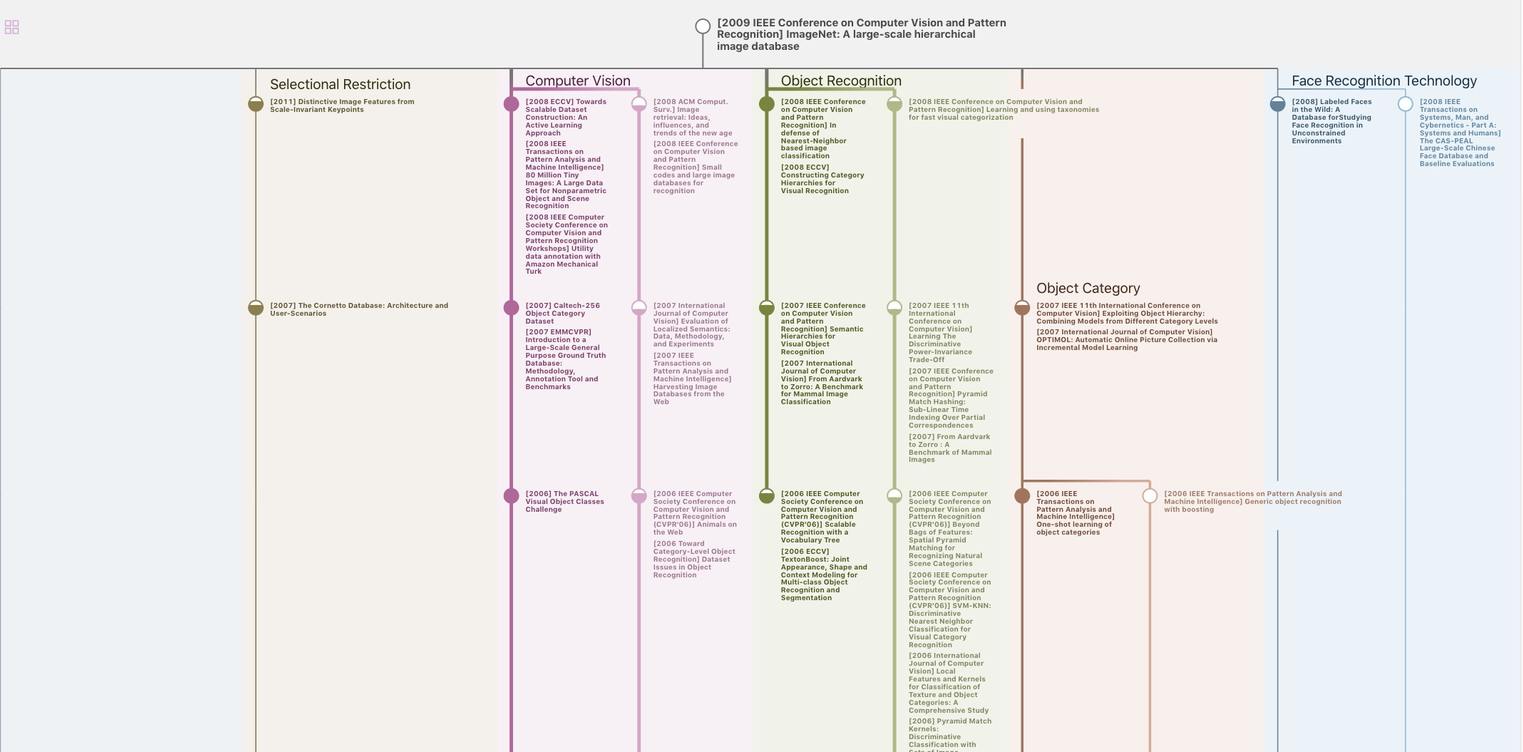
生成溯源树,研究论文发展脉络
Chat Paper
正在生成论文摘要