An ultra-lightweight efficient network for image-based plant disease and pest infection detection
Precision Agriculture(2023)
摘要
Plant diseases and pest infections are major factors that undermine the growth of plants along with their life cycle. Optical image-based plant disease detection provides an efficient and low cost way for real-time plant growth monitoring and management. In recent years, the thriving development of deep learning techniques in a variety of communities has validated its great performance in image interpretation and understanding. Existing deep learning-based methods for plant disease classification mostly adopt convolutional neural networks (CNNs) that have been originally developed for general image classification purposes. These CNN architectures consist of a very large volume of training parameters, which severely hinders its applicability under scenarios requiring fast and flexible deployment on compact devices with limited computation powers. In this paper, an ultra-lightweight efficient network (ULEN) is proposed targeting image-based plant disease and pest infection detection. The proposed network consists of two parts, a deep feature extraction module that adopts residual depth-wise convolution and a classification module receiving multi-scale features enhanced by a spatial pyramid pooling layer. The network is constructed in a very compact design with approximately only 100 000 parameters, which greatly favors the demand for a lightweight model for practical needs. Two publicly available plant datasets collected at the indoor and outdoor environments were tested on two compact devices to validate its applicability under different scenarios. Compared with the state-of-the-art architectures, the proposed network showed superior performance with the least computation complexity and compelling classification accuracy.
更多查看译文
关键词
Plant disease detection,Pest infection detection,Convolutional neural network,Deep learning
AI 理解论文
溯源树
样例
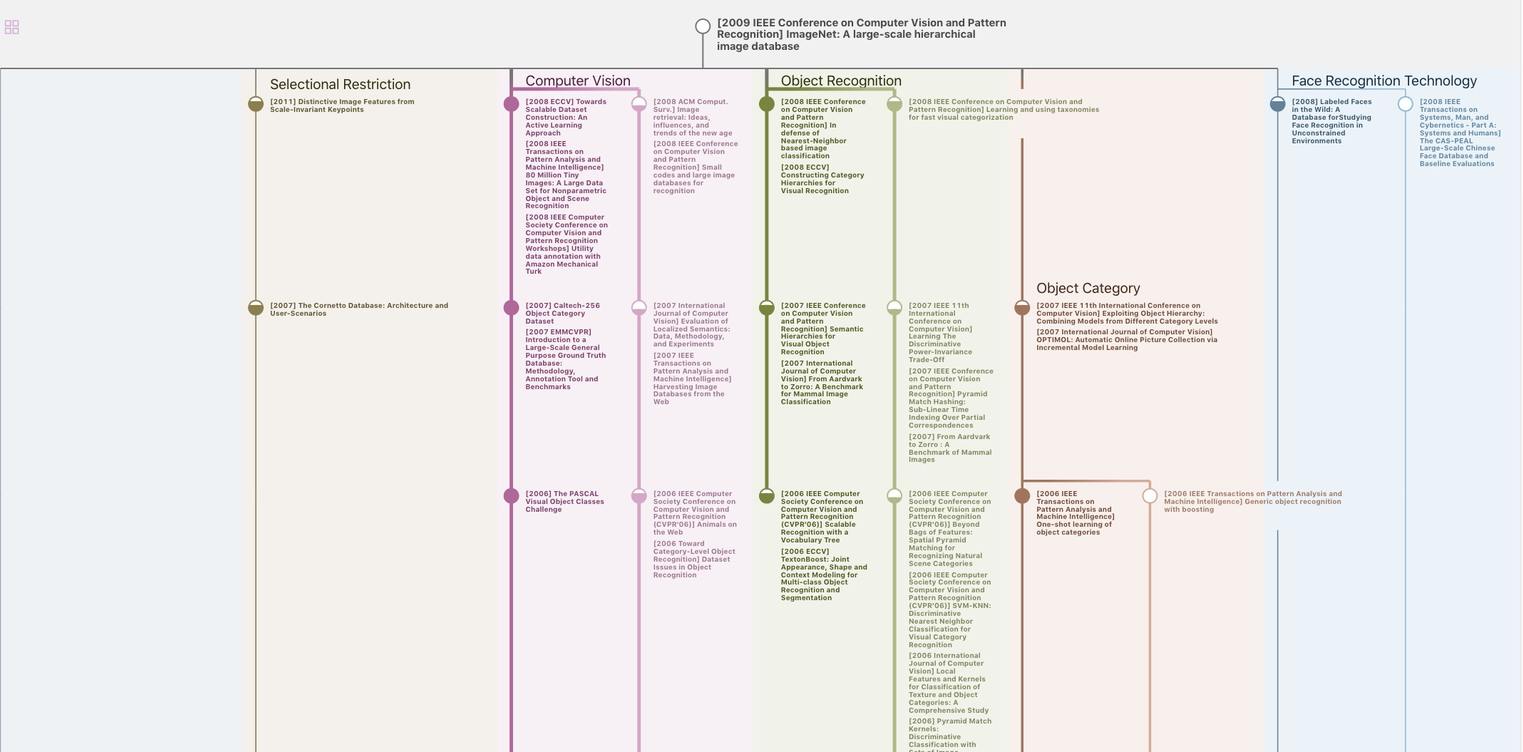
生成溯源树,研究论文发展脉络
Chat Paper
正在生成论文摘要