Stealthy Low-frequency Backdoor Attack against Deep Neural Networks
CoRR(2023)
摘要
Deep neural networks (DNNs) have gain its popularity in various scenarios in recent years. However, its excellent ability of fitting complex functions also makes it vulnerable to backdoor attacks. Specifically, a backdoor can remain hidden indefinitely until activated by a sample with a specific trigger, which is hugely concealed. Nevertheless, existing backdoor attacks operate backdoors in spatial domain, i.e., the poisoned images are generated by adding additional perturbations to the original images, which are easy to detect. To bring the potential of backdoor attacks into full play, we propose low-pass attack, a novel attack scheme that utilizes low-pass filter to inject backdoor in frequency domain. Unlike traditional poisoned image generation methods, our approach reduces high-frequency components and preserve original images' semantic information instead of adding additional perturbations, improving the capability of evading current defenses. Besides, we introduce "precision mode" to make our backdoor triggered at a specified level of filtering, which further improves stealthiness. We evaluate our low-pass attack on four datasets and demonstrate that even under pollution rate of 0.01, we can perform stealthy attack without trading off attack performance. Besides, our backdoor attack can successfully bypass state-of-the-art defending mechanisms. We also compare our attack with existing backdoor attacks and show that our poisoned images are nearly invisible and retain higher image quality.
更多查看译文
关键词
low-frequency
AI 理解论文
溯源树
样例
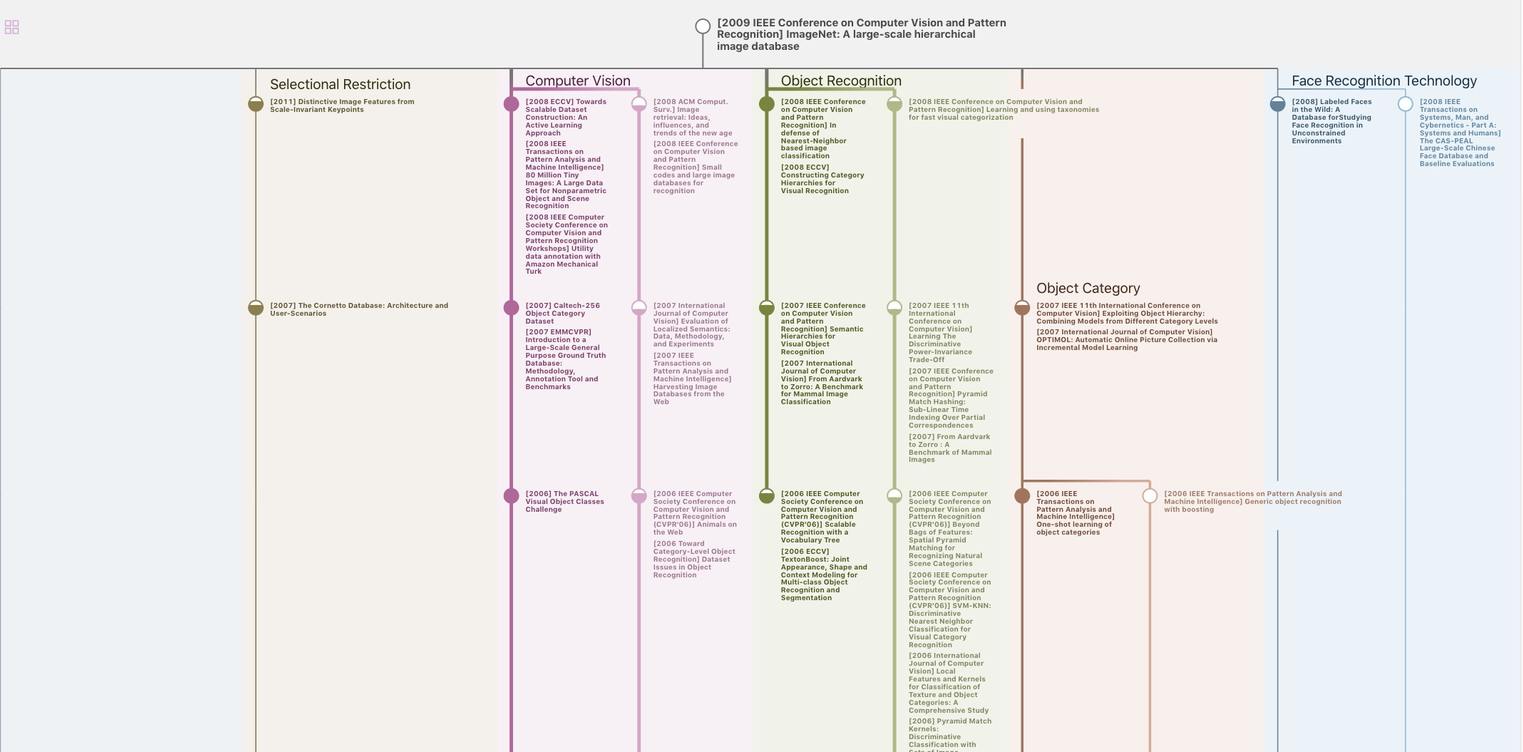
生成溯源树,研究论文发展脉络
Chat Paper
正在生成论文摘要