Reinforcement Learning for Safe Robot Control using Control Lyapunov Barrier Functions
CoRR(2023)
摘要
Reinforcement learning (RL) exhibits impressive performance when managing complicated control tasks for robots. However, its wide application to physical robots is limited by the absence of strong safety guarantees. To overcome this challenge, this paper explores the control Lyapunov barrier function (CLBF) to analyze the safety and reachability solely based on data without explicitly employing a dynamic model. We also proposed the Lyapunov barrier actor-critic (LBAC), a model-free RL algorithm, to search for a controller that satisfies the data-based approximation of the safety and reachability conditions. The proposed approach is demonstrated through simulation and real-world robot control experiments, i.e., a 2D quadrotor navigation task. The experimental findings reveal this approach's effectiveness in reachability and safety, surpassing other model-free RL methods.
更多查看译文
关键词
safe robot control,control lyapunov
AI 理解论文
溯源树
样例
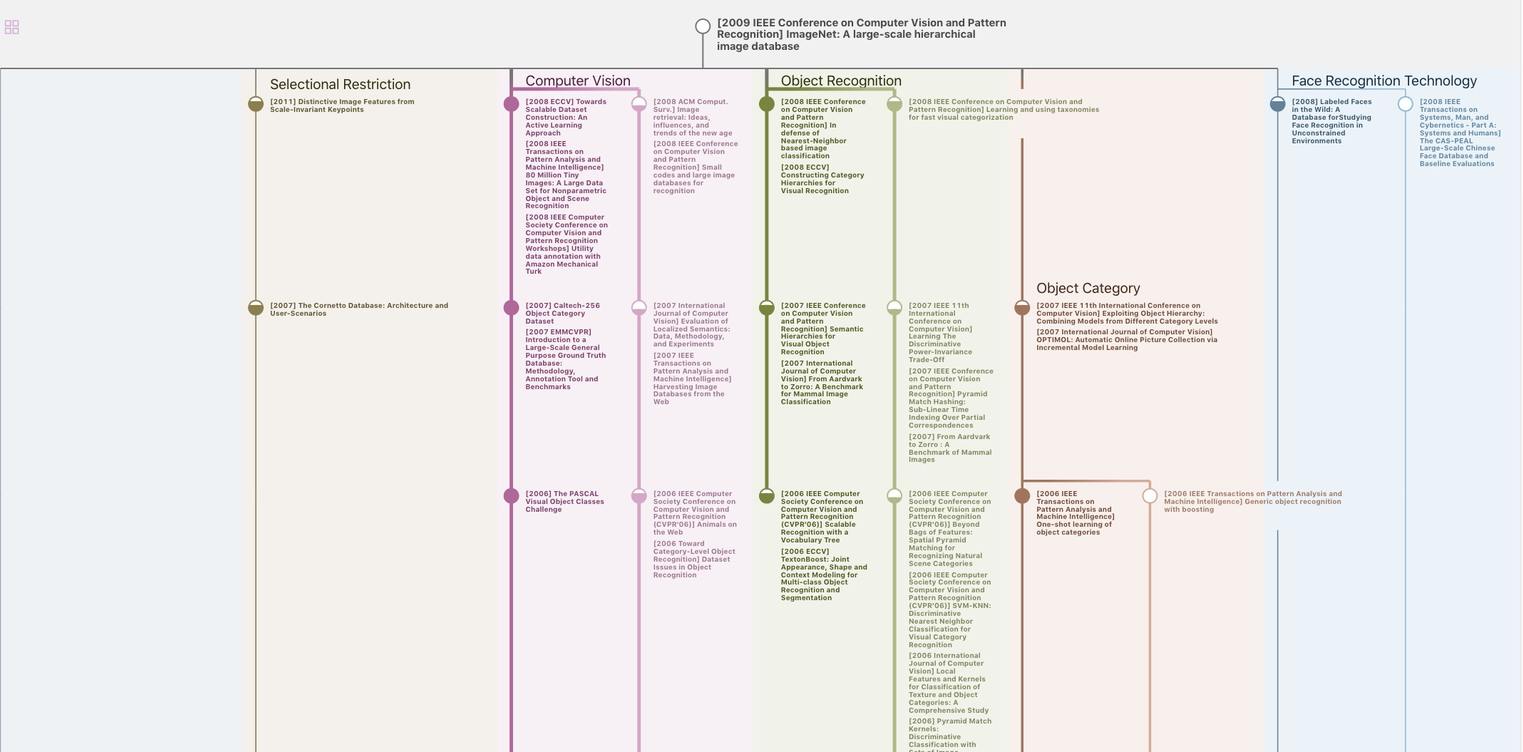
生成溯源树,研究论文发展脉络
Chat Paper
正在生成论文摘要