NAI_2: Learning Noise-Aware Illumination-Interpolator for Unsupervised Low-Light Image Enhancement
arxiv(2023)
摘要
Contemporary Low-Light Image Enhancement (LLIE) techniques have made notable
advancements in preserving image details and enhancing contrast, achieving
commendable results on specific datasets. Nevertheless, these approaches
encounter persistent challenges in efficiently mitigating dynamic noise and
accommodating diverse low-light scenarios. Insufficient constraints on complex
pixel-wise mapping learning lead to overfitting to specific types of noise and
artifacts associated with low-light conditions, reducing effectiveness in
variable lighting scenarios. To this end, we first propose a method for
estimating the noise level in low light images in a quick and accurate way.
This facilitates precise denoising, prevents over-smoothing, and adapts to
dynamic noise patterns. Subsequently, we devise a Learnable Illumination
Interpolator (LII), which employs learnlable interpolation operations between
the input and unit vector to satisfy general constraints between illumination
and input. Finally, we introduce a self-regularization loss that incorporates
intrinsic image properties and essential visual attributes to guide the output
towards meeting human visual expectations. Comprehensive experiments validate
the competitiveness of our proposed algorithm in both qualitative and
quantitative assessments. Notably, our noise estimation method, with linear
time complexity and suitable for various denoisers, significantly improves both
denoising and enhancement performance. Benefiting from this, our approach
achieves a 0.675dB PSNR improvement on the LOL dataset and 0.818dB on the MIT
dataset on LLIE task, even compared to supervised methods.
更多查看译文
AI 理解论文
溯源树
样例
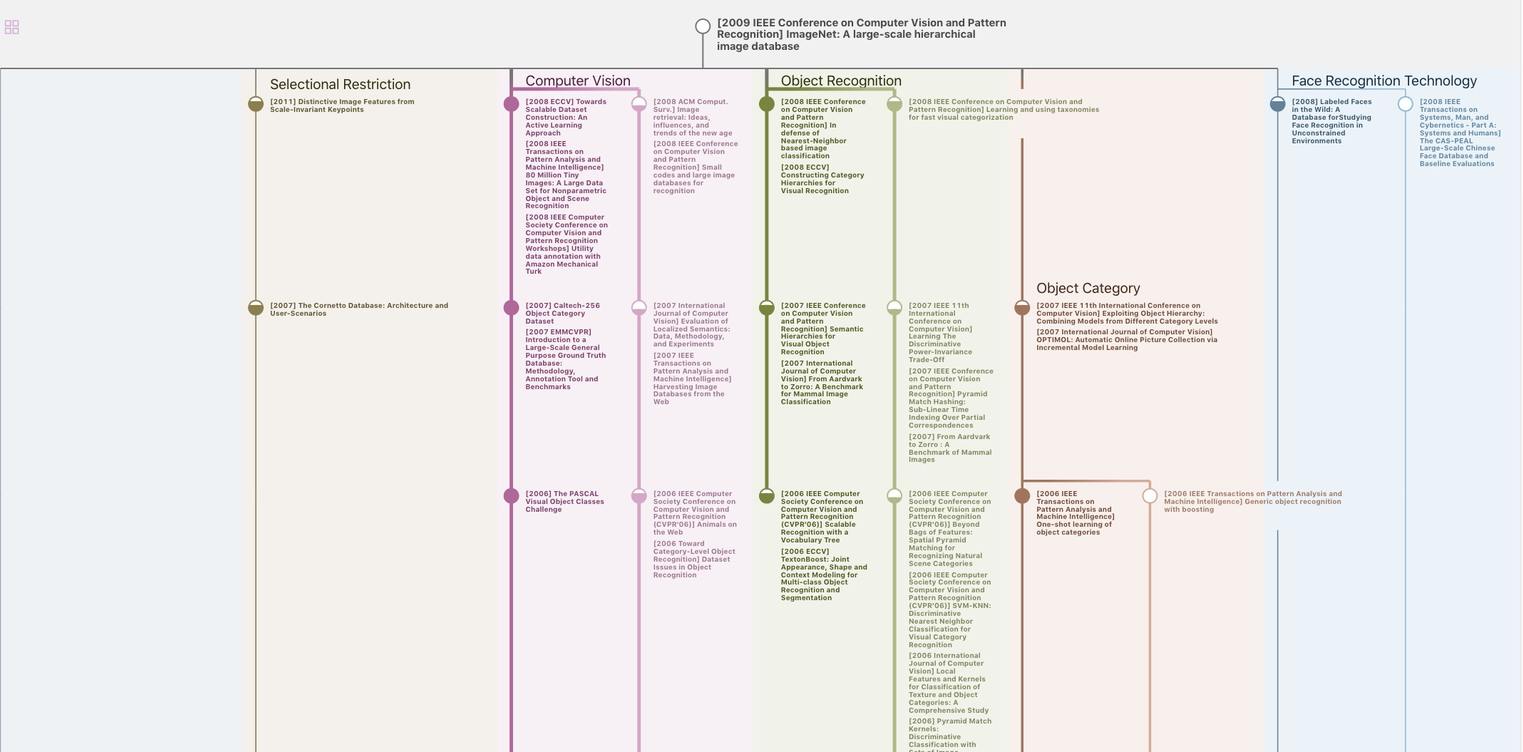
生成溯源树,研究论文发展脉络
Chat Paper
正在生成论文摘要