Landslide Detection with Ensemble-of-Deep Learning Classifiers Trained with Optimal Features
Advances in Data Science and Artificial Intelligence(2023)
摘要
Landslide Susceptibility Mapping (LSM) depends on aerial picture interpretation and field verification, but gathering aerial photos is difficult. Researchers have gradually applied LSM to environmental monitoring using remote sensing. In modest shallow landslides, laser scans cannot detect a landslide’s texture, color, and other features compared to the surrounding ground objects in optical photographs. WorldView, QuickBird, GaoFen-2, IKONOS, and InSAR technologies are increasingly employed to LSM. CNN model is often utilized in landslide research. In landslide detection, its upgraded approach residual neural networks (ResNets) has demonstrated promising results. An ensemble of deep learning classifiers is proposed. In this research work, a novel landslide detection model for GIS images will be introduced by following their major phases: (i) pre-processing, (ii) feature extraction (iii) feature selection, and (iv) classification. The captured images will be pre-processed via Gabor filtering. Subsequently, the features like GLCM based texture features, temperature-vegetative index-based characteristics, Brightness Index (BR), Normalized Difference Vegetation Index (NDVI) and Green Normalized Difference Vegetation Index (GNDVI), Red-over-Green difference (RGD), Vegetation index difference (VID), Brightness difference (BRD), NDI based features and coloration index features are extracted from the pre-processed data. The ensemble-of-classifiers model is constructed with Recurrent Neural Network (RNN), Bi-LSTM, and Bi-GRU. All these classifiers are trained with the selected optimal features acquired with the new hybrid optimization model. The ultimate outcome regarding the landslide forecasting has been acquired by fusing the outcomes acquired from RNN, Bi-LSTM and Bi-GRU. The obtained results outperform the existing state-of-the-art models and achieve an accuracy of 87% over Bijie landslide datasets. The dataset splitted into 70% training data and 30% testing dataset.
更多查看译文
关键词
detection,features,ensemble-of-deep
AI 理解论文
溯源树
样例
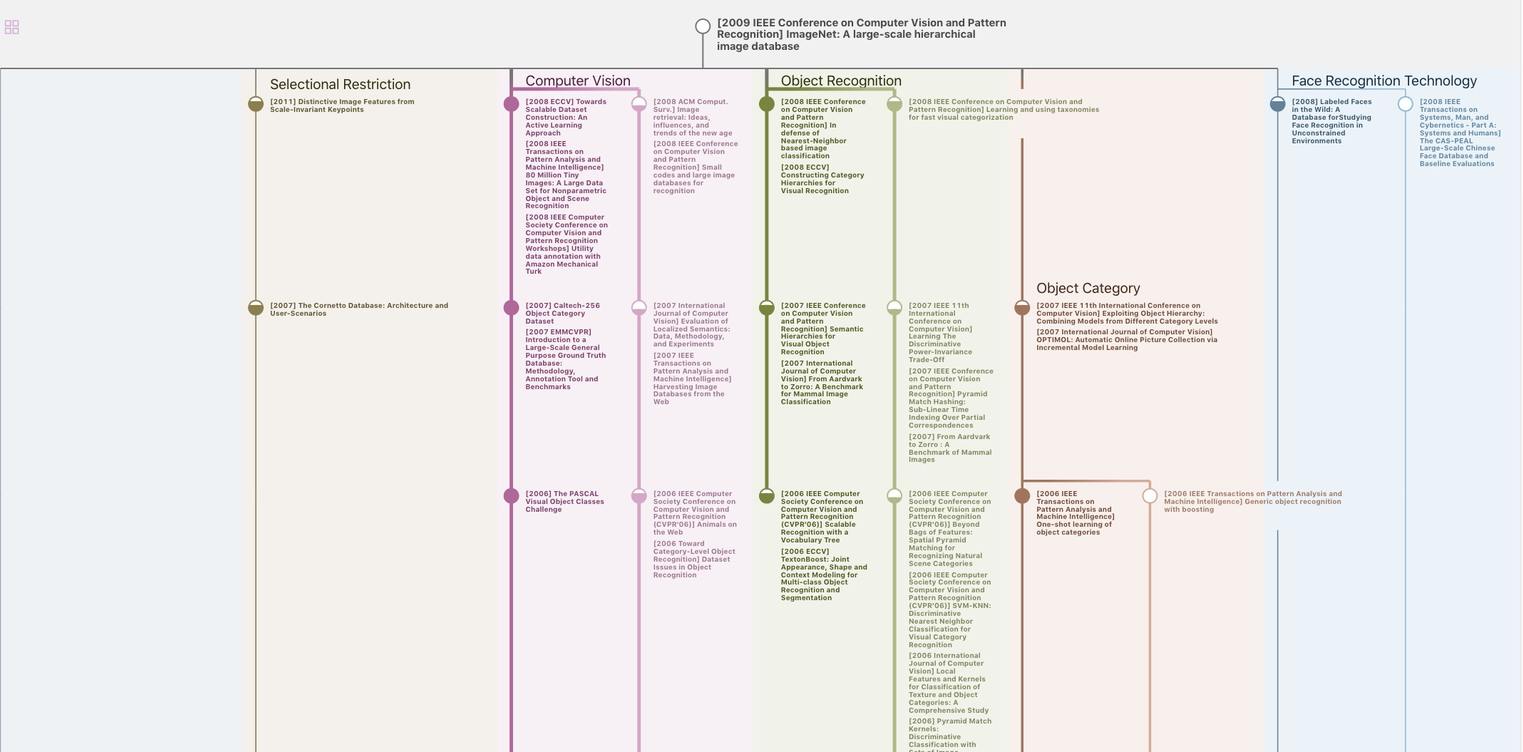
生成溯源树,研究论文发展脉络
Chat Paper
正在生成论文摘要