Powering Fine-Tuning: Learning Compatible and Class-Sensitive Representations for Domain Adaption Few-shot Relation Extraction
Database Systems for Advanced Applications(2023)
摘要
Relation extraction (RE) is an important task in information extraction that has drawn much attention. Although many RE models have achieved impressive performance, their performance drops dramatically when adapting to the new domain and under few-shot scenarios. One reason is that the huge gap in semantic space between different domains makes the model obtain suboptimal representations in the new domain. The other is the inability to learn class-sensitive information with only a few samples, which makes the instances with confusing factors hard to be distinguished. To address these issues, we propose a Contrastive learning-based Fine-Tuning approach with Knowledge Enhancement (CFTKE) for the Domain Adaptation Few-Shot RE task (DAFSRE). Specifically, we fine-tune the model in a contrastive-learning way to refine the semantic space in the new domain, which can bridge the gap between different domains and obtain better representations. To enhance the stability and learning ability of contrastive learning-based fine-tuning, we design the data augmentation mechanism and type-aware networks to enrich the instances and stand out the class-sensitive features. Extensive experiments on the DAFSRE benchmark dataset demonstrate that our approach significantly outperforms the state-of-the-art models (by 2.73% on average).
更多查看译文
关键词
relation extraction,representations,fine-tuning,class-sensitive,few-shot
AI 理解论文
溯源树
样例
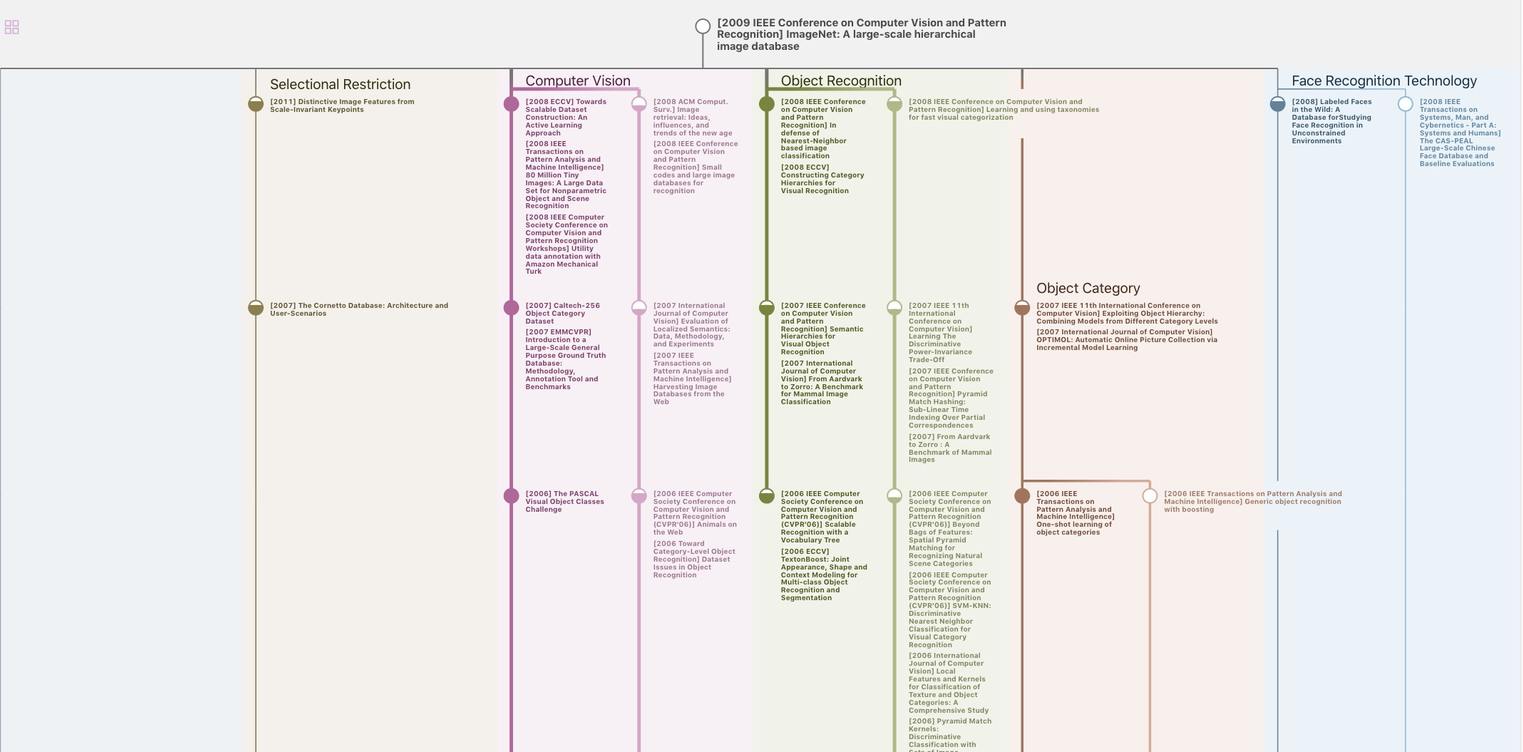
生成溯源树,研究论文发展脉络
Chat Paper
正在生成论文摘要