Investigation of Predictive Maintenance Algorithms for Rotating Shafts Under Various Bending Loads
Smart, Sustainable Manufacturing in an Ever-Changing World(2023)
摘要
Condition monitoring plays an important role with regard to forecasting structural failure of shafts in Advanced Manufacturing Systems (AMS). Repairs affect the downtime of machines considerably due to scheduled maintenance. The waste product of scheduled maintenance are parts that are treated as exhausted components for disposal. The disposed parts contain Residual Useful Life (RUL). Operational costs due to scheduled maintenance can be reduced through Condition Monitoring (CM) parameters that are utilized in Predictive Maintenance (PdM). The study utilizes logistic regression Machine Learning (ML) algorithm to predicting specific classification markers as a relevant step to monitor the health of a bright-steel shaft under various bending loading. Various loading conditions were monitored and compared, employing Principal Component Analysis (PCA). Predictions were tested by utilizing K-Means and DBSCAN clustering techniques. The Logistic Regression (LR) machine learning algorithm was employed to determine the prediction accuracy under various loads. A shaft was rotated under various bending loads which followed the experimental methodology of the R.R. Moore fatigue test. The goal of the experiment was to determine the prediction accuracy under various loads. Prediction scores for K-means clustering showed a overall decrease in accuracy in the increase of cluster numbers and the prediction accuracy showed increases and decreases for DBSCAN clustering with the increase of loading for various cluster selection.
更多查看译文
关键词
predictive maintenance algorithms,shafts,various bending loads
AI 理解论文
溯源树
样例
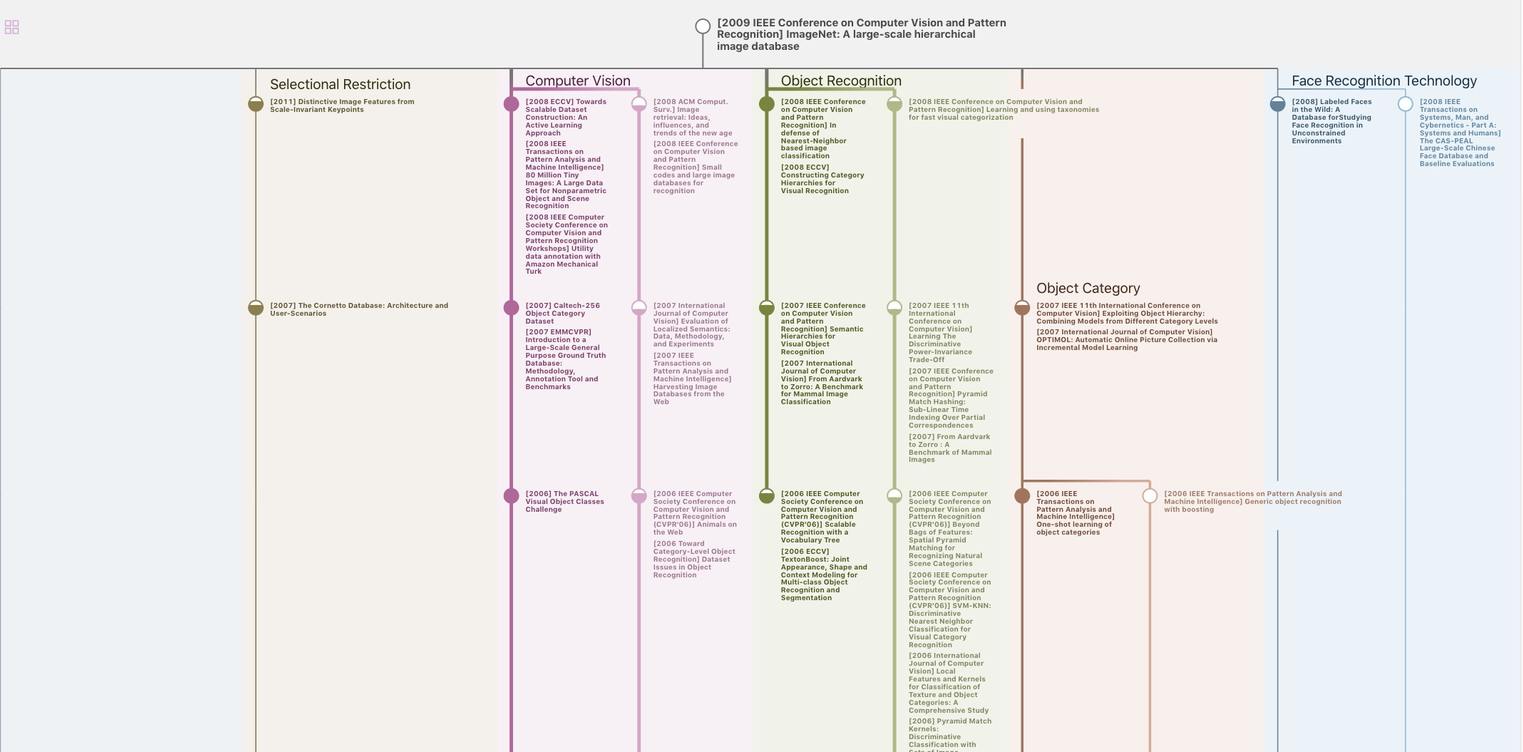
生成溯源树,研究论文发展脉络
Chat Paper
正在生成论文摘要