Intrinsic Use of Genetic Optimizer in CNN Towards Efficient Image Classification
Soft Computing and Its Engineering Applications(2023)
摘要
The inception of genetic algorithms in the 80’s laid a strong foundation in the theory of optimization. Numerous engineering applications are rewarded with wings of optimal and faster solutions through suitable genetic modelling. So far, a handful of evolutionary algorithms and modelling have been introduced by researchers. This has led to vivid applications in numerous domains. In this paper a customized evolutionary framework is proposed that is blended with deep learning mechanism. The widely used convolutional neural networks (CNN) model has been customized whereby optimally informative features are selected through intermittent genetic optimization. The inherent convolution layer outcomes are subjected to the optimizer module that in turn results in optimized set of feature points. The pooling process is abandoned for the purpose; thus, getting rid of uniform feature selection. Now, with this model the feature selection inhibits dynamic process of optimized feature selection. Case study on the usage of the same is shown on classification of facial expression images. Performance of the proposed mechanism is further compared with the simulated outcomes of the generic CNN model. Nevertheless, to say the results show promising rate of efficiency.
更多查看译文
关键词
CNN,Genetic optimization,Soft computing,Deep learning,Fitness function
AI 理解论文
溯源树
样例
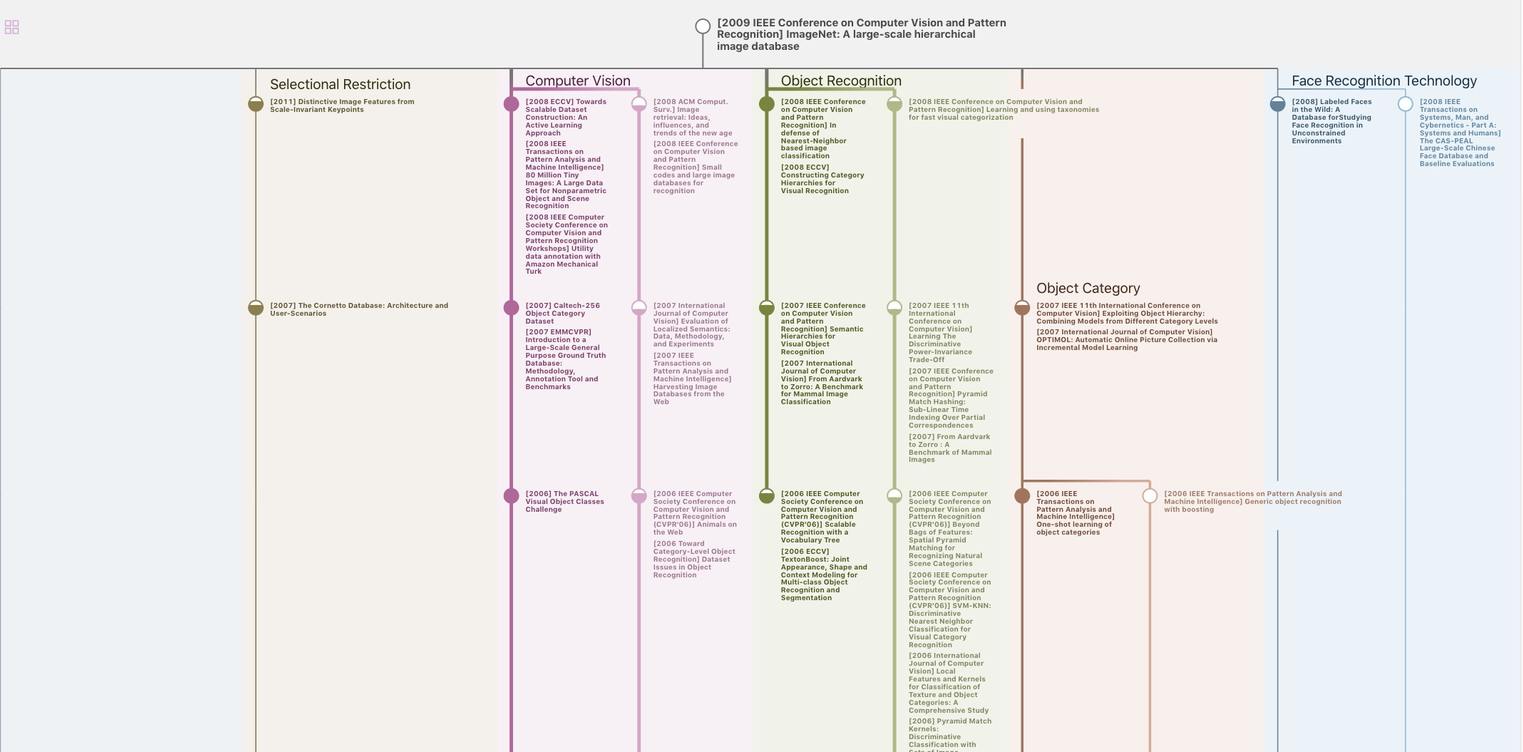
生成溯源树,研究论文发展脉络
Chat Paper
正在生成论文摘要