Detecting Fake Reviews Using Multiple Machine Learning Models: A Comparative Study
Computer Vision and Robotics(2023)
摘要
With the manifold growth of the e-commerce ecosystem in the last decade, online reviews are increasingly being viewed as a crucial factor in establishing and maintaining a positive reputation between customers and vendors. Further, reviews play an important and unbiased role in the decision making process for customers and a positive review for a targeted item usually draws a large number of customers and leads to significant rise in sales. These days, fake reviews are published on purpose to boost the company's virtual reputation and attract new customers. Due to which, detection of fake reviews is an active and emerging research topic. The purpose of this paper is to compare different machine learning techniques to detect fake reviews. For that purpose, a comparative study has been done among four well-known machine learning approaches namely Naive Bayes (NB), Support Vector Machine (SVM), Multi-layer Perceptron (MLP) and Long Short-term Memory (LSTM) on the Fake Reviews Dataset from Salminen. Among these, the Long Short-term Memory model provides the highest accuracy of 92% followed by Multi-layer Perceptron and Support Vector Machine.
更多查看译文
关键词
Fake reviews, Dataset, Amazon, Naïve Bayes (NB), Support Vector Machine (SVM), Multi-layer Perceptron (MLP), Long Short-term Memory (LSTM), Feature engineering
AI 理解论文
溯源树
样例
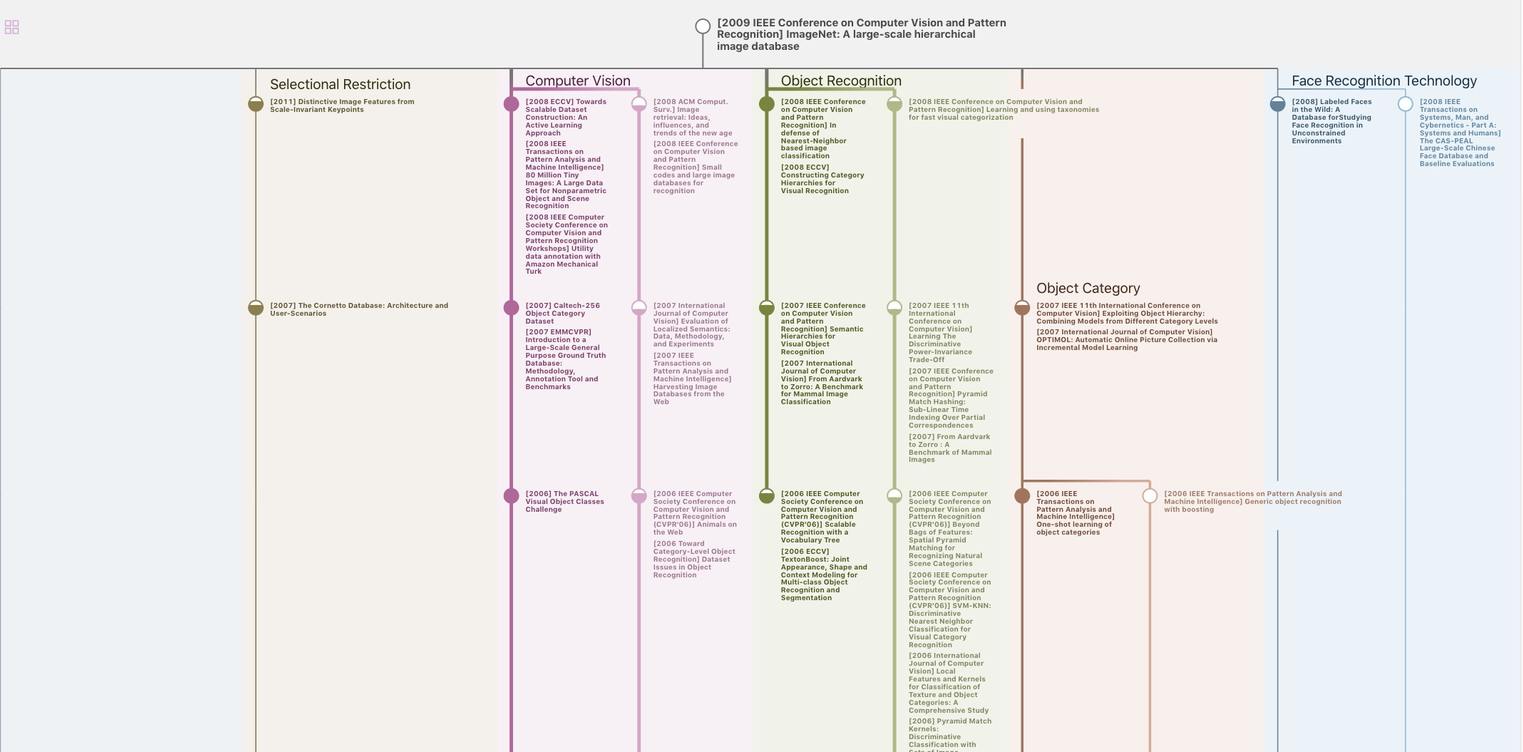
生成溯源树,研究论文发展脉络
Chat Paper
正在生成论文摘要