Introducing Spatial Context in Patch-Based Deep Learning for Semantic Segmentation in Whole Body MRI
Image Analysis(2023)
摘要
Improved imaging protocols and rapid scanning are making whole-body imaging increasingly popular in medical applications, where accurate object segmentation often plays an important role. Nowadays, convolutional neural networks (CNN) are the go-to method for automated image segmentation. Unfortunately, with improved image resolution, whole-body scans can grow large in size, preventing CNN training on entire images due to GPU memory limitations. An alternative to full-image training is patch-based training, which however suffers from context loss. We propose a way of reintroducing spatial context with marginal increase in memory requirements by employing landmark-normalized distance maps that carry relative position information for the patches. As a proof-of-concept we evaluate its effect with multiple networks with and without built-in context integration, using a multi-class whole-body MRI dataset. The results show that our proposed approach yields significant improvement over the baseline networks, even when used out-of-the box. We provide a discussion on its regularization properties and usability cases.
更多查看译文
关键词
semantic segmentation,deep learning,spatial context,mri,patch-based
AI 理解论文
溯源树
样例
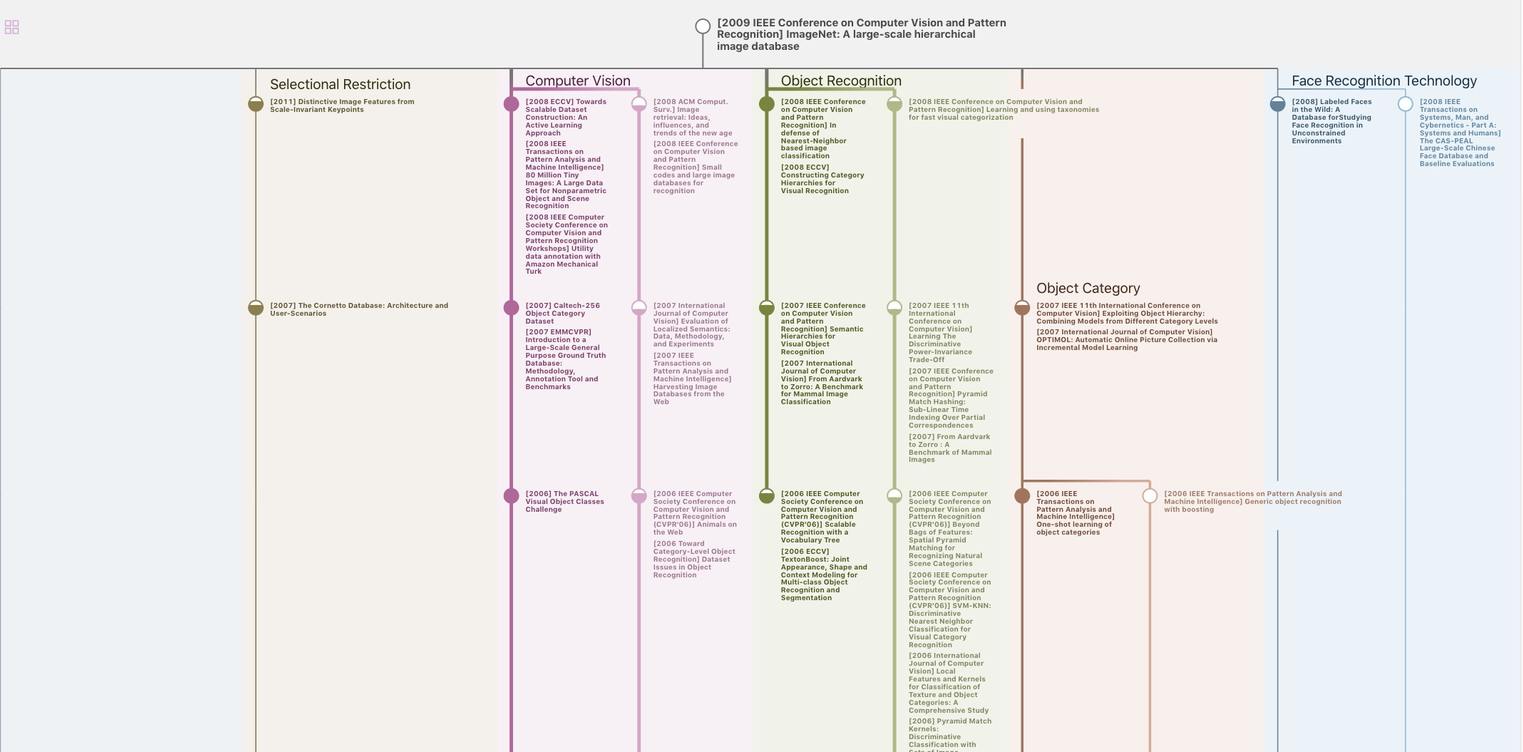
生成溯源树,研究论文发展脉络
Chat Paper
正在生成论文摘要