Data-Driven Approach for Defining Demand Scenarios for Shared Autonomous Cargo-Bike Fleets
Smart Energy for Smart Transport(2023)
摘要
Bike sharing systems have become a sustainable alternative to motorized private transport in urban areas. However, users often face high costs and availability issues due to the operational effort required to redistribute bicycles between stations. For addressing those issues, the AuRa (Autonomes Rad, Eng. Autonomous Bicycle) project introduces a new mobility offer in terms of an on-demand, shared-use, self-driving cargo bikes service (OSABS) that enables automated redistribution. Within the project, we develop different order management and rebalancing strategies and validate them using simulation models. One prerequisite for this is sound demand scenarios. However, due to the novelty of OSABS, there is currently no information about its utilization. Consequently, the objective of this study was to develop an approach for defining OSABS demand scenarios in a temporally and spatially disaggregated manner as an input for simulation models. Therefore, we first derived city-wide usage potentials of OSABS from a survey on mobility needs. We then spatially and temporally disaggregated the determined usage likelihood using travel demand matrices and usage patterns from a conventional bike-sharing system, respectively. Finally, we performed cluster analyses on the resulting annual demand to summarize sections of the yearly profile into representative units and thus reduce the simulation effort. As we applied this approach as a case study to the city of Magdeburg, Germany, we could show that our methodology enables the determination of reasonable OSABS demand scenarios from scratch. Furthermore, we were able to show that annual usage patterns of (conventional) bike sharing systems can be modeled by using demand data for only eight representative weeks.
更多查看译文
关键词
bike sharing,demand generation,mobility on demand,autonomous bike,future mobility,cargo bike
AI 理解论文
溯源树
样例
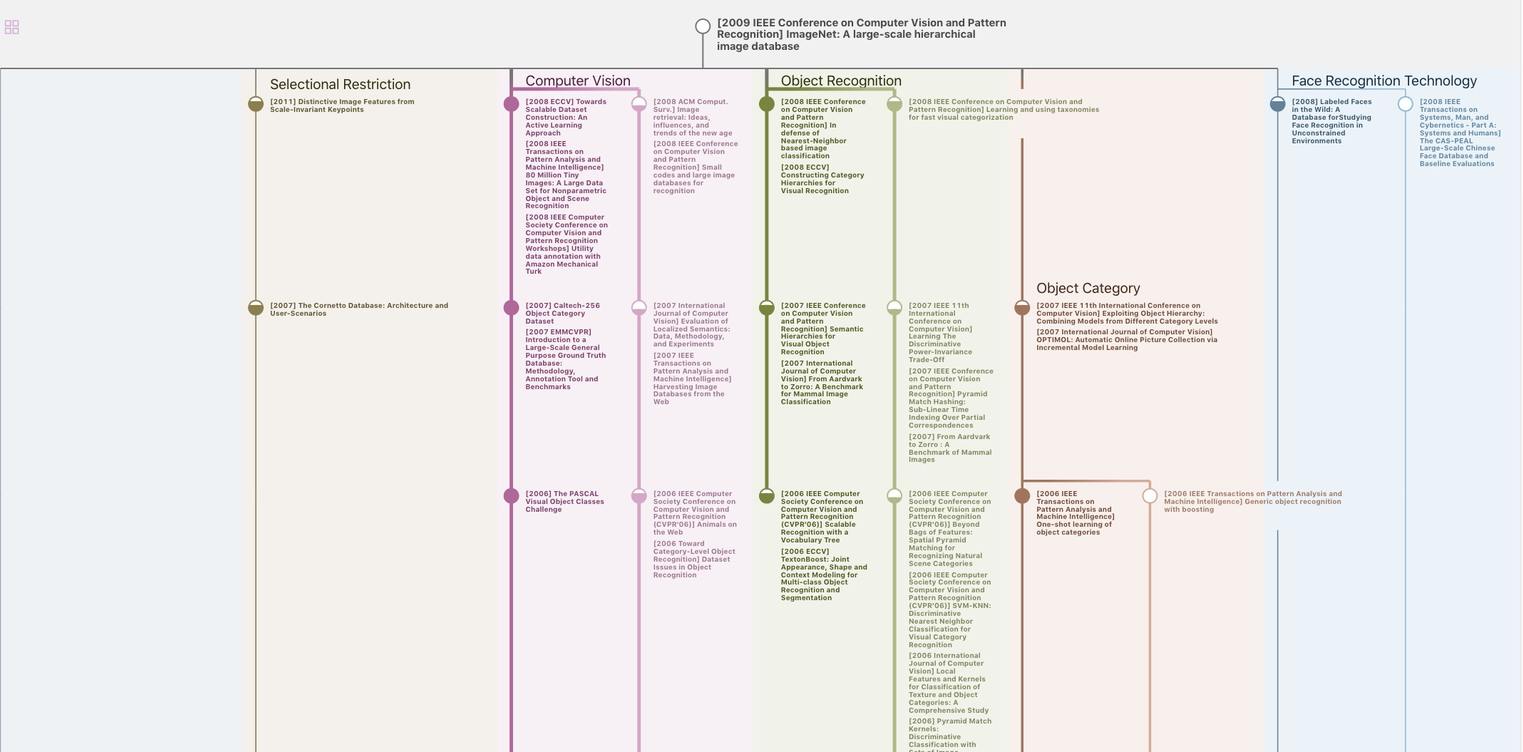
生成溯源树,研究论文发展脉络
Chat Paper
正在生成论文摘要