Prediction and Analysis of Polycystic Ovary Syndrome Using Machine Learning
Computational Intelligence for Engineering and Management Applications(2023)
摘要
Polycystic ovary syndrome (PCOS) is a widespread pathology that affects many aspects of women’s health, with long-term consequences beyond the reproductive age. The wide variety of clinical referrals, as well as the lack of internationally accepted diagnostic procedures, had a significant impact on making it difficult to determine the exact aetiology of the disease. The exact cause of PCOS is not yet clear. Therefore, multiple features are responsible for it. The aim of this project is to analyze simple factors (height, weight, lifestyle changes, etc.) and complex (imbalances of bio hormones and chemicals such as insulin and vitamin D) factors that contribute to the development of the disease. The data we used for our project was published in Kaggle, written by Prasoon Kottarathil, called polycystic ovary syndrome (PCOS) in 2020. This database contains records of 543 PCOS patients tested on the basis of 40 parameters. For this, we have used machine learning techniques such as logistic regression, decision trees, SVMs, and random forests. A detailed analysis of all the items made using graphs and programmes and prediction using machine learning models helped us to identify the most important indicators for the same.
更多查看译文
关键词
Random forest, Machine learning, PCOS, Chi-square test, Feature selection, Information gain
AI 理解论文
溯源树
样例
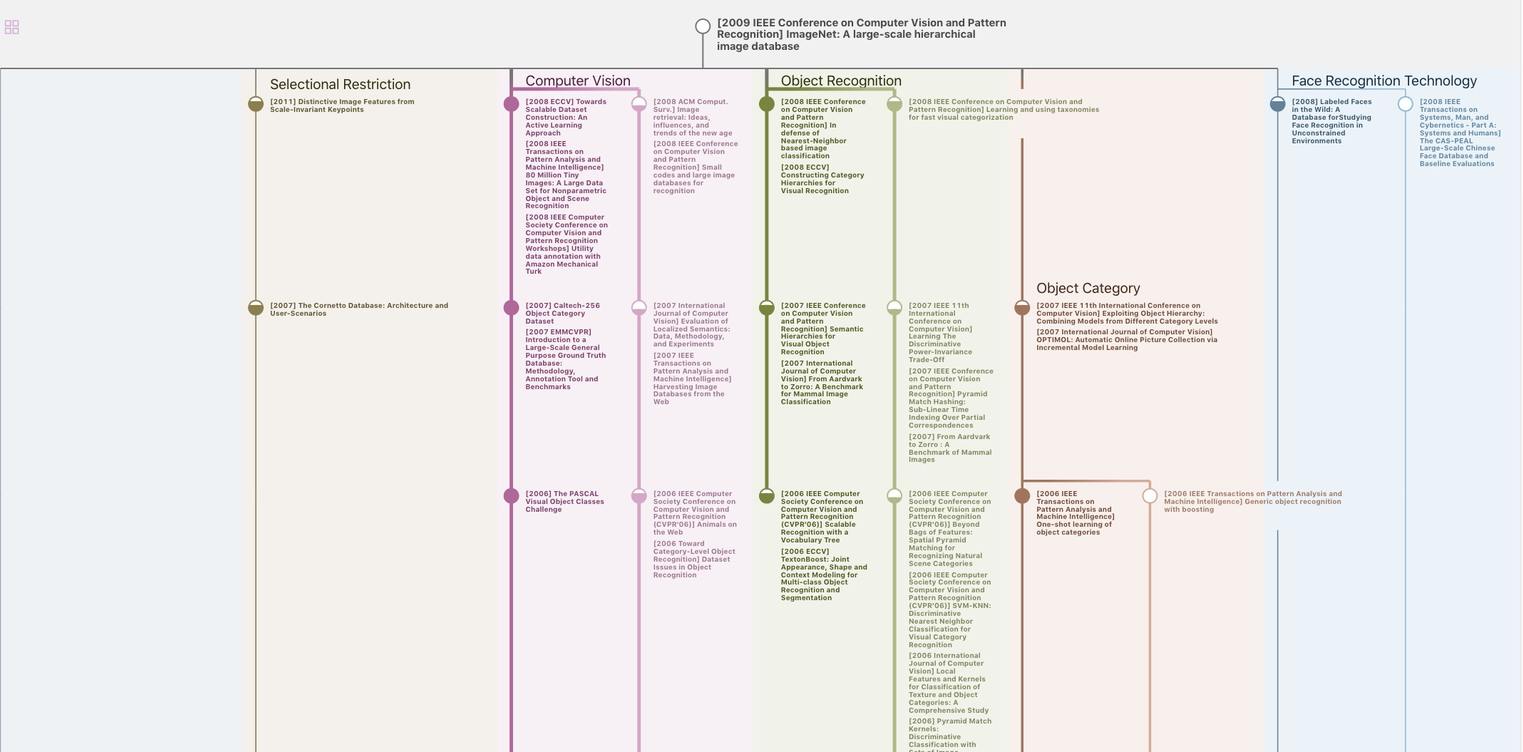
生成溯源树,研究论文发展脉络
Chat Paper
正在生成论文摘要