Music Generation and Composition Using Machine Learning
Proceedings of 3rd International Conference on Artificial Intelligence: Advances and Applications(2023)
摘要
In recent years, the complexity of music production has significantly reduced substantially, resulting in a large number of individuals creating music and uploading it to online streaming. Individuals are spending a lot of time looking for certain songs because to the massive music streaming media. As a result, in recent times, the ability to quickly classify music genres is critical. As machine learning and deep learning algorithms have been applied to a wide range of sectors, a number of DNN-based models have evolved. To manually extract characteristics from time series data, conventional music genre categorization needs necessary professional skills. In time series data, deep learning has been shown to be efficient and effective. In this work, the benefits and features of LSTM in audio is used to construct a music genre categorization model in order to reduce the user's time while looking for various types of music. In the feature extraction stage, some audio related features are extracted such as MFCC, mean harmony ratio, mean STFT, mean spectral centroid, etc. Then feature correlation was adopted to extract relevant features and fed into the LSTM model for training. The testing was evaluated on two datasets, classic piano dataset and GTZAN dataset. The average accuracy achieved on the classic piano dataset and GTZAN dataset is 85% and 96% respectively.
更多查看译文
关键词
Music generation, Machine learning, Deep learning, LSTM, Genre classification
AI 理解论文
溯源树
样例
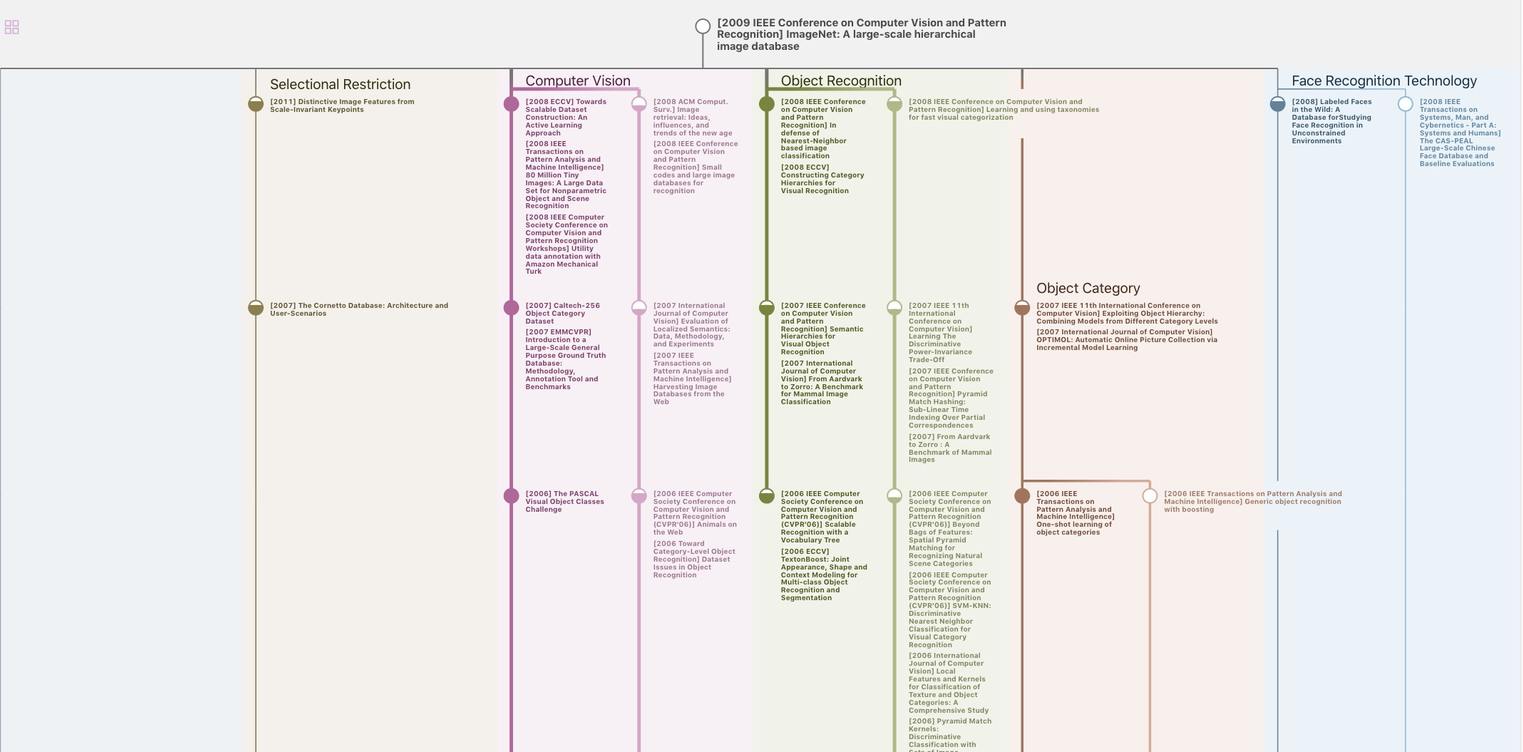
生成溯源树,研究论文发展脉络
Chat Paper
正在生成论文摘要